决策树python代码
时间: 2023-05-20 07:04:38 浏览: 76
以下是一个简单的决策树 Python 代码示例:
```python
from sklearn import tree
# 训练数据
features = [[140, 1], [130, 1], [150, 0], [170, 0]]
labels = [0, 0, 1, 1]
# 创建决策树模型
clf = tree.DecisionTreeClassifier()
# 训练模型
clf = clf.fit(features, labels)
# 预测新数据
print(clf.predict([[160, 0]]))
```
这个代码示例使用 scikit-learn 库中的决策树分类器来训练一个简单的模型,然后使用模型来预测新的数据。在这个示例中,我们使用两个特征来预测一个水果是苹果还是橘子:重量和表面是否光滑。
阅读全文
相关推荐
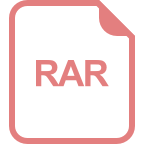
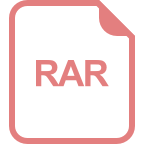
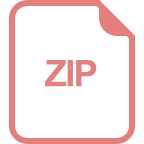
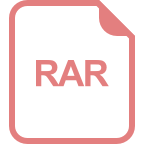
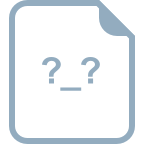
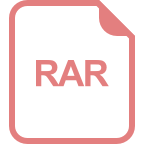
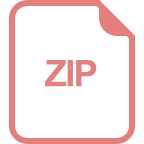
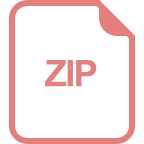
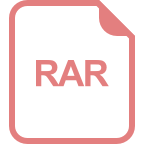
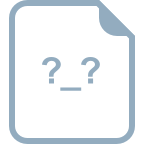