ind = find(desc<0); stepmax = min(-(SigmaNew(ind))./desc(ind)); deltmax = stepmax; if isempty(stepmax) || stepmax==0 Sigma = SigmaNew; return end if stepmax > 0.1 stepmax=0.1; end %----------------------------------------------------- % Projected gradient %----------------------------------------------------- while costmax<costmin [costmax, S] = costgraph(KH,stepmax,desc,SigmaNew); if costmax<costmin costmin = costmax; SigmaNew = SigmaNew + stepmax * desc; %------------------------------- % Numerical cleaning %------------------------------- % SigmaNew(find(abs(SigmaNew<option.numericalprecision)))=0; % SigmaNew=SigmaNew/sum(SigmaNew); % SigmaNew =SigmaP; % project descent direction in the new admissible cone % keep the same direction of descent while cost decrease %desc = desc .* ( (SigmaNew>0) | (desc>0) ) ; desc = desc .* ( (SigmaNew>option.numericalprecision)|(desc>0)); desc(coord) = - sum(desc([[1:coord-1] [coord+1:end]])); ind = find(desc<0); if ~isempty(ind) stepmax = min(-(SigmaNew(ind))./desc(ind)); deltmax = stepmax; costmax = 0; else stepmax = 0; deltmax = 0; end end end %----------------------------------------------------- % Linesearch %----------------------------------------------------- Step = [stepmin stepmax]; Cost = [costmin costmax]; [val,coord] = min(Cost); % optimization of stepsize by golden search while (stepmax-stepmin)>option.goldensearch_deltmax*(abs(deltmax)) && stepmax > eps stepmedr = stepmin+(stepmax-stepmin)/gold; stepmedl = stepmin+(stepmedr-stepmin)/gold; [costmedr, S1] = costgraph(KH,stepmedr,desc,SigmaNew); [costmedl, S2] = costgraph(KH,stepmedl,desc,SigmaNew); Step = [stepmin stepmedl stepmedr stepmax]; Cost = [costmin costmedl costmedr costmax]; [val,coord] = min(Cost); switch coord case 1 stepmax = stepmedl; costmax = costmedl; S = S2; case 2 stepmax = stepmedr; costmax = costmedr; S = S2; case 3 stepmin = stepmedl; costmin = costmedl; S = S2; case 4 stepmin = stepmedr; costmin = costmedr; S = S1; end end %--------------------------------- % Final Updates %--------------------------------- CostNew = Cost(coord); step = Step(coord); % Sigma update if CostNew < CostOld SigmaNew = SigmaNew + step * desc; end Sigma = SigmaNew;
时间: 2023-08-15 17:12:26 浏览: 61
这段代码是一个优化算法的实现,具体来说是一个基于梯度下降和线搜索的优化算法。它的主要目的是在给定的约束条件下,最小化一个代价函数。这个代价函数是通过调用 costgraph 函数计算得出的,其中的参数包括 KH、stepmax、desc 和 SigmaNew。
在这个算法中,首先计算出一个初始的步长 stepmax,然后根据这个步长进行梯度下降。在下降的过程中,通过比较当前得到的代价函数值和之前的最小代价函数值,来判断是否需要继续下降。如果代价函数值继续减小,则更新 SigmaNew,并重新计算下降的步长 stepmax。如果代价函数值不再减小,则进行线搜索,找到一个更小的代价函数值对应的步长,然后再进行梯度下降。这个过程一直持续,直到找到一个满足收敛条件的最小代价函数值。
除了梯度下降和线搜索,这个算法还包括其他一些细节处理,例如数值清理、梯度方向的更新等等。这些处理可以提高算法的稳定性和收敛速度。
相关问题
解释这段代码:ind = find(desc<0); stepmax = min(-(SigmaNew(ind))./desc(ind)); deltmax = stepmax; if isempty(stepmax) || stepmax==0 Sigma = SigmaNew; return end if stepmax > 0.1 stepmax=0.1; end
这段代码涉及到一个最优化算法中的步长选择问题,以下是对代码的解释:
1. `ind = find(desc<0);`:找到梯度向量中所有元素值小于0的位置,将这些位置的下标保存在`ind`中。
2. `stepmax = min(-(SigmaNew(ind))./desc(ind));`:计算可以沿着梯度方向移动的最大步长,这个步长应该是使得目标函数下降最快的步长。具体来说,这里使用了一种简单的线搜索方法,即按比例缩放梯度向量,使其长度等于步长。其中,`SigmaNew`是一个向量,`SigmaNew(ind)`表示`SigmaNew`中下标为`ind`的元素,`desc`也是一个向量,`desc(ind)`表示`desc`中下标为`ind`的元素。
3. `deltmax = stepmax;`:将步长赋值给`deltmax`,在接下来的代码中会用到。
4. `if isempty(stepmax) || stepmax==0`:判断步长是否为空或者为0,如果是,则说明当前位置已经是一个极小值点,直接返回。
5. `Sigma = SigmaNew;`:将`SigmaNew`复制给`Sigma`。
6. `return`:返回结果。
7. `if stepmax > 0.1`:如果计算出来的步长大于0.1,则将步长截断为0.1。
8. `stepmax=0.1;`:将步长赋值为0.1。这个值是一个经验值,如果步长太大,可能会导致算法不稳定,所以将其截断为一个较小的值。
解释这段代码:ind = find(desc<0); stepmax = min(-(SigmaNew(ind))./desc(ind));
这段代码涉及到数值计算中的最大步长问题。
首先,`desc` 是一个向量,它表示当前点的梯度方向(下降方向)。`find(desc<0)` 表示找到所有 `desc` 中小于零的元素的下标,这些元素对应的方向是当前点的下降方向。
接着,`SigmaNew` 是一个向量,表示当前点在每个下降方向上的最优步长。`SigmaNew(ind)` 表示在所有下降方向中,只考虑那些在 `ind` 中出现的下降方向的最优步长。
最后,`stepmax = min(-(SigmaNew(ind))./desc(ind))` 表示在所有考虑的下降方向中,找到一个最大的可行步长,使得在这个步长内可以保证函数值单调递减。具体地,我们先将 `SigmaNew(ind)` 按照对应的下降方向的 `desc(ind)` 进行缩放,然后取这些缩放后的值中的最小值。这个最小值就是当前点能够沿着所有考虑的下降方向走的最大步长。注意到这里使用了负号,是因为我们要找到最大的可行步长,而 `SigmaNew` 表示的是在每个方向上的最优步长,所以需要取负号。
相关推荐
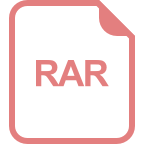
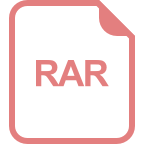


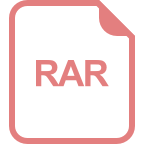
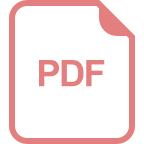
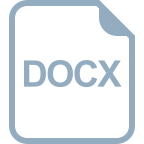
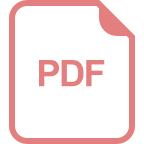
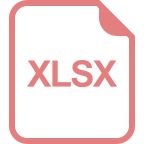
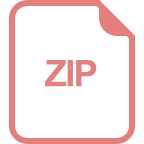
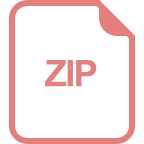