import matplotlib.colors as mcolors colors=list(mcolors.TABLEAU_COLORS.keys()) # 定义可视化函数 def vis_result_2D(df,label): fig = plt.figure(figsize=(16,6)) plt.rcParams['font.sans-serif'] = ['SimHei'] plt.rcParams['axes.unicode_minus'] = False ax = fig.add_subplot(121) for i in df['label'].unique(): temp_df = df[df['label']==i] ax.scatter(temp_df['WindSpeed'],temp_df['Power'],s=0.3,color=mcolors.TABLEAU_COLORS[colors[i]],label=i) plt.legend() plt.title("各类异常的分布") plt.grid(True) ax = fig.add_subplot(122) df = df[df['label']==0] ax.scatter(df['WindSpeed'],df['Power'],s=0.3) plt.title("清洗后的结果") plt.grid(True) plt.show()详细解释
时间: 2024-01-30 20:02:03 浏览: 25
这段代码主要用于可视化数据集的聚类结果,具体解释如下:
1. 导入 matplotlib.colors 模块,并将所有 TABLEAU_COLORS 的 key 存储在 colors 列表中。
2. 定义了一个名为 vis_result_2D 的可视化函数,该函数接受两个参数,一个是数据集 df,另一个是聚类的标签 label。
3. 创建一个大小为 16x6 的图形窗口,并设置字体和负轴。
4. 在左边的子图中,循环遍历聚类标签的唯一值,对于每个标签,将数据集中标签值等于该标签值的数据筛选出来,并使用 scatter 函数将其可视化到图中,每个标签使用不同的颜色。同时,添加图例、标题和网格线。
5. 在右边的子图中,筛选出标签值等于 0 的数据,使用 scatter 函数将其可视化到图中,不添加图例,添加标题和网格线。
6. 显示图形窗口。
相关问题
import numpy as np import matplotlib.pyplot as plt from mpl_toolkits.mplot3d
import numpy是一个用于科学计算的Python库,它提供了大量用于数组操作和数学计算的函数和工具。使用import numpy as np将numpy库导入为np别名,以便在代码中更方便地使用numpy的函数和工具。
而import matplotlib.pyplot as plt是导入matplotlib库的一种常用方式,matplotlib是一个用于绘制图表和数据可视化的库,import语句将matplotlib.pyplot模块导入为plt别名,以便在代码中更方便地使用matplotlib库的绘图函数和工具。
最后,from mpl_toolkits.mplot3d导入模块同样是用于matplotlib库的一种常见操作,它用于导入mpl_toolkits.mplot3d模块,这是一个用于绘制三维图表和可视化三维数据的工具模块。
综合起来,这些import语句在Python代码中的作用是将numpy和matplotlib库以及其相关的模块导入到代码中,以便使用它们提供的各种函数和工具进行数组操作、数学计算和绘制图表等操作。这样可以极大地方便数据科学家、工程师和研究人员们对数据的处理和可视化工作。
data = np.random.rand(80640, 3)使用import matplotlib.pyplot as plt可视化
可以使用 Matplotlib 库将数组可视化,示例代码如下:
```python
import numpy as np
import matplotlib.pyplot as plt
# 生成数据
data = np.random.rand(80640, 3)
# 取出x,y,z坐标
x = data[:, 0]
y = data[:, 1]
z = data[:, 2]
# 绘制散点图
fig = plt.figure()
ax = fig.add_subplot(111, projection='3d')
ax.scatter(x, y, z, s=1, c='b', marker='.')
plt.show()
```
该示例代码将数组可视化为三维散点图,其中x、y、z坐标分别为数组的三个维度,s参数设置散点大小,c参数设置散点颜色,marker参数设置散点形状。你可以根据自己的需求调整这些参数。
相关推荐
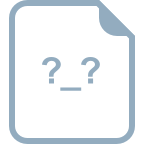
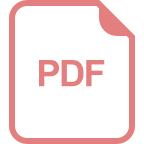












