编写pytorch代码,定义SSAE模型,读入数据集,将数据时间列转换为日期时间数据类型,将包含时间列在内的特征分到特征集,将最后一列放到标签集
时间: 2024-03-26 16:34:18 浏览: 65
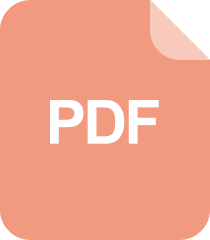
Pytorch 实现数据集自定义读取
好的,我可以帮你实现这个需求。首先,我们需要导入必要的库和模块:
```python
import torch
import torch.nn as nn
import pandas as pd
import numpy as np
from sklearn.preprocessing import MinMaxScaler
```
然后,定义SSAE模型:
```python
class SSAE(nn.Module):
def __init__(self, input_size, hidden_size, output_size):
super(SSAE, self).__init__()
self.encoder = nn.Sequential(
nn.Linear(input_size, hidden_size),
nn.ReLU(),
nn.Linear(hidden_size, output_size),
nn.ReLU()
)
self.decoder = nn.Sequential(
nn.Linear(output_size, hidden_size),
nn.ReLU(),
nn.Linear(hidden_size, input_size),
nn.ReLU()
)
def forward(self, x):
x = self.encoder(x)
x = self.decoder(x)
return x
```
接着,读入数据集并将时间列转换为日期时间数据类型:
```python
data = pd.read_csv('your_data.csv')
data['time'] = pd.to_datetime(data['time'])
```
将包含时间列在内的特征分到特征集:
```python
features = data.drop(['label'], axis=1)
```
将最后一列放到标签集:
```python
labels = data['label']
```
最后,我们可以对特征集进行归一化处理:
```python
scaler = MinMaxScaler()
features = scaler.fit_transform(features)
```
这样,我们就完成了数据集的预处理。接下来,我们可以将数据集划分为训练集和测试集,然后使用SSAE模型进行训练和预测。
阅读全文
相关推荐
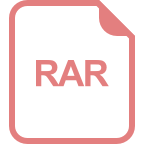
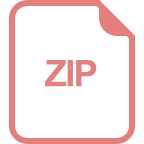
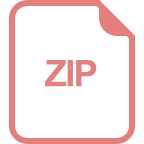
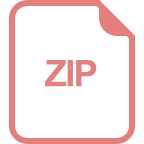
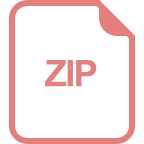
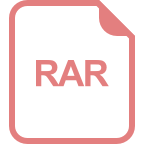
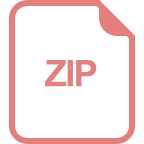
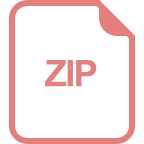
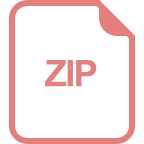
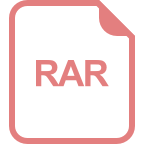
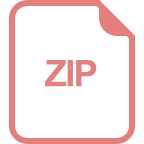
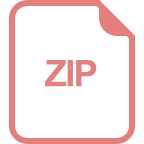
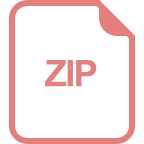
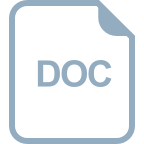