torch.profiler
时间: 2023-10-12 18:07:47 浏览: 231
torch.profiler 是 PyTorch 提供的一个性能分析工具,用于分析 PyTorch 模型的运行时性能。它可以帮助开发者找出模型中的瓶颈,并优化模型的性能。
使用 torch.profiler,你可以记录模型的运行时间、内存占用情况以及函数调用堆栈等信息。这些信息可以帮助你分析模型中每个操作的耗时和资源占用情况,从而找出性能瓶颈。
下面是一个使用 torch.profiler 的简单示例:
```python
import torch
from torchvision.models import resnet50
# 创建一个 ResNet50 模型
model = resnet50()
# 定义输入数据
input_data = torch.randn(1, 3, 224, 224)
# 创建一个 profiler 对象,并指定要分析的模块
profiler = torch.profiler.profile(
activities=[torch.profiler.ProfilerActivity.CPU, torch.profiler.ProfilerActivity.CUDA], schedule=torch.profiler.schedule(wait=2, warmup=1),
on_trace_ready=torch.profiler.tensorboard_trace_handler('./log'),
)
# 启动 profiler
with profiler:
output = model(input_data)
# 分析结果将保存到 './log' 目录下
```
以上示例中,我们创建了一个 ResNet50 模型,并使用 torch.profiler 来对模型的运行进行性能分析。可以通过调整 profiler 的参数来获取不同层次的细粒度分析结果,如 CPU、CUDA 的运行时间、内存占用情况等。同时,可以通过指定不同的输出路径和格式,将分析结果保存到不同的文件中,以供后续查看和分析。
需要注意的是,使用 torch.profiler 可能会对模型的运行速度产生一定的影响,所以在进行性能分析时应尽量避免对模型进行过多的修改和调整。另外,为了获取更准确的分析结果,建议在模型运行之前进行适当的预热和等待时间设置。
阅读全文
相关推荐
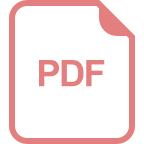
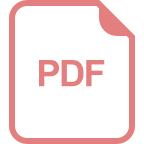
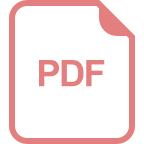















