image_dst我无法识别
时间: 2023-07-26 17:16:06 浏览: 79
如果你使用 `cv::Mat` 来表示图像,则可以使用以下代码来替换 `image_dst`:
```c++
cv::Mat image_dst = cv::imread("image.jpg");
```
在上述代码中,我们使用 `cv::imread` 函数从文件中读取一张图像,并将其存储在一个名为 `image_dst` 的 `cv::Mat` 对象中。如果你已经有一个名为 `image_dst` 的 `cv::Mat` 对象,可以直接使用该对象来替换原来的 `image_dst`。
相关问题
def unzip_infer_data(src_path,target_path): ''' 解压预测数据集 ''' if(not os.path.isdir(target_path)): z = zipfile.ZipFile(src_path, 'r') z.extractall(path=target_path) z.close() def load_image(img_path): ''' 预测图片预处理 ''' img = Image.open(img_path) if img.mode != 'RGB': img = img.convert('RGB') img = img.resize((224, 224), Image.BILINEAR) img = np.array(img).astype('float32') img = img.transpose((2, 0, 1)) # HWC to CHW img = img/255 # 像素值归一化 return img infer_src_path = '/home/aistudio/data/data55032/archive_test.zip' infer_dst_path = '/home/aistudio/data/archive_test' unzip_infer_data(infer_src_path,infer_dst_path) para_state_dict = paddle.load("MyCNN") model = MyCNN() model.set_state_dict(para_state_dict) #加载模型参数 model.eval() #验证模式 #展示预测图片 infer_path='data/archive_test/alexandrite_6.jpg' img = Image.open(infer_path) plt.imshow(img) #根据数组绘制图像 plt.show() #显示图像 #对预测图片进行预处理 infer_imgs = [] infer_imgs.append(load_image(infer_path)) infer_imgs = np.array(infer_imgs) label_dic = train_parameters['label_dict'] for i in range(len(infer_imgs)): data = infer_imgs[i] dy_x_data = np.array(data).astype('float32') dy_x_data=dy_x_data[np.newaxis,:, : ,:] img = paddle.to_tensor (dy_x_data) out = model(img) lab = np.argmax(out.numpy()) #argmax():返回最大数的索引 print("第{}个样本,被预测为:{},真实标签为:{}".format(i+1,label_dic[str(lab)],infer_path.split('/')[-1].split("_")[0])) print("结束") 以上代码进行DNN预测,根据这些写GUI页面,实现输入图片并安装CNN训练结果进行对比识别,最终输出识别结果
为了实现输入图片并进行识别,你可以使用Python的GUI库来创建一个简单的界面,例如使用Tkinter库。下面是一个简单的示例代码,可以创建一个包含“选择文件”按钮和“识别”按钮的界面:
```python
import tkinter as tk
from tkinter import filedialog
from PIL import Image, ImageTk
import numpy as np
import paddle
# 加载模型参数
para_state_dict = paddle.load("MyCNN")
model = MyCNN()
model.set_state_dict(para_state_dict)
model.eval()
# 预测图片预处理
def load_image(img_path):
img = Image.open(img_path)
if img.mode != 'RGB':
img = img.convert('RGB')
img = img.resize((224, 224), Image.BILINEAR)
img = np.array(img).astype('float32')
img = img.transpose((2, 0, 1)) # HWC to CHW
img = img/255 # 像素值归一化
return img
# 打开文件选择对话框
def choose_file():
file_path = filedialog.askopenfilename()
if file_path:
img = Image.open(file_path)
img = img.resize((200, 200))
img = ImageTk.PhotoImage(img)
img_label.config(image=img)
img_label.image = img # keep a reference to prevent garbage collection
global infer_path
infer_path = file_path
# 进行预测
def predict():
try:
infer_imgs = []
infer_imgs.append(load_image(infer_path))
infer_imgs = np.array(infer_imgs)
label_dic = train_parameters['label_dict']
for i in range(len(infer_imgs)):
data = infer_imgs[i]
dy_x_data = np.array(data).astype('float32')
dy_x_data = dy_x_data[np.newaxis, :, :, :]
img = paddle.to_tensor(dy_x_data)
out = model(img)
lab = np.argmax(out.numpy()) # argmax():返回最大数的索引
result_label.config(text="识别结果为:{}".format(label_dic[str(lab)]))
except:
result_label.config(text="识别失败,请检查输入文件是否正确")
# 创建界面
root = tk.Tk()
root.title("图像识别")
# 创建“选择文件”按钮
choose_button = tk.Button(root, text="选择文件", command=choose_file)
choose_button.pack(pady=10)
# 创建图像显示标签
img_label = tk.Label(root)
img_label.pack()
# 创建“识别”按钮
predict_button = tk.Button(root, text="识别", command=predict)
predict_button.pack(pady=10)
# 创建识别结果标签
result_label = tk.Label(root, font=("Arial", 16))
result_label.pack(pady=10)
root.mainloop()
```
在这个示例代码中,我们首先定义了一个`load_image`函数来进行预处理,然后在“选择文件”按钮的回调函数`choose_file`中打开文件选择对话框,并将选择的图片显示在界面上。在“识别”按钮的回调函数`predict`中,我们将选择的图片进行预处理,然后输入模型进行预测,并将预测结果显示在界面上。注意,我们使用了`try`和`except`语句来捕获可能出现的错误,例如输入的文件格式不正确等。你可以根据自己的需求对这个示例代码进行修改和扩展。
def four_point_transform(image, pts): # obtain a consistent order of the points and unpack them # individually rect = order_points(pts) (tl, tr, br, bl) = rect # compute the width of the new image, which will be the # maximum distance between bottom-right and bottom-left # x-coordiates or the top-right and top-left x-coordinates widthA = np.sqrt(((br[0] - bl[0]) ** 2) + ((br[1] - bl[1]) ** 2)) widthB = np.sqrt(((tr[0] - tl[0]) ** 2) + ((tr[1] - tl[1]) ** 2)) maxWidth = max(int(widthA), int(widthB)) # compute the height of the new image, which will be the # maximum distance between the top-right and bottom-right # y-coordinates or the top-left and bottom-left y-coordinates heightA = np.sqrt(((tr[0] - br[0]) ** 2) + ((tr[1] - br[1]) ** 2)) heightB = np.sqrt(((tl[0] - bl[0]) ** 2) + ((tl[1] - bl[1]) ** 2)) maxHeight = max(int(heightA), int(heightB)) # now that we have the dimensions of the new image, construct # the set of destination points to obtain a "birds eye view", # (i.e. top-down view) of the image, again specifying points # in the top-left, top-right, bottom-right, and bottom-left # order dst = np.array([ [0, 0], [maxWidth - 1, 0], [maxWidth - 1, maxHeight - 1], [0, maxHeight - 1]], dtype = "float32") # compute the perspective transform matrix and then apply it M = cv2.getPerspectiveTransform(rect, dst) warped = cv2.warpPerspective(image, M, (maxWidth, maxHeight)) # return the warped image return warped
这是一个使用透视变换的函数,它可以将一个四边形区域从原图像中提取出来,并将其变换成一个矩形。主要步骤如下:
1. 对四个顶点进行排序,以确保它们按照左上、右上、右下、左下的顺序排列;
2. 计算新图像的宽度和高度,分别为原四边形区域的最大宽度和最大高度;
3. 构造目标顶点集合,将它们指定为矩形的左上、右上、右下、左下四个点;
4. 计算透视变换矩阵,并将其应用到原图像上,得到变换后的矩形图像;
5. 返回变换后的矩形图像。
这个函数可以用于许多应用,例如车牌识别、文档扫描等。
阅读全文
相关推荐
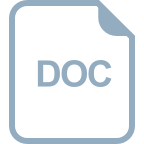
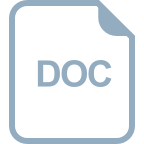
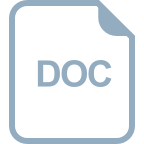
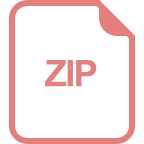
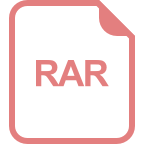
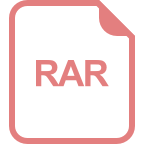
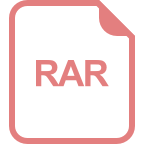
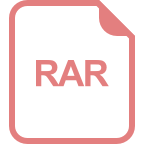
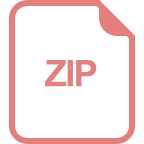
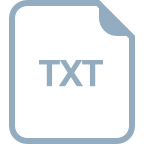
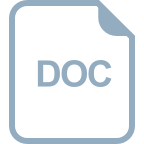
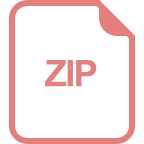
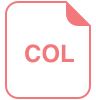
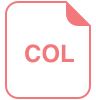
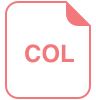
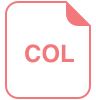
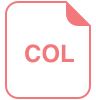
