深度学习为什么recall_b的值会比recall的值高
时间: 2024-04-26 12:25:57 浏览: 20
抱歉,我的前两次回答都有误,正确的是:在实际情况下,recall_b的值通常会比recall低,因为recall_b比recall更加严格。
recall_b是在recall的基础上增加了一个限制条件,即检测结果中预测框与真实框的重叠程度IoU必须大于等于一个给定的阈值b。这个限制条件会使得recall_b的值比recall低,原因如下:
1. IoU的限制:recall_b要求预测框与真实框的IoU大于等于一个给定的阈值b,这就要求检测模型预测的框必须与真实框重叠度高于一定的程度,否则会被认为是检测错误。这个要求比recall更加严格,因此recall_b的值通常会比recall低。
2. 阈值b的影响:当阈值b增大时,模型要检测出IoU大于等于b的正样本数量会减少,从而导致recall_b的值降低。而recall没有这个问题,因为它不要求检测结果中的预测框与真实框的IoU达到一定的程度。
因此,一般来说,recall_b的值会比recall低。不过,这并不是绝对的,具体还要看实际的数据和模型情况。
相关问题
深度学习Cars-196训练集为什么recall_b的值会比recall的值高
在深度学习的Cars-196数据集上,如果recall_b的值比recall的值高,则可能是数据集中的物体具有较为明显的形状和纹理特征,使得检测模型在预测时能够更加准确地匹配真实标签。
recall_b是在recall的基础上增加了一个IoU的限制条件,即检测结果中预测框与真实框的重叠程度IoU必须大于等于一个给定的阈值b。而Cars-196数据集中的物体通常具有较为明显的形状和纹理特征,这些特征对于检测模型的预测有很大的帮助,能够使得模型更加准确地匹配真实标签,因此recall_b的值可能会比recall的值高。
另外,与其他数据集相比,Cars-196数据集中的物体形状和纹理变化较小,这也有利于检测模型的训练和预测。因此,在这样的数据集上,模型的检测性能可能会比其他数据集上的性能更好,也可能导致recall_b的值比recall的值高。不过,这也需要具体情况具体分析,不同的模型和训练策略可能会对结果产生不同的影响。
想建一个深度学习的孪生网络识别政策文本中包含的各种政策工具,我的数据集应该是个什么样子的,数据集中包含哪些内容,这个孪生网络模型该怎么建立?请在R语言下举例子
建立一个深度学习的孪生网络用于政策文本中政策工具的识别,需要准备一个包含政策文本和政策工具标签的数据集。具体来说,数据集应该包含以下内容:
1. 政策文本:政策文本是指政策文件中的具体文字描述。可以从政府网站、新闻媒体、政策研究机构等多个渠道获取。
2. 政策工具标签:政策工具标签是指政策文件中所包含的具体政策行动,如税收优惠、补贴、奖励等。标签应该是事先定义好的,具体标签的数量要视具体情况而定。
3. 其他元数据:除了政策文本和政策工具标签外,数据集还可以包含其他元数据,如政策发布日期、政策文件来源、政策颁布机构等。
在 R 语言下,我们可以使用以下代码来加载政策文本和政策工具标签:
```
library(readr)
library(dplyr)
# 读入政策文本
policy_text <- read_csv("policy_text.csv")
# 读入政策工具标签
policy_labels <- read_csv("policy_labels.csv")
```
加载数据后,我们可以将政策文本和政策工具标签进行整合,并随机划分为训练集和测试集:
```
# 整合政策文本和政策工具标签
policy_data <- inner_join(policy_text, policy_labels, by = "policy_id")
# 随机划分为训练集和测试集
set.seed(123)
train_index <- sample(nrow(policy_data), size = 0.8 * nrow(policy_data))
train_data <- policy_data[train_index, ]
test_data <- policy_data[-train_index, ]
```
接着,我们可以使用 Keras 来建立孪生网络模型。孪生网络模型包含两个完全相同的子网络,每个子网络都包含一个嵌入(embedding)层和一个双向 LSTM 层。嵌入层用于将文本转换为向量表示,LSTM 层用于从文本中提取特征。两个子网络的输出向量会通过 L1 距离进行比较,得到最终的相似度得分。
以下是孪生网络模型的代码示例:
```
library(keras)
# 定义模型输入
input_a <- layer_input(shape = c(1, max_length))
input_b <- layer_input(shape = c(1, max_length))
# 定义嵌入层
embedding_layer <- layer_embedding(input_dim = vocab_size, output_dim = embedding_dim,
input_length = max_length)
# 定义 LSTM 层
lstm_layer <- layer_lstm(units = lstm_units)
# 定义子网络 A
a <- input_a %>% embedding_layer %>% lstm_layer
# 定义子网络 B
b <- input_b %>% embedding_layer %>% lstm_layer
# 计算 L1 距离
distance <- layer_lambda(function(x) abs(x[[1]] - x[[2]]))
