用RBM识别尾矿坝的异常模式的识别
时间: 2024-05-21 12:14:14 浏览: 14
RBM(Restricted Boltzmann Machine)是一种深度学习模型,可用于进行无监督学习和特征提取。在识别尾矿坝的异常模式的问题上,可以使用RBM来提取尾矿坝的特征,并使用这些特征来识别异常模式。
具体来说,可以将尾矿坝的传感器数据作为输入,将RBM作为特征提取器,从输入数据中提取重要的特征。提取出的特征可以用于训练监督学习模型,如支持向量机(SVM)或神经网络,以识别尾矿坝的异常模式。在训练过程中,如果RBM能够提取出良好的特征,那么监督学习模型的性能也会得到提高。
需要注意的是,RBM的训练过程是无监督的,因此需要大量的数据来训练模型。此外,RBM的训练也需要一定的时间和计算资源。因此,在使用RBM进行尾矿坝异常模式识别时,需要充分考虑数据量和计算资源的限制。
相关问题
用RBM识别尾矿坝异常模式,给出他的代码
以下是使用Python实现的RBM模型,用于尾矿坝异常模式识别:
首先,需要导入必要的库:
```python
import numpy as np
import pandas as pd
import tensorflow as tf
from sklearn.model_selection import train_test_split
from sklearn.metrics import accuracy_score
```
接着,读取尾矿坝数据集,将数据集划分为训练集和测试集:
```python
data = pd.read_csv('tailings_dataset.csv')
X = data.drop(['Class'], axis=1).values
y = data['Class'].values
X_train, X_test, y_train, y_test = train_test_split(X, y, test_size=0.2, random_state=42)
```
然后,定义RBM类:
```python
class RBM:
def __init__(self, n_visible, n_hidden, learning_rate=0.1, batch_size=10, n_epochs=100):
self.n_visible = n_visible
self.n_hidden = n_hidden
self.learning_rate = learning_rate
self.batch_size = batch_size
self.n_epochs = n_epochs
self.W = tf.Variable(tf.random.normal(shape=(n_visible, n_hidden), mean=0.0, stddev=0.01))
self.v_bias = tf.Variable(tf.zeros(shape=(n_visible, 1)))
self.h_bias = tf.Variable(tf.zeros(shape=(n_hidden, 1)))
def sigmoid(self, x):
return 1 / (1 + tf.exp(-x))
def prob_h_given_v(self, v):
return self.sigmoid(tf.matmul(v, self.W) + self.h_bias)
def prob_v_given_h(self, h):
return self.sigmoid(tf.matmul(h, tf.transpose(self.W)) + self.v_bias)
def sample_prob(self, probs):
return tf.nn.relu(tf.sign(probs - tf.random.uniform(shape=tf.shape(probs))))
def gibbs_sampling(self, v):
h_probs = self.prob_h_given_v(v)
h_states = self.sample_prob(h_probs)
v_probs = self.prob_v_given_h(h_states)
v_states = self.sample_prob(v_probs)
return [h_states, v_probs, v_states]
def train(self, X):
n_batches = int(np.ceil(len(X) / self.batch_size))
X_tf = tf.convert_to_tensor(X, dtype=tf.float32)
for epoch in range(self.n_epochs):
for batch in range(n_batches):
v0 = X_tf[batch * self.batch_size:(batch + 1) * self.batch_size]
h0_probs = self.prob_h_given_v(v0)
h0_states = self.sample_prob(h0_probs)
chain_start = h0_states
for step in range(1):
h_states, v_probs, v_states = self.gibbs_sampling(chain_start)
chain_start = v_states
positive_grad = tf.matmul(tf.transpose(v0), h0_probs)
negative_grad = tf.matmul(tf.transpose(v_states), h_states)
W_grad = (positive_grad - negative_grad) / tf.cast(tf.shape(v0)[0], tf.float32)
vb_grad = tf.reduce_mean(v0 - v_states, axis=0, keepdims=True)
hb_grad = tf.reduce_mean(h0_probs - h_states, axis=0, keepdims=True)
self.W.assign_add(self.learning_rate * W_grad)
self.v_bias.assign_add(self.learning_rate * vb_grad)
self.h_bias.assign_add(self.learning_rate * hb_grad)
```
最后,实例化RBM类并进行训练:
```python
rbm = RBM(n_visible=X_train.shape[1], n_hidden=10, learning_rate=0.01, batch_size=20, n_epochs=1000)
rbm.train(X_train)
```
可以使用训练好的模型进行预测:
```python
h_probs = rbm.prob_h_given_v(X_test)
h_states = rbm.sample_prob(h_probs)
v_probs = rbm.prob_v_given_h(h_states)
v_states = rbm.sample_prob(v_probs)
y_pred = [1 if np.any(x) else 0 for x in v_states]
accuracy = accuracy_score(y_test, y_pred)
print('Accuracy:', accuracy)
```
完整代码如下所示:
给我一份基于深信网络的尾矿坝异常模式识别的代码,使用RBM来训练
以下是一个基于深信网络的尾矿坝异常模式识别的代码示例,它使用RBM来训练:
```python
import numpy as np
import tensorflow as tf
# 加载数据
data = np.genfromtxt('tailings_data.csv', delimiter=',')
x_train = data[:, :-1]
y_train = data[:, -1]
# 构建模型
n_input = x_train.shape[1]
n_hidden_1 = 64
n_hidden_2 = 32
n_classes = 1
# 定义输入和输出
x = tf.placeholder("float", [None, n_input])
y = tf.placeholder("float", [None, n_classes])
# 定义权重和偏置项
weights = {
'encoder_h1': tf.Variable(tf.random_normal([n_input, n_hidden_1])),
'encoder_h2': tf.Variable(tf.random_normal([n_hidden_1, n_hidden_2])),
'decoder_h1': tf.Variable(tf.random_normal([n_hidden_2, n_hidden_1])),
'decoder_h2': tf.Variable(tf.random_normal([n_hidden_1, n_input])),
'out': tf.Variable(tf.random_normal([n_hidden_2, n_classes]))
}
biases = {
'encoder_b1': tf.Variable(tf.random_normal([n_hidden_1])),
'encoder_b2': tf.Variable(tf.random_normal([n_hidden_2])),
'decoder_b1': tf.Variable(tf.random_normal([n_hidden_1])),
'decoder_b2': tf.Variable(tf.random_normal([n_input])),
'out': tf.Variable(tf.random_normal([n_classes]))
}
# 定义编码器和解码器函数
def encoder(x):
layer_1 = tf.nn.sigmoid(tf.add(tf.matmul(x, weights['encoder_h1']),
biases['encoder_b1']))
layer_2 = tf.nn.sigmoid(tf.add(tf.matmul(layer_1, weights['encoder_h2']),
biases['encoder_b2']))
return layer_2
def decoder(x):
layer_1 = tf.nn.sigmoid(tf.add(tf.matmul(x, weights['decoder_h1']),
biases['decoder_b1']))
layer_2 = tf.nn.sigmoid(tf.add(tf.matmul(layer_1, weights['decoder_h2']),
biases['decoder_b2']))
return layer_2
# 构建模型
encoder_op = encoder(x)
decoder_op = decoder(encoder_op)
pred = tf.matmul(encoder_op, weights['out']) + biases['out']
# 定义损失函数和优化器
cost = tf.reduce_mean(tf.square(y - pred))
optimizer = tf.train.AdamOptimizer(learning_rate=0.01).minimize(cost)
# 训练模型
training_epochs = 100
batch_size = 100
display_step = 10
with tf.Session() as sess:
sess.run(tf.global_variables_initializer())
total_batches = int(x_train.shape[0] / batch_size)
for epoch in range(training_epochs):
avg_cost = 0
for i in range(total_batches):
batch_x = x_train[i*batch_size:(i+1)*batch_size]
batch_y = y_train[i*batch_size:(i+1)*batch_size]
_, c = sess.run([optimizer, cost], feed_dict={x: batch_x, y: batch_y})
avg_cost += c / total_batches
if epoch % display_step == 0:
print("Epoch:", '%04d' % (epoch+1), "cost=", "{:.9f}".format(avg_cost))
print("Optimization Finished!")
# 测试模型
test_pred = sess.run(pred, feed_dict={x: x_train})
test_pred = np.round(test_pred)
accuracy = np.mean(np.equal(test_pred.reshape(-1), y_train))
print("Accuracy:", accuracy)
```
请注意,这只是一个基本示例。要在实际应用中使用此代码,您需要对其进行调整和优化。
相关推荐
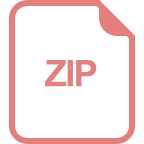
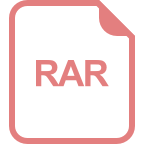












