verilog实现TLBO算法
时间: 2023-10-12 21:10:43 浏览: 38
TLBO(Teaching-Learning-Based Optimization)算法是一种基于群体智能的优化算法,可以用来解决各种问题。在Verilog中实现TLBO算法需要以下步骤:
1. 定义问题:确定要优化的目标函数以及变量的范围和约束条件。
2. 初始化种群:定义种群大小和每个个体的变量值。
3. 计算适应度函数:根据目标函数计算每个个体的适应度值。
4. 进行Teaching阶段:选择适应度最好的个体作为老师,根据老师的变量值更新其他个体的变量值。
5. 进行Learning阶段:对于每个个体,从种群中选择一个不同于自己的个体作为学生,根据学生的变量值更新自己的变量值。
6. 更新适应度函数:根据新的变量值计算每个个体的适应度值。
7. 判断是否达到停止条件:判断是否满足停止条件,如果满足则输出最优解,否则回到第4步进行下一轮迭代。
以下是一个简单的Verilog代码实现:
```verilog
module TLBO (
input clk,
input reset,
output reg [7:0] best_solution
);
parameter POP_SIZE = 10; // 种群大小
parameter MAX_ITER = 100; // 最大迭代次数
parameter F_LOW = 0; // 变量最低值
parameter F_HIGH = 255; // 变量最高值
parameter F_TARGET = 128; // 目标值
reg [7:0] population [POP_SIZE-1:0]; // 种群变量值
reg [7:0] fitness [POP_SIZE-1:0]; // 种群适应度值
reg [7:0] teacher; // 老师个体
reg [3:0] teacher_index; // 老师个体下标
initial begin
// 初始化种群
for (int i = 0; i < POP_SIZE; i = i + 1) begin
population[i] = $random(F_LOW, F_HIGH);
end
end
always @(posedge clk) begin
if (reset) begin
best_solution <= 0;
end
else begin
// 计算适应度函数
for (int i = 0; i < POP_SIZE; i = i + 1) begin
fitness[i] <= abs(population[i] - F_TARGET);
end
// Teaching阶段
teacher_index <= 0;
for (int i = 1; i < POP_SIZE; i = i + 1) begin
if (fitness[i] < fitness[teacher_index]) begin
teacher_index <= i;
end
end
teacher <= population[teacher_index];
for (int i = 0; i < POP_SIZE; i = i + 1) begin
if (i != teacher_index) begin
population[i] <= population[i] + $random(-2, 2) * (teacher - population[i]);
end
end
// Learning阶段
for (int i = 0; i < POP_SIZE; i = i + 1) begin
int student_index = $random(0, POP_SIZE-1);
while (student_index == i) begin
student_index = $random(0, POP_SIZE-1);
end
if (fitness[student_index] < fitness[i]) begin
population[i] <= population[i] + $random(-2, 2) * (population[student_index] - population[i]);
end
end
// 更新适应度函数
for (int i = 0; i < POP_SIZE; i = i + 1) begin
fitness[i] <= abs(population[i] - F_TARGET);
end
// 判断是否达到停止条件
if (fitness[teacher_index] < fitness[best_solution]) begin
best_solution <= teacher_index;
end
if ($time > MAX_ITER) begin
$display("Best solution: %d", population[best_solution]);
$finish;
end
end
end
endmodule
```
在这个代码中,我们使用了Verilog中的随机函数$random来产生随机数,实现了TLBO算法的Teaching和Learning阶段,并且在每轮迭代结束后判断是否满足停止条件。最后,我们使用$display输出最优解,并且使用$finish停止仿真。注意,这个代码只是一个简单的实现,可能需要根据具体问题进行修改。
相关推荐
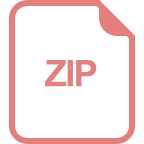