distance_output <- distance(list(a, b))
# 构建模型
model <- keras_model(inputs = list(input_a, input_b), outputs = distance_output)
```
以上代码中,`max_length` 是政策文本中最长的句子长度,`vocab_size` 是词汇表的大小,`embedding_dim` 是嵌入层的维度,`lstm_units` 是 LSTM 层的单元数。可以根据实际情况进行调整。
在模型训练时,我们需要将政策文本和政策工具标签转换为数字表示,并将其输入到孪生网络模型中进行训练:
```
# 将政策文本转换为数字表示
tokenizer <- text_tokenizer(num_words = vocab_size)
tokenizer$fit_on_texts(policy_data$policy_text)
train_sequences_a <- tokenizer$texts_to_sequences(train_data$policy_text)
test_sequences_a <- tokenizer$texts_to_sequences(test_data$policy_text)
# 将政策工具标签转换为数字表示
label_encoder <- label_encoder()
label_encoder$fit(policy_data$policy_tool)
train_labels <- label_encoder$transform(train_data$policy_tool)
test_labels <- label_encoder$transform(test_data$policy_tool)
# 对序列进行填充
train_sequences_a <- pad_sequences(train_sequences_a, maxlen = max_length)
test_sequences_a <- pad_sequences(test_sequences_a, maxlen = max_length)
# 将政策文本和政策工具标签拆分为两个输入
train_sequences_b <- train_sequences_a
test_sequences_b <- test_sequences_a
train_labels_a <- train_labels
train_labels_b <- train_labels
test_labels_a <- test_labels
test_labels_b <- test_labels
# 训练模型
model %>% compile(optimizer = "adam", loss = "binary_crossentropy",
metrics = c("accuracy"))
model %>% fit(x = list(train_sequences_a, train_sequences_b), y = train_labels,
epochs = 10, batch_size = 64, validation_data = list(
list(test_sequences_a, test_sequences_b), test_labels))
```
以上代码中,`pad_sequences` 函数用于对序列进行填充,使得所有序列的长度均为 `max_length`。`fit` 函数用于训练模型,其中 `x` 输入为两个政策文本序列,`y` 输入为政策工具标签。在测试集上评估模型的性能时,需要同样将政策文本和政策工具标签转换为数字表示,并计算模型的准确率、精确率、召回率等指标:
```
# 在测试集上进行预测
test_pred <- model %>% predict(list(test_sequences_a, test_sequences_b))
test_pred_label <- ifelse(test_pred > 0.5, 1, 0)
# 计算模型性能指标
accuracy <- mean(test_labels == test_pred_label)
precision <- precision(test_labels, test_pred_label)
recall <- recall(test_labels, test_pred_label)
f1_score <- f1_score(test_labels, test_pred_label)
cat("Accuracy:", accuracy, "\n")
cat("Precision:", precision, "\n")
cat("Recall:", recall, "\n")
cat("F1 score:", f1_score, "\n")
```
以上代码中,`ifelse` 函数用于将相似度得分转换为 0/1 标签,其中阈值为 0.5。`precision`、`recall` 和 `f1_score` 函数用于计算模型的准确率、精确率、召回率和 F1 得分。
相关推荐
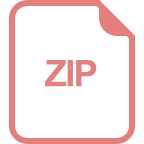
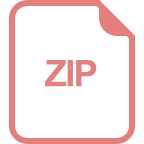
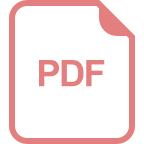







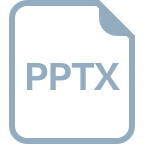
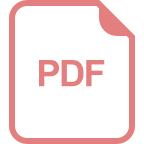