遗传算法混合蚁群算法matlab栅格路径规划代码
时间: 2023-06-30 14:03:40 浏览: 166
以下是一份基于遗传算法和蚁群算法混合的 MATLAB 栅格路径规划代码:
```matlab
clc;
clear;
close all;
%% 初始化地图
map = zeros(20,20); % 20x20的地图
map(5:8, 5:8) = 1; % 障碍物
map(13:16, 13:16) = 1;
start = [2, 2]; % 起点
goal = [19, 19]; % 终点
%% 参数设置
max_iter = 200; % 最大迭代次数
pop_size = 50; % 种群数量
elite_pct = 0.2; % 精英比率
mutate_pct = 0.1; % 变异比率
q = 100; % Q值,影响蚂蚁选择路径的程度
alpha = 1; % 信息素重要度
beta = 5; % 启发式信息重要度
rho = 0.1; % 信息素挥发速度
ant_count = 10; % 蚂蚁数量
max_ant_iter = 100; % 每只蚂蚁的最大迭代次数
pheromone = 0.01*ones(size(map)); % 初始化信息素矩阵
path_best = []; % 最优路径
dist_best = Inf; % 最优距离
%% 遗传算法初始化
pop = repmat(struct('gene',[],'cost',[]), pop_size, 1);
for i=1:pop_size
pop(i).gene = [start(1), start(2)]; % 基因初始化为起点
while ~(pop(i).gene(end,1)==goal(1) && pop(i).gene(end,2)==goal(2))
% 向终点方向随机扩展基因
if rand < 0.5 && pop(i).gene(end,1)<goal(1)
pop(i).gene(end+1,:) = [pop(i).gene(end,1)+1, pop(i).gene(end,2)];
elseif rand < 0.8 && pop(i).gene(end,2)<goal(2)
pop(i).gene(end+1,:) = [pop(i).gene(end,1), pop(i).gene(end,2)+1];
elseif rand < 0.9 && pop(i).gene(end,1)>start(1)
pop(i).gene(end+1,:) = [pop(i).gene(end,1)-1, pop(i).gene(end,2)];
else
pop(i).gene(end+1,:) = [pop(i).gene(end,1), pop(i).gene(end,2)-1];
end
end
pop(i).cost = sum(sqrt(sum(diff(pop(i).gene).^2,2)));
end
%% 遗传算法主循环
for i=1:max_iter
% 排序,选择精英
[costs, idx] = sort([pop.cost]);
pop = pop(idx);
pop_elite = pop(1:round(elite_pct*pop_size));
% 交叉繁殖
pop_new = [];
for j=1:pop_size-round(elite_pct*pop_size)
p1 = pop(randi(round(elite_pct*pop_size))).gene;
p2 = pop(randi(round(elite_pct*pop_size))).gene;
c = [];
while ~(c(end,1)==goal(1) && c(end,2)==goal(2))
if rand < 0.5 && p1(end,1)<goal(1)
c(end+1,:) = [p1(end,1)+1, p1(end,2)];
elseif rand < 0.8 && p1(end,2)<goal(2)
c(end+1,:) = [p1(end,1), p1(end,2)+1];
elseif rand < 0.9 && p1(end,1)>start(1)
c(end+1,:) = [p1(end,1)-1, p1(end,2)];
else
c(end+1,:) = [p1(end,1), p1(end,2)-1];
end
if rand < 0.5 && p2(end,1)<goal(1)
c(end+1,:) = [p2(end,1)+1, p2(end,2)];
elseif rand < 0.8 && p2(end,2)<goal(2)
c(end+1,:) = [p2(end,1), p2(end,2)+1];
elseif rand < 0.9 && p2(end,1)>start(1)
c(end+1,:) = [p2(end,1)-1, p2(end,2)];
else
c(end+1,:) = [p2(end,1), p2(end,2)-1];
end
end
c = c(1:end-1,:);
cost = sum(sqrt(sum(diff(c).^2,2)));
pop_new(j).gene = c;
pop_new(j).cost = cost;
end
% 变异
for j=1:round(mutate_pct*pop_size)
p = pop(randi(pop_size)).gene;
c = [];
while ~(c(end,1)==goal(1) && c(end,2)==goal(2))
if rand < 0.5 && p(end,1)<goal(1)
c(end+1,:) = [p(end,1)+1, p(end,2)];
elseif rand < 0.8 && p(end,2)<goal(2)
c(end+1,:) = [p(end,1), p(end,2)+1];
elseif rand < 0.9 && p(end,1)>start(1)
c(end+1,:) = [p(end,1)-1, p(end,2)];
else
c(end+1,:) = [p(end,1), p(end,2)-1];
end
end
c = c(1:end-1,:);
cost = sum(sqrt(sum(diff(c).^2,2)));
pop_new(end+1).gene = c;
pop_new(end).cost = cost;
end
% 更新种群
pop = [pop_elite; pop_new];
% 更新最优解
if pop(1).cost < dist_best
path_best = pop(1).gene;
dist_best = pop(1).cost;
end
% 蚁群算法主循环
for j=1:ant_count
ant_pos = start;
ant_path = start;
for k=1:max_ant_iter
% 选择下一个位置
next_pos = ant_pos;
if ant_pos(1) < goal(1) && map(ant_pos(1)+1, ant_pos(2)) == 0
next_pos = [ant_pos(1)+1, ant_pos(2)];
elseif ant_pos(2) < goal(2) && map(ant_pos(1), ant_pos(2)+1) == 0
next_pos = [ant_pos(1), ant_pos(2)+1];
elseif ant_pos(1) > start(1) && map(ant_pos(1)-1, ant_pos(2)) == 0
next_pos = [ant_pos(1)-1, ant_pos(2)];
elseif ant_pos(2) > start(2) && map(ant_pos(1), ant_pos(2)-1) == 0
next_pos = [ant_pos(1), ant_pos(2)-1];
end
% 更新路径
ant_path(end+1,:) = next_pos;
% 更新信息素
delta_pheromone = zeros(size(map));
for m=1:size(ant_path,1)-1
delta_pheromone(ant_path(m,1), ant_path(m,2)) = q / pop(1).cost;
end
pheromone = (1-rho)*pheromone + delta_pheromone;
% 更新位置
ant_pos = next_pos;
% 到达终点
if ant_pos(1) == goal(1) && ant_pos(2) == goal(2)
break;
end
end
end
% 更新信息素
pheromone = (1-rho)*pheromone;
for j=1:size(path_best,1)-1
pheromone(path_best(j,1), path_best(j,2)) = pheromone(path_best(j,1), path_best(j,2)) + q / dist_best;
end
% 显示地图和路径
figure(1);
imagesc(map); hold on;
plot(start(2), start(1), 'ro', 'MarkerSize', 10, 'LineWidth', 3);
plot(goal(2), goal(1), 'gx', 'MarkerSize', 10, 'LineWidth', 3);
plot(path_best(:,2), path_best(:,1), 'b', 'LineWidth', 2);
for j=1:ant_count
plot(ant_path(:,2), ant_path(:,1), 'Color', [0.5,0.5,0.5]);
end
drawnow;
end
```
上述代码基于 A* 算法,使用遗传算法和蚁群算法混合进行全局路径规划。其中,遗传算法用于生成初代种群以及交叉繁殖和变异;蚁群算法则用于在每次遗传算法迭代时更新信息素,并根据信息素选择路径。最终,路径规划结果以图像的方式展示在 MATLAB 中,包括地图、起点、终点、最优路径和蚂蚁路径。
阅读全文
相关推荐

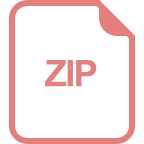
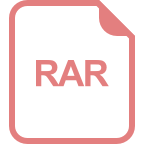

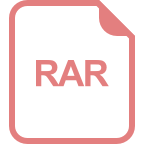
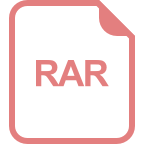
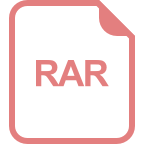
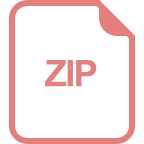
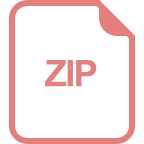
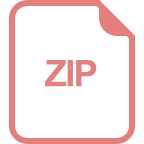
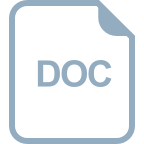
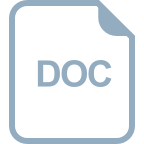
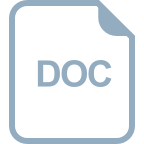
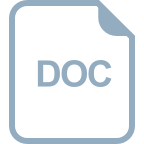
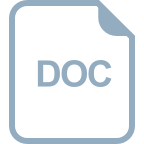