list怎么ignore_index=True
时间: 2023-12-29 10:52:52 浏览: 158
在Python中,我们可以使用`ignore_index=True`参数来忽略索引,该参数适用于许多与列表相关的函数和方法,例如`concat()`函数和`append()`方法。这个参数可以在许多情况下很有用,特别是在合并数据集或添加新行时需要忽略索引。下面是一个简单的例子,展示了如何使用`ignore_index=True`参数来合并两个包含不同索引的列表:
```python
import pandas as pd
# 创建两个列表
list1 = ['a', 'b', 'c']
list2 = ['d', 'e', 'f']
# 将两个列表合并
merged_list = pd.concat([pd.Series(list1), pd.Series(list2)], ignore_index=True)
print(merged_list)
```
输出结果为:
```
0 a
1 b
2 c
3 d
4 e
5 f
dtype: object
```
可以看到,合并后的列表中的索引已被重新设置为连续的整数。
相关问题
all_etf_data = pd.concat(df_list, ignore_index=True)
all_etf_data 是一个数据帧,它由多个数据帧组成,这些数据帧来自 df_list 列表。pd.concat() 函数用于将多个数据帧合并成一个数据帧。ignore_index 参数用于忽略原来每个数据帧的索引,并在合并后使用一个新的索引。
例如:
```
df1 = pd.DataFrame({'A': ['A0', 'A1', 'A2'],
'B': ['B0', 'B1', 'B2'],
'C': ['C0', 'C1', 'C2']},
index=[0, 1, 2])
df2 = pd