用theano库求神经网络模型召回率
时间: 2024-03-22 09:17:35 浏览: 59
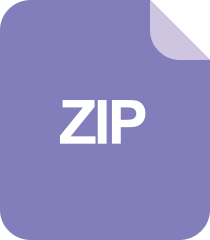
Theano-3D-ConvNet:使用Theano的3D卷积神经网络。 视频的CNN; 时空分类器
首先,需要明确神经网络模型的召回率是指模型正确预测为正例的样本数(True Positive,TP)占所有实际为正例的样本数(True Positive + False Negative,TP+FN)的比例。在使用theano库实现神经网络模型时,可以通过以下步骤计算模型的召回率:
1. 定义神经网络模型:首先,需要使用theano库定义一个神经网络模型。可以选择使用现成的模型结构(如LeNet、VGG等),或者自己定义一个模型结构。
2. 编译模型:定义好神经网络模型后,需要使用theano库编译模型,以便可以对输入数据进行前向传播计算。
3. 加载数据集:为了计算召回率,需要准备一个数据集,其中包含训练数据和测试数据。可以使用theano库提供的数据集(如MNIST、CIFAR-10等),或者自己准备数据集。
4. 训练模型:将数据集输入到神经网络模型中,进行训练。在训练过程中,需要使用theano库提供的优化算法(如SGD、Adam等)来更新模型的参数,以使模型的预测结果更加准确。
5. 测试模型:训练完成后,需要使用测试数据集对模型进行测试。在测试过程中,可以计算模型的召回率,以评估模型的性能。具体计算方法如下:
首先,使用模型对测试数据集进行预测,得到预测结果。将预测结果与实际标签进行比较,得到模型的混淆矩阵(confusion matrix),其中包括TP、FP、FN、TN四个数值。
然后,根据混淆矩阵计算模型的召回率,公式为:Recall = TP / (TP + FN)。
下面是一个简单的示例代码,用于计算LeNet模型在MNIST数据集上的召回率:
```
import theano
import theano.tensor as T
import numpy as np
from theano.tensor.nnet import conv2d
from theano.tensor.signal import pool
# 定义LeNet模型
def LeNet():
x = T.tensor4('x')
y = T.ivector('y')
w1 = theano.shared(np.random.randn(6, 1, 5, 5).astype(np.float32), name='w1')
b1 = theano.shared(np.zeros((6,), dtype=np.float32), name='b1')
w2 = theano.shared(np.random.randn(16, 6, 5, 5).astype(np.float32), name='w2')
b2 = theano.shared(np.zeros((16,), dtype=np.float32), name='b2')
w3 = theano.shared(np.random.randn(256, 120).astype(np.float32), name='w3')
b3 = theano.shared(np.zeros((120,), dtype=np.float32), name='b3')
w4 = theano.shared(np.random.randn(120, 84).astype(np.float32), name='w4')
b4 = theano.shared(np.zeros((84,), dtype=np.float32), name='b4')
w5 = theano.shared(np.random.randn(84, 10).astype(np.float32), name='w5')
b5 = theano.shared(np.zeros((10,), dtype=np.float32), name='b5')
conv1 = conv2d(x, w1)
pool1 = pool.pool_2d(conv1, (2, 2), ignore_border=True)
conv2 = conv2d(pool1, w2)
pool2 = pool.pool_2d(conv2, (2, 2), ignore_border=True)
flatten = T.flatten(pool2, outdim=2)
fc1 = T.nnet.relu(T.dot(flatten, w3) + b3)
fc2 = T.nnet.relu(T.dot(fc1, w4) + b4)
fc3 = T.nnet.softmax(T.dot(fc2, w5) + b5)
prediction = T.argmax(fc3, axis=1)
cost = T.nnet.categorical_crossentropy(fc3, y).mean()
params = [w1, b1, w2, b2, w3, b3, w4, b4, w5, b5]
grads = T.grad(cost, params)
updates = [(p, p - 0.1 * g) for p, g in zip(params, grads)]
train_model = theano.function([x, y], cost, updates=updates)
test_model = theano.function([x], prediction)
return train_model, test_model
# 加载MNIST数据集
from keras.datasets import mnist
(X_train, y_train), (X_test, y_test) = mnist.load_data()
X_train = X_train.reshape(-1, 1, 28, 28).astype(np.float32) / 255.0
X_test = X_test.reshape(-1, 1, 28, 28).astype(np.float32) / 255.0
y_train = y_train.astype(np.int32)
y_test = y_test.astype(np.int32)
# 训练模型
train_model, test_model = LeNet()
for i in range(10):
cost = train_model(X_train, y_train)
print('Epoch %d, cost=%.4f' % (i+1, cost))
# 测试模型
y_pred = test_model(X_test)
confusion_matrix = np.zeros((10, 10), dtype=np.int32)
for i in range(y_test.shape[0]):
confusion_matrix[y_test[i], y_pred[i]] += 1
TP = np.diag(confusion_matrix)
FN = np.sum(confusion_matrix, axis=1) - TP
recall = TP / (TP + FN)
print('Recall:', recall)
```
注意,以上代码仅作为示例,实际应用中需要根据具体情况进行修改和优化。
阅读全文
相关推荐
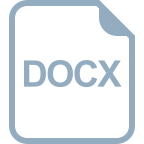
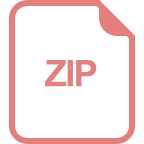

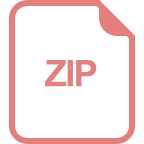
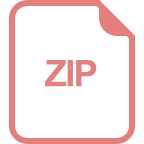
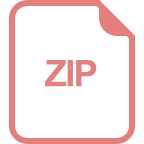
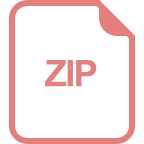
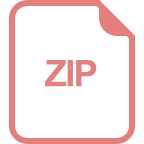
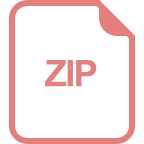
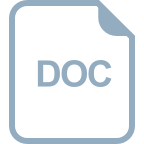
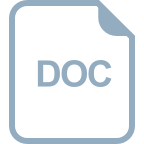
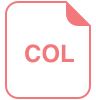
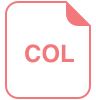
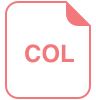
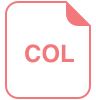
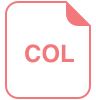
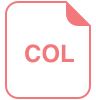
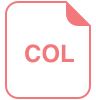