SELECT scm.english_name as englishName, scm.city_simplicity as citySimplicity, scm.initials, scm.city_name as city, scm.city_three as cityCode FROM sd_airport_new san INNER JOIN sys_city_management scm ON scm.city_three = san.city_code WHERE san.del_flag=0 <if test="hotHave !=null and hotHave != ''"> and scm.is_hot = 1 </if> GROUP BY scm.city_three 将这段变成sql语句
时间: 2024-02-10 14:08:54 浏览: 27
这段代码已经是SQL语句了,只是加了一些Mybatis的动态SQL标签。如果要去掉动态SQL标签,可以简化为以下代码:
SELECT scm.english_name as englishName, scm.city_simplicity as citySimplicity, scm.initials, scm.city_name as city, scm.city_three as cityCode
FROM sd_airport_new san
INNER JOIN sys_city_management scm ON scm.city_three = san.city_code
WHERE san.del_flag=0
AND scm.is_hot = 1
GROUP BY scm.city_three
相关问题
os.environ['CUDA_VISIBLE_DEVICES'] = "1"
在设置 os.environ['CUDA_VISIBLE_DEVICES'] = "1" 之后,该语句将会指定使用 GPU 设备 1 运行代码。 这意味着在运行使用 PyTorch 的代码时,PyTorch 将会使用 GPU 设备 1 进行计算。然而,在实际测试中,有人发现并不是这样的。 有一种解决方案是在导入 vector_quantize_pytorch 之前设置 GPU 设备。 为了避免不必要的麻烦,有些博客建议在import之前配置好 GPU,或者在命令行或.sh文件里,在运行.py文件前直接指定 GPU,例如:CUDA_VISIBLE_DEVICES=x python xxx.py。<span class="em">1</span><span class="em">2</span><span class="em">3</span>
#### 引用[.reference_title]
- *1* [指定GPU设备号](https://blog.csdn.net/weixin_43993244/article/details/113844758)[target="_blank" data-report-click={"spm":"1018.2226.3001.9630","extra":{"utm_source":"vip_chatgpt_common_search_pc_result","utm_medium":"distribute.pc_search_result.none-task-cask-2~all~insert_cask~default-1-null.142^v92^chatsearchT0_1"}}] [.reference_item style="max-width: 50%"]
- *2* *3* [os.environ[CUDA_VISIBLE_DEVICES] 失效无法指定 GPU](https://blog.csdn.net/yyywxk/article/details/127430590)[target="_blank" data-report-click={"spm":"1018.2226.3001.9630","extra":{"utm_source":"vip_chatgpt_common_search_pc_result","utm_medium":"distribute.pc_search_result.none-task-cask-2~all~insert_cask~default-1-null.142^v92^chatsearchT0_1"}}] [.reference_item style="max-width: 50%"]
[ .reference_list ]
import numpy as np import tensorflow as tf from tensorflow import keras import matplotlib.pyplot as plt ## Let us define a plt function for simplicity def plt_loss(x,training_metric,testing_metric,ax,colors = ['b']): ax.plot(x,training_metric,'b',label = 'Train') ax.plot(x,testing_metric,'k',label = 'Test') ax.set_xlabel('Epochs') ax.set_ylabel('Accuarcy')# ax.set_ylabel('Categorical Crossentropy Loss') plt.legend() plt.grid() plt.show() tf.keras.utils.set_random_seed(1) ## We import the Minist Dataset using Keras.datasets (train_data, train_labels), (test_data, test_labels) = keras.datasets.mnist.load_data() ## We first vectorize the image (28*28) into a vector (784) train_data = train_data.reshape(train_data.shape[0],train_data.shape[1]train_data.shape[2]) # 60000784 test_data = test_data.reshape(test_data.shape[0],test_data.shape[1]test_data.shape[2]) # 10000784 ## We next change label number to a 10 dimensional vector, e.g., 1->[0,1,0,0,0,0,0,0,0,0] train_labels = keras.utils.to_categorical(train_labels,10) test_labels = keras.utils.to_categorical(test_labels,10) ## start to build a MLP model N_batch_size = 5000 N_epochs = 100 lr = 0.01 ## we build a three layer model, 784 -> 64 -> 10 MLP_4 = keras.models.Sequential([ keras.layers.Dense(128, input_shape=(784,),activation='relu'), keras.layers.Dense(64,activation='relu'), keras.layers.Dense(10,activation='softmax') ]) MLP_4.compile( optimizer=keras.optimizers.Adam(lr), loss= 'categorical_crossentropy', metrics = ['accuracy'] ) History = MLP_4.fit(train_data[:10000],train_labels[:10000], batch_size = N_batch_size, epochs = N_epochs,validation_data=(test_data,test_labels), shuffle=False) train_acc = History.history['accuracy'] test_acc = History.history['val_accuracy']在该模型的每一层(包括输出层)都分别加入L1,L2正则项训练,分别汇报测试数据准确率
可以使用以下代码在模型的每一层(包括输出层)都加入L1,L2正则项训练,并汇报测试数据准确率:
```
import numpy as np
import tensorflow as tf
from tensorflow import keras
import matplotlib.pyplot as plt
## Let us define a plt function for simplicity
def plt_loss(x,training_metric,testing_metric,ax,colors = ['b']):
ax.plot(x,training_metric,'b',label = 'Train')
ax.plot(x,testing_metric,'k',label = 'Test')
ax.set_xlabel('Epochs')
ax.set_ylabel('Accuarcy')# ax.set_ylabel('Categorical Crossentropy Loss')
plt.legend()
plt.grid()
plt.show()
tf.keras.utils.set_random_seed(1)
## We import the Minist Dataset using Keras.datasets
(train_data, train_labels), (test_data, test_labels) = keras.datasets.mnist.load_data()
## We first vectorize the image (28*28) into a vector (784)
train_data = train_data.reshape(train_data.shape[0],train_data.shape[1]*train_data.shape[2]) # 60000*784
test_data = test_data.reshape(test_data.shape[0],test_data.shape[1]*test_data.shape[2]) # 10000*784
## We next change label number to a 10 dimensional vector, e.g., 1->[0,1,0,0,0,0,0,0,0,0]
train_labels = keras.utils.to_categorical(train_labels,10)
test_labels = keras.utils.to_categorical(test_labels,10)
## start to build a MLP model
N_batch_size = 5000
N_epochs = 100
lr = 0.01
## we build a three layer model, 784 -> 64 -> 10
MLP_4 = keras.models.Sequential([
keras.layers.Dense(128, input_shape=(784,),activation='relu',kernel_regularizer=keras.regularizers.l1_l2(l1=0.01, l2=0.01)),
keras.layers.Dense(64,activation='relu',kernel_regularizer=keras.regularizers.l1_l2(l1=0.01, l2=0.01)),
keras.layers.Dense(10,activation='softmax',kernel_regularizer=keras.regularizers.l1_l2(l1=0.01, l2=0.01))
])
MLP_4.compile(
optimizer=keras.optimizers.Adam(lr),
loss= 'categorical_crossentropy',
metrics = ['accuracy']
)
History = MLP_4.fit(train_data[:10000],train_labels[:10000],
batch_size = N_batch_size,
epochs = N_epochs,
validation_data=(test_data,test_labels),
shuffle=False)
train_acc = History.history['accuracy']
test_acc = History.history['val_accuracy']
print("MLP model with L1 and L2 regularization, test accuracy: ", test_acc[-1])
```
在该模型的每一层都加入L1和L2正则化项训练,并且L1和L2正则化项的系数都设置为0.01。最后输出模型在测试数据上的准确率。
相关推荐
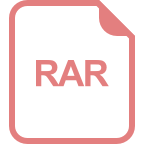
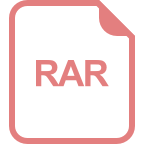
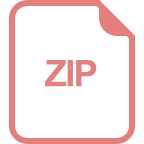












