怎么得到差异基因表达的数据,R语言
时间: 2023-07-10 11:26:54 浏览: 175
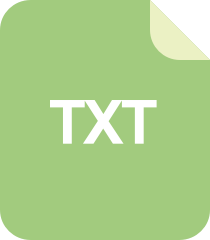
差异基因分析R代码
要进行差异基因表达分析,首先需要获得基因表达谱数据。基因表达谱数据可以从公共数据库中下载,例如GEO、TCGA、ENCODE等,也可以通过RNA测序实验获得。如果您已经获得了基因表达谱数据,可以使用以下代码将其导入到R中:
```R
# 导入表达谱数据
data <- read.table("expression_data.txt", header=TRUE, row.names=1)
```
其中,"expression_data.txt"为基因表达谱数据的文件名,文件格式为txt格式,第一行为列名,第一列为基因名。
如果您使用的是RNA测序数据,可以使用以下代码将其转化为基因表达谱数据:
```R
# 导入RNA测序数据
library(Rsubread)
counts <- featureCounts(files=list("sample1.bam", "sample2.bam"), annot.ext="gene.gtf", isGTFAnnotationFile=TRUE)
data <- counts$counts
rownames(data) <- counts$annotation$gene_id
```
其中,"sample1.bam"和"sample2.bam"为RNA测序数据的文件名,"gene.gtf"为参考基因组注释文件。使用featureCounts()函数从RNA测序数据中提取基因计数,然后将其转化为基因表达谱数据并导入到R中。
导入数据后,就可以进行后续的差异基因表达分析了。
阅读全文
相关推荐



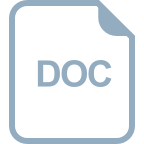
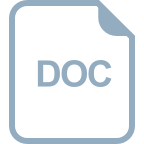
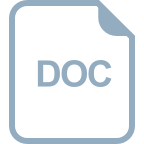









