python手写数字识别体
时间: 2024-06-11 08:03:37 浏览: 22
Python手写数字识别通常指的是使用机器学习和深度学习技术,如卷积神经网络(Convolutional Neural Networks, CNN)在Python中实现的MNIST数据集上的手写数字识别任务。MNIST是一个经典的数据集,包含60,000个训练样本和10,000个测试样本,每个样本都是28x28像素的手写数字图片。
以下是手写数字识别的一个简单概述:
1. 数据预处理:首先,加载MNIST数据并将其转换为模型可以接受的格式,例如灰度图像和归一化的数值。
2. 模型架构:构建CNN,它包括卷积层、池化层和全连接层。卷积层用于特征提取,池化层用于降低数据维度,全连接层用于分类。
3. 训练过程:使用训练数据集对模型进行训练,优化算法如随机梯度下降(SGD)或Adam等更新权重,目标是减小损失函数(如交叉熵损失)。
4. 评估与验证:使用验证集检查模型性能,避免过拟合,并调整超参数以优化模型。
5. 测试:最后,用测试集对模型进行最终性能评估,计算准确率和其他评估指标。
相关问题
python手写数字体识别
Python手写数字体识别通常指的是使用机器学习和深度学习技术,特别是像卷积神经网络(Convolutional Neural Networks, CNN)来识别图像中的手写字迹。这在计算机视觉领域是一个经典的应用场景,比如MNIST数据集就是常被用于训练此类模型的标准数据集,它包含了大量的灰度图像,每张图像代表一个28x28像素的手写数字。
在这个过程中,首先你需要对输入的手写图像进行预处理,例如归一化、二值化等步骤,然后构建一个CNN模型来提取特征并进行分类。训练时,模型会学习到不同数字的笔画模式,测试阶段则用来预测新图片中的数字。
具体实现步骤可能包括:
1. **数据加载与预处理**:加载MNIST或其他类似的库获取数据,对图片进行缩放、归一化,并将图像转化为模型能接受的格式。
2. **构建CNN模型**:设计卷积层、池化层和全连接层的组合,这些层能够捕获图像的空间特征。
3. **模型编译**:选择优化器(如Adam)、损失函数(如交叉熵)和评估指标(如准确率)。
4. **训练模型**:使用训练数据对模型进行训练,并通过验证集监控模型性能。
5. **模型评估与预测**:在测试集上评估模型的性能,并用模型对新的手写数字图片进行识别。
Python手写数字识别
Python手写数字识别是指使用Python编程语言实现的一种数字识别算法,它可以对手写数字进行自动识别。常用的手写数字识别算法包括K近邻算法、支持向量机(SVM)算法、神经网络算法等。其中,神经网络算法是目前最为流行和有效的手写数字识别算法之一。下面是一个简单的基于神经网络的Python手写数字识别示例,使用Keras库来构建神经网络模型,使用MNIST数据集进行训练和测试。
```python
import numpy as np
from keras.datasets import mnist
from keras.models import Sequential
from keras.layers import Dense, Dropout, Flatten
from keras.layers import Conv2D, MaxPooling2D
from keras.utils import np_utils
# 加载MNIST数据集
(X_train, y_train), (X_test, y_test) = mnist.load_data()
# 数据预处理
X_train = X_train.reshape(X_train.shape[0], 28, 28, 1)
X_test = X_test.reshape(X_test.shape[0], 28, 28, 1)
X_train = X_train.astype('float32')
X_test = X_test.astype('float32')
X_train /= 255
X_test /= 255
y_train = np_utils.to_categorical(y_train, 10)
y_test = np_utils.to_categorical(y_test, 10)
# 构建神经网络模型
model = Sequential()
model.add(Conv2D(32, kernel_size=(3, 3), activation='relu', input_shape=(28, 28, 1)))
model.add(Conv2D(64, (3, 3), activation='relu'))
model.add(MaxPooling2D(pool_size=(2, 2)))
model.add(Dropout(0.25))
model.add(Flatten())
model.add(Dense(128, activation='relu'))
model.add(Dropout(0.5))
model.add(Dense(10, activation='softmax'))
# 编译模型
model.compile(loss='categorical_crossentropy', optimizer='adam', metrics=['accuracy'])
# 训练模型
model.fit(X_train, y_train, batch_size=128, epochs=10, verbose=1, validation_data=(X_test, y_test))
# 测试模型
score = model.evaluate(X_test, y_test, verbose=0)
print('Test loss:', score[0])
print('Test accuracy:', score[1])
# 预测手写数字
img = cv2.imread('test.jpg', 0)
img = cv2.resize(img, (28, 28))
img = img.reshape(1, 28, 28, 1)
img = img.astype('float32')
img /= 255
result = model.predict_classes(img)
print(result)
```
相关推荐
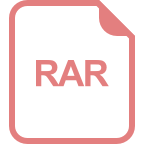
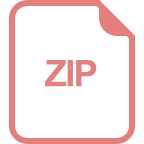
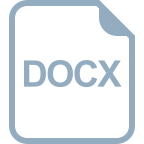












