卷积神经网络的面部表情识别全部代码
时间: 2023-11-01 21:07:05 浏览: 67
这里提供一个使用卷积神经网络进行面部表情识别的示例代码,基于Python和Keras框架实现。
首先,需要安装Keras和相关依赖库。可以通过以下命令安装:
```
pip install keras numpy pandas matplotlib tensorflow
```
接下来,我们来看一下完整的代码:
```python
import numpy as np
import pandas as pd
import matplotlib.pyplot as plt
from sklearn.model_selection import train_test_split
from keras.utils import np_utils
from keras.models import Sequential
from keras.layers.core import Dense, Dropout, Activation, Flatten
from keras.layers.convolutional import Conv2D, MaxPooling2D
# 加载数据集
data = pd.read_csv("fer2013.csv")
# 将像素列转换为像素数组
pixels = data['pixels'].tolist()
images = []
for pixel_sequence in pixels:
single_image = [int(pixel) for pixel in pixel_sequence.split(' ')]
single_image = np.asarray(single_image).reshape(48, 48)
images.append(single_image.astype('float32'))
# 将标签列转换为独热编码
labels = pd.get_dummies(data['emotion']).values
# 将数据集拆分为训练集、验证集和测试集
train_images, test_images, train_labels, test_labels = train_test_split(images, labels, test_size=0.1, random_state=42)
train_images, val_images, train_labels, val_labels = train_test_split(train_images, train_labels, test_size=0.1, random_state=41)
# 将图像数据转换为4D张量形式
train_images = np.array(train_images).reshape(-1, 48, 48, 1)
val_images = np.array(val_images).reshape(-1, 48, 48, 1)
test_images = np.array(test_images).reshape(-1, 48, 48, 1)
# 归一化图像数据
train_images /= 255
val_images /= 255
test_images /= 255
# 将标签数据转换为独热编码
train_labels = np_utils.to_categorical(train_labels, 7)
val_labels = np_utils.to_categorical(val_labels, 7)
test_labels = np_utils.to_categorical(test_labels, 7)
# 构建卷积神经网络模型
model = Sequential()
model.add(Conv2D(32, (3, 3), padding='same', activation='relu', input_shape=(48, 48, 1)))
model.add(Conv2D(64, (3, 3), padding='same', activation='relu'))
model.add(MaxPooling2D(pool_size=(2, 2)))
model.add(Dropout(0.25))
model.add(Conv2D(128, (3, 3), padding='same', activation='relu'))
model.add(MaxPooling2D(pool_size=(2, 2)))
model.add(Conv2D(256, (3, 3), padding='same', activation='relu'))
model.add(MaxPooling2D(pool_size=(2, 2)))
model.add(Dropout(0.25))
model.add(Flatten())
model.add(Dense(256, activation='relu'))
model.add(Dropout(0.5))
model.add(Dense(7, activation='softmax'))
# 编译模型
model.compile(loss='categorical_crossentropy', optimizer='adam', metrics=['accuracy'])
# 训练模型
history = model.fit(train_images, train_labels, batch_size=64, epochs=50, verbose=1, validation_data=(val_images, val_labels))
# 评估模型
score = model.evaluate(test_images, test_labels, verbose=0)
print('Test loss:', score[0])
print('Test accuracy:', score[1])
# 绘制训练和验证的损失和准确率曲线
plt.figure(figsize=[6, 4])
plt.plot(history.history['loss'], 'black', linewidth=1.0)
plt.plot(history.history['val_loss'], 'green', linewidth=1.0)
plt.legend(['Training Loss', 'Validation Loss'], fontsize=10)
plt.xlabel('Epochs', fontsize=10)
plt.ylabel('Loss', fontsize=10)
plt.title('Loss Curves', fontsize=12)
plt.figure(figsize=[6, 4])
plt.plot(history.history['accuracy'], 'black', linewidth=1.0)
plt.plot(history.history['val_accuracy'], 'green', linewidth=1.0)
plt.legend(['Training Accuracy', 'Validation Accuracy'], fontsize=10)
plt.xlabel('Epochs', fontsize=10)
plt.ylabel('Accuracy', fontsize=10)
plt.title('Accuracy Curves', fontsize=12)
plt.show()
```
代码中首先加载FER2013数据集,然后将像素列转换为像素数组,将标签列转换为独热编码,并将数据集拆分为训练集、验证集和测试集。接下来将图像数据转换为4D张量形式,并归一化图像数据。最后构建卷积神经网络模型,并使用训练集和验证集来训练模型。最后评估模型在测试集上的表现,并绘制训练和验证的损失和准确率曲线。
相关推荐
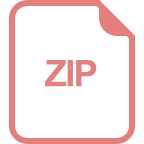
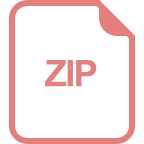
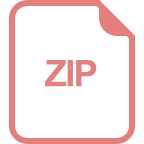











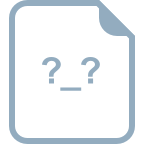
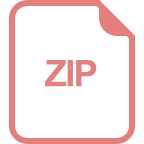
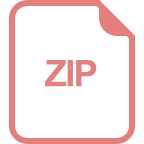
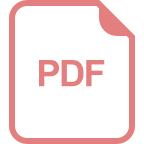