C+-+2048+Game You+are+playing+a+variation+of+game+2048.+Intially+you+have+a+multisetsof+nintegers.
时间: 2024-03-01 10:47:17 浏览: 196
C++ 2048 Game是一种变体的2048游戏。初始时,你有一个包含n个整数的多重集合。
在游戏中,你需要使用C++编程语言来实现一个2048游戏。游戏的规则是将相同数字的方块合并在一起,直到达到2048或无法继续移动为止。
具体的实现方式可以包括以下几个步骤:
1. 创建一个n*n的游戏棋盘,用二维数组表示。
2. 在棋盘上随机生成两个数字方块,初始时这些数字方块的值是从给定的多重集合中选择的。
3. 接受玩家输入的移动指令(上、下、左、右),并根据指令移动数字方块。
4. 如果相邻的两个数字方块的值相同,它们将合并成一个新的数字方块,并更新分数。
5. 每次移动后,在空白位置上随机生成一个新的数字方块(2或4)。
6. 当棋盘上出现2048时,游戏胜利;当无法继续移动时,游戏结束。
你可以使用C++语言中的类和函数来实现游戏逻辑,例如使用类来表示数字方块和棋盘,使用函数来处理移动和合并操作。
相关问题
PCX1 = 1.5482 $Shape factor Cfx for longitudinal force PDX1 = 1.1632 $Longitudinal friction Mux at Fznom PDX2 = -0.11154 $Variation of friction Mux with load PDX3 = 0.94173 $Variation of friction Mux with camber squared PEX1 = 0.27 $Longitudinal curvature Efx at Fznom PEX2 = 0.011693 $Variation of curvature Efx with load PEX3 = 0.053303 $Variation of curvature Efx with load squared PEX4 = 0.59223 $Factor in curvature Efx while driving PKX1 = 32.9102 $Longitudinal slip stiffness Kfx/Fz at Fznom PKX2 = 12.7911 $Variation of slip stiffness Kfx/Fz with load PKX3 = -0.11254 $Exponent in slip stiffness Kfx/Fz with load PHX1 = -0.0017527 $Horizontal shift Shx at Fznom PHX2 = 0.00068824 $Variation of shift Shx with load PVX1 = 0.068079 $Vertical shift Svx/Fz at Fznom PVX2 = 0.0023429 $Variation of shift Svx/Fz with load PPX1 = -0.8733 $linear influence of inflation pressure on longitudinal slip stiffness PPX2 = 0.7035 $quadratic influence of inflation pressure on longitudinal slip stiffness PPX3 = -0.0080216 $linear influence of inflation pressure on peak longitudinal friction PPX4 = -0.47776 $quadratic influence of inflation pressure on peak longitudinal friction RBX1 = 18.3369 $Slope factor for combined slip Fx reduction RBX2 = 18.2559 $Variation of slope Fx reduction with kappa RBX3 = 607.8133 $Influence of camber on stiffness for Fx combined RCX1 = 0.96372 $Shape factor for combined slip Fx reduction REX1 = -1.2699 $Curvature factor of combined Fx REX2 = -0.14323 $Curvature factor of combined Fx with load RHX1 = 0.0037359 $Shift factor for combined slip Fx reduction帮我做成表格
| 参数 | 值 |
| --- | --- |
| PCX1 | 1.5482 |
| PDX1 | 1.1632 |
| PDX2 | -0.11154 |
| PDX3 | 0.94173 |
| PEX1 | 0.27 |
| PEX2 | 0.011693 |
| PEX3 | 0.053303 |
| PEX4 | 0.59223 |
| PKX1 | 32.9102 |
| PKX2 | 12.7911 |
| PKX3 | -0.11254 |
| PHX1 | -0.0017527 |
| PHX2 | 0.00068824 |
| PVX1 | 0.068079 |
| PVX2 | 0.0023429 |
| PPX1 | -0.8733 |
| PPX2 | 0.7035 |
| PPX3 | -0.0080216 |
| PPX4 | -0.47776 |
| RBX1 | 18.3369 |
| RBX2 | 18.2559 |
| RBX3 | 607.8133 |
| RCX1 | 0.96372 |
| REX1 | -1.2699 |
| REX2 | -0.14323 |
| RHX1 | 0.0037359 |
阅读全文
相关推荐
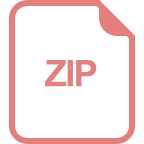
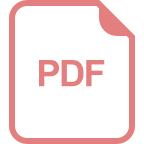
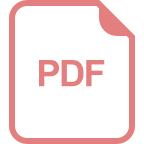
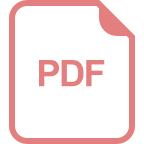
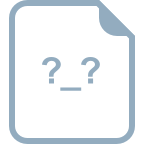
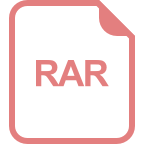
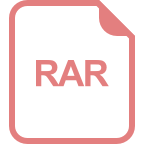
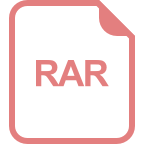
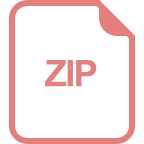
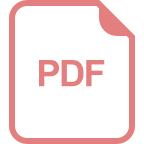
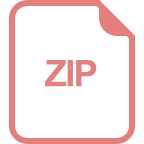
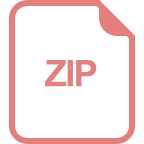
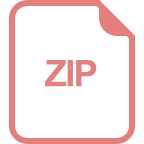
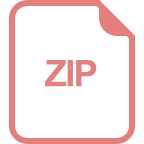