首页解释代码 if args.distributed: if cfg.MODEL.SYNC_BN: model = nn.SyncBatchNorm.convert_sync_batchnorm(model)
解释代码 if args.distributed: if cfg.MODEL.SYNC_BN: model = nn.SyncBatchNorm.convert_sync_batchnorm(model)
时间: 2024-06-02 19:13:53 浏览: 152
这段代码的作用是在分布式训练中,将模型的批量标准化层(Batch Normalization)转换为同步批量标准化层(Sync Batch Normalization)。
在分布式训练中,每个进程在不同的GPU上运行,会独立计算梯度,并且在每个GPU上进行前向传播和反向传播。在这种情况下,如果使用普通的批量标准化层,每个GPU上的均值和方差计算是独立的,不能反映整个训练集的统计特征。这会导致模型的性能下降。
同步批量标准化层可以解决这个问题。它会收集所有GPU上的均值和方差,并进行同步更新,保证每个GPU上的批量标准化层都使用相同的统计特征。这可以提高模型的性能和收敛速度。
因此,如果在配置文件中设置了`MODEL.SYNC_BN`为True,那么就需要将模型的批量标准化层转换为同步批量标准化层。这就是这段代码的作用。如果`args.distributed`也为True,说明当前是在分布式训练模式下,需要进行这个转换。
阅读全文
CSDN会员
开通CSDN年卡参与万元壕礼抽奖
最新推荐
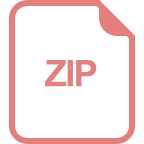
vmware workstatiions pro 17.6.1个人使用免费,不用证书直接安装使用
虚拟机vmware workstatiions pro 17.6.1个人使用免费,不用证书直接安装使用
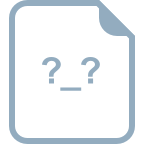
scipy-1.2.0-cp35-cp35m-linux_armv7l.whl
scipy-1.2.0-cp35-cp35m-linux_armv7l.whl
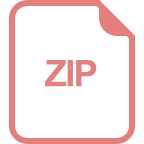
基于springboot爬虫高考志愿智能推荐系统源码数据库文档.zip
基于springboot爬虫高考志愿智能推荐系统源码数据库文档.zip
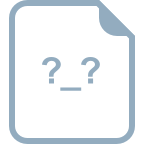
bimdata_api_client-4.3.0-py3-none-any.whl
bimdata_api_client-4.3.0-py3-none-any.whl
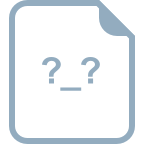
Pillow-7.0.0-cp37-cp37m-linux_armv7l.whl
Pillow-7.0.0-cp37-cp37m-linux_armv7l.whl
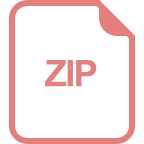
基于Python和Opencv的车牌识别系统实现
资源摘要信息:"车牌识别项目系统基于python设计"
1. 车牌识别系统概述
车牌识别系统是一种利用计算机视觉技术、图像处理技术和模式识别技术自动识别车牌信息的系统。它广泛应用于交通管理、停车场管理、高速公路收费等多个领域。该系统的核心功能包括车牌定位、车牌字符分割和车牌字符识别。
2. Python在车牌识别中的应用
Python作为一种高级编程语言,因其简洁的语法和强大的库支持,非常适合进行车牌识别系统的开发。Python在图像处理和机器学习领域有丰富的第三方库,如OpenCV、PIL等,这些库提供了大量的图像处理和模式识别的函数和类,能够大大提高车牌识别系统的开发效率和准确性。
3. OpenCV库及其在车牌识别中的应用
OpenCV(Open Source Computer Vision Library)是一个开源的计算机视觉和机器学习软件库,提供了大量的图像处理和模式识别的接口。在车牌识别系统中,可以使用OpenCV进行图像预处理、边缘检测、颜色识别、特征提取以及字符分割等任务。同时,OpenCV中的机器学习模块提供了支持向量机(SVM)等分类器,可用于车牌字符的识别。
4. SVM(支持向量机)在字符识别中的应用
支持向量机(SVM)是一种二分类模型,其基本模型定义在特征空间上间隔最大的线性分类器,间隔最大使它有别于感知机;SVM还包括核技巧,这使它成为实质上的非线性分类器。SVM算法的核心思想是找到一个分类超平面,使得不同类别的样本被正确分类,且距离超平面最近的样本之间的间隔(即“间隔”)最大。在车牌识别中,SVM用于字符的分类和识别,能够有效地处理手写字符和印刷字符的识别问题。
5. EasyPR在车牌识别中的应用
EasyPR是一个开源的车牌识别库,它的c++版本被广泛使用在车牌识别项目中。在Python版本的车牌识别项目中,虽然项目描述中提到了使用EasyPR的c++版本的训练样本,但实际上OpenCV的SVM在Python中被用作车牌字符识别的核心算法。
6. 版本信息
在项目中使用的软件环境信息如下:
- Python版本:Python 3.7.3
- OpenCV版本:opencv*.*.*.**
- Numpy版本:numpy1.16.2
- GUI库:tkinter和PIL(Pillow)5.4.1
以上版本信息对于搭建运行环境和解决可能出现的兼容性问题十分重要。
7. 毕业设计的意义
该项目对于计算机视觉和模式识别领域的初学者来说,是一个很好的实践案例。它不仅能够让学习者在实践中了解车牌识别的整个流程,而且能够锻炼学习者利用Python和OpenCV等工具解决问题的能力。此外,该项目还提供了一定量的车牌标注图片,这在数据不足的情况下尤其宝贵。
8. 文件信息
本项目是一个包含源代码的Python项目,项目代码文件位于一个名为"Python_VLPR-master"的压缩包子文件中。该文件中包含了项目的所有源代码文件,代码经过详细的注释,便于理解和学习。
9. 注意事项
尽管该项目为初学者提供了便利,但识别率受限于训练样本的数量和质量,因此在实际应用中可能存在一定的误差,特别是在处理复杂背景或模糊图片时。此外,对于中文字符的识别,第一个字符的识别误差概率较大,这也是未来可以改进和优化的方向。

管理建模和仿真的文件
管理Boualem Benatallah引用此版本:布阿利姆·贝纳塔拉。管理建模和仿真。约瑟夫-傅立叶大学-格勒诺布尔第一大学,1996年。法语。NNT:电话:00345357HAL ID:电话:00345357https://theses.hal.science/tel-003453572008年12月9日提交HAL是一个多学科的开放存取档案馆,用于存放和传播科学研究论文,无论它们是否被公开。论文可以来自法国或国外的教学和研究机构,也可以来自公共或私人研究中心。L’archive ouverte pluridisciplinaire
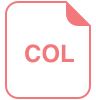
网络隔离与防火墙策略:防御网络威胁的终极指南

# 1. 网络隔离与防火墙策略概述
## 网络隔离与防火墙的基本概念
网络隔离与防火墙是网络安全中的两个基本概念,它们都用于保护网络不受恶意攻击和非法入侵。网络隔离是通过物理或逻辑方式,将网络划分为几个互不干扰的部分,以防止攻击的蔓延和数据的泄露。防火墙则是设置在网络边界上的安全系统,它可以根据预定义的安全规则,对进出网络

在密码学中,对称加密和非对称加密有哪些关键区别,它们各自适用于哪些场景?
在密码学中,对称加密和非对称加密是两种主要的加密方法,它们在密钥管理、计算效率、安全性以及应用场景上有显著的不同。
参考资源链接:[数缘社区:密码学基础资源分享平台](https://wenku.csdn.net/doc/7qos28k05m?spm=1055.2569.3001.10343)
对称加密使用相同的密钥进行数据的加密和解密。这种方法的优点在于加密速度快,计算效率高,适合大量数据的实时加密。但由于加密和解密使用同一密钥,密钥的安全传输和管理就变得十分关键。常见的对称加密算法包括AES(高级加密标准)、DES(数据加密标准)、3DES(三重数据加密算法)等。它们通常适用于那些需要
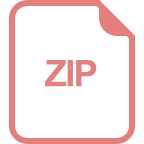
我的代码小部件库:统计、MySQL操作与树结构功能
资源摘要信息:"leetcode用例构造-my-widgets是作者为练习、娱乐或实现某些项目功能而自行开发的一个代码小部件集合。这个集合中包含了作者使用Python语言编写的几个实用的小工具模块,每个模块都具有特定的功能和用途。以下是具体的小工具模块及其知识点的详细说明:
1. statistics_from_scratch.py
这个模块包含了一些基础的统计函数实现,包括但不限于均值、中位数、众数以及四分位距等。此外,它还实现了二项分布、正态分布和泊松分布的概率计算。作者强调了使用Python标准库(如math和collections模块)来实现这些功能,这不仅有助于巩固对统计学的理解,同时也锻炼了Python编程能力。这些统计函数的实现可能涉及到了算法设计和数学建模的知识。
2. mysql_io.py
这个模块是一个Python与MySQL数据库交互的接口,它能够自动化执行数据的导入导出任务。作者原本的目的是为了将Leetcode平台上的SQL测试用例以字典格式自动化地导入到本地MySQL数据库中,从而方便在本地测试SQL代码。这个模块中的MysqlIO类支持将MySQL表导出为pandas.DataFrame对象,也能够将pandas.DataFrame对象导入为MySQL表。这个工具的应用场景可能包括数据库管理和数据处理,其内部可能涉及到对数据库API的调用、pandas库的使用、以及数据格式的转换等编程知识点。
3. tree.py
这个模块包含了与树结构相关的一系列功能。它目前实现了二叉树节点BinaryTreeNode的构建,并且提供了从列表构建二叉树的功能。这可能涉及到数据结构和算法中的树形结构、节点遍历、树的构建和操作等。利用这些功能,开发者可以在实际项目中实现更高效的数据存储和检索机制。
以上三个模块构成了my-widgets库的核心内容,它们都以Python语言编写,并且都旨在帮助开发者在特定的编程场景中更加高效地完成任务。这些工具的开发和应用都凸显了作者通过实践提升编程技能的意图,并且强调了开源精神,即将这些工具共享给更广泛的开发者群体,以便他们也能够从中受益。
通过这些小工具的使用,开发者可以更好地理解编程在不同场景下的应用,并且通过观察和学习作者的代码实现,进一步提升自己的编码水平和问题解决能力。"