matlab Astar二维最优路径
时间: 2024-06-29 15:00:42 浏览: 6
A*算法是一种启发式搜索算法,常用于寻找两点之间的最短路径,尤其是在二维网格环境中,如Matlab中的Astar寻路。A*算法结合了Dijkstra算法(寻找最短路径)和简单的启发式函数,可以有效地解决复杂的路径规划问题。
在Matlab中,实现A*算法的一般步骤包括:
1. 初始化:定义地图(二维矩阵表示,其中0通常代表空地,1或其它值代表障碍物),起始点和目标点。
2. 定义状态节点:每个节点由一个坐标(x, y)和一个cost(到起点的成本)和 heuristic(启发式估计到目标点的成本)组成。
3. 创建开放列表和关闭列表:开放列表包含待探索的节点,关闭列表用于存储已经访问过的节点。
4. 开始搜索:将起始点加入开放列表,然后循环执行以下步骤直到找到目标点或者开放列表为空:
a. 从开放列表中选择cost + heuristic最小的节点(即最佳节点)。
b. 如果该节点是目标点,返回路径。
c. 否则,将该节点从开放列表移除并加入关闭列表,然后对其相邻节点执行以下操作:
i. 计算新节点的cost(父节点cost加上到当前节点的代价)。
ii. 如果该节点不在关闭列表中,或者通过当前节点到达的成本更低,更新该节点的cost和父节点,并将其加入开放列表。
5. 结果处理:如果找不到路径,说明目标点不可达。
相关问题
astar二维路径规划代码
A*算法是一种常用的寻路算法,适用于二维地图上的路径规划。它的核心思想是启发式搜索,通过估计当前节点到目标节点的距离来选择下一个节点,从而找到最短路径。
A*算法主要包含以下几个步骤:
1. 初始化起点和终点,放入开启列表中。
2. 从开启列表中找到F值最小的节点作为当前节点,并将其放入闭合列表中。如果当前节点是终点,则结束搜索。
3. 对当前节点的邻居进行遍历,对每个邻居节点计算G值和H值,并计算出F值。
4. 如果邻居节点不在开启列表中,则将其加入开启列表中,并记录其父节点为当前节点。
5. 如果邻居节点已经在开启列表中,比较新的G值与旧的G值大小,如果新的G值更小,则更新邻居节点的父节点。
6. 重复以上步骤,直到找到终点或开启列表为空。
下面是一个简单的A*算法的伪代码:
open_list = {起点};
closed_list = {};
while (open_list is not empty) {
current_node = node with lowest F value in open_list;
if (current_node == 终点) {
return path;
}
remove current_node from open_list;
add current_node to closed_list;
for each neighbor_node of current_node {
if (neighbor_node is not walkable or neighbor_node in closed_list) {
continue;
}
new_G = current_node.G + distance(current_node, neighbor_node);
if (neighbor_node not in open_list or new_G < neighbor_node.G) {
neighbor_node.G = new_G;
neighbor_node.H = heuristic(neighbor_node, 终点);
neighbor_node.F = neighbor_node.G + neighbor_node.H;
neighbor_node.parent = current_node;
if (neighbor_node not in open_list) {
add neighbor_node to open_list;
}
}
}
}
return no path found;
以上是A*算法的核心代码,其中distance函数用于计算两个节点之间的距离,heuristic函数用于估计当前节点到终点的距离。在实际应用中,还需要根据实际需求对算法进行优化和改进。
基于astar算法的栅格地图最优路径搜索matlab仿真,可以修改任意数量栅格
基于Astar算法的栅格地图最优路径搜索Matlab仿真,是一种常见的路径规划方法。该算法可以在复杂的地形或者地图上实现最优的路径搜索。在这种算法中,地图被划分为许多网格,每个网格有自己的代价值。代价值代表了该网格被穿越的难度,例如高山或河流会增加代价值,而平地则是较低的代价值。
在Matlab中,可以借助编程语言来编写基于Astar算法的栅格地图最优路径搜索仿真程序。首先,需要通过Matlab的图形用户界面(GUI)来创建一个栅格地图。可以通过该界面来添加、删除或者修改地图中的栅格。接下来,需要将地图转换为一个类似于矩阵的数据结构,使得每个栅格都对应到一个元素。然后,根据Astar算法的原理,可以计算出每个栅格到起点和终点的距离,构建出一个距离矩阵。通过距离矩阵,可以执行最优路径搜索,并输出路径点序列或者路径规划图。
由于该算法的可扩展性,可以修改任意数量的栅格,从而更加准确地模拟真实环境中的求解问题,例如在实际环境中存在的建筑、汽车或者其他形状不规则的物体。此外,该算法还具有较高的路径求解效率,可以快速地生成最优路径规划方案。综上所述,基于Astar算法的栅格地图最优路径搜索Matlab仿真是一个非常实用的工具,可以满足许多路径规划应用的需求。
相关推荐
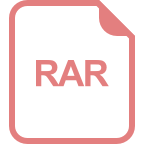
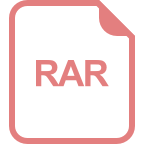
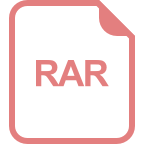












