def calCrowdcarCost(crowd_route_list, model): cost_of_distance = 0 crowd_wait_time = 0 model.crowdcarcost = 0 for route in crowd_route_list: timetable = [] for i in range(len(route)): if i == 0: depot_id = route[i] next_node_id = route[i+1] cost_of_distance = model.distance_matrix[depot_id, next_node_id] travel_time = int(model.distance_matrix[depot_id, next_node_id]) departure = max(0, model.demand_dict[next_node_id].start_time - travel_time) if departure == 0: crowd_wait_time = travel_time - model.demand_dict[next_node_id].start_time timetable.append((departure, departure)) elif 1 <= i <= len(route)-2: last_node_id = route[i-1] current_node_id = route[i] current_node = model.demand_dict[current_node_id] travel_time = int(model.distance_matrix[last_node_id, current_node_id]) cost_of_distance += model.distance_matrix[last_node_id, current_node_id] arrival = max(timetable[-1][1]+travel_time, current_node.start_time) if arrival == timetable[-1][1]+travel_time: crowd_wait_time += timetable[-1][1]+travel_time - current_node.start_time else: break model.crowdcarcost += model.fixcost + model.percost * cost_of_distance return model.crowdcarcost, crowd_wait_time 在这一段代码中我想知道每个点的等待时间总和,等待时间等于到达这个的时间减去它的时间窗上限
时间: 2024-02-27 12:59:10 浏览: 33
在这段代码中,你已经计算了每个点的等待时间,它们被累加到了`crowd_wait_time`变量中。如果你想得到每个点的等待时间总和,可以在循环中添加一个变量来记录。以下是修改后的代码:
```python
def calCrowdcarCost(crowd_route_list, model):
cost_of_distance = 0
crowd_wait_time = 0
point_wait_time = {} # 记录每个点的等待时间
model.crowdcarcost = 0
for route in crowd_route_list:
timetable = []
for i in range(len(route)):
if i == 0:
depot_id = route[i]
next_node_id = route[i+1]
cost_of_distance = model.distance_matrix[depot_id, next_node_id]
travel_time = int(model.distance_matrix[depot_id, next_node_id])
departure = max(0, model.demand_dict[next_node_id].start_time - travel_time)
if departure == 0:
crowd_wait_time = travel_time - model.demand_dict[next_node_id].start_time
point_wait_time[next_node_id] = travel_time - model.demand_dict[next_node_id].start_time
timetable.append((departure, departure))
elif 1 <= i <= len(route)-2:
last_node_id = route[i-1]
current_node_id = route[i]
current_node = model.demand_dict[current_node_id]
travel_time = int(model.distance_matrix[last_node_id, current_node_id])
cost_of_distance += model.distance_matrix[last_node_id, current_node_id]
arrival = max(timetable[-1][1]+travel_time, current_node.start_time)
if arrival == timetable[-1][1]+travel_time:
wait_time = timetable[-1][1]+travel_time - current_node.start_time
crowd_wait_time += wait_time
point_wait_time[current_node_id] = wait_time
else:
break
model.crowdcarcost += model.fixcost + model.percost * cost_of_distance
return model.crowdcarcost, crowd_wait_time, point_wait_time
```
这样,`point_wait_time`字典中的每个键值对代表一个点的等待时间,其中键为点的ID,值为该点的等待时间。
相关推荐
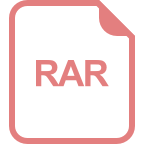
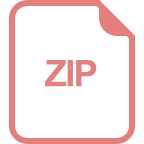















