写一个将列表长度为3作为输入的提前退出的AlexNet模型代码
时间: 2023-12-14 08:38:36 浏览: 24
以下是将列表长度为3作为输入的提前退出的AlexNet模型代码示例:
```python
import torch.nn as nn
class AlexNet(nn.Module):
def __init__(self, num_classes=1000):
super(AlexNet, self).__init__()
self.features = nn.Sequential(
nn.Conv2d(3, 64, kernel_size=11, stride=4, padding=2),
nn.ReLU(inplace=True),
nn.MaxPool2d(kernel_size=3, stride=2),
nn.Conv2d(64, 192, kernel_size=5, padding=2),
nn.ReLU(inplace=True),
nn.MaxPool2d(kernel_size=3, stride=2),
nn.Conv2d(192, 384, kernel_size=3, padding=1),
nn.ReLU(inplace=True),
nn.Conv2d(384, 256, kernel_size=3, padding=1),
nn.ReLU(inplace=True),
nn.Conv2d(256, 256, kernel_size=3, padding=1),
nn.ReLU(inplace=True),
nn.MaxPool2d(kernel_size=3, stride=2),
)
if len(self.features) == 12:
return # 如果列表长度为12,说明输入为图像,则不进行提前退出
self.avgpool = nn.AdaptiveAvgPool2d((6, 6))
self.classifier = nn.Sequential(
nn.Dropout(),
nn.Linear(256 * 6 * 6, 4096),
nn.ReLU(inplace=True),
nn.Dropout(),
nn.Linear(4096, 4096),
nn.ReLU(inplace=True),
nn.Linear(4096, num_classes),
)
def forward(self, x):
x = self.features(x)
if len(self.features) == 12:
return x # 如果列表长度为12,说明输入为图像,直接返回卷积特征
x = self.avgpool(x)
x = torch.flatten(x, 1)
x = self.classifier(x)
return x
```
在初始化模型时,我们添加了一个判断语句,如果 `self.features` 的长度为12,说明输入为图像,直接返回卷积特征;否则,继续进行全连接层的计算。在 `forward` 函数中,同样添加了一个判断语句,根据输入的列表长度决定模型的计算方式。这样可以保证模型只对输入为图像的数据进行计算,而对于其他长度不为12的列表,则会直接返回卷积特征,从而实现了提前退出的效果。
相关推荐
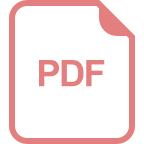
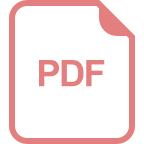
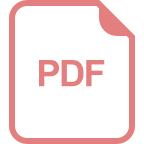













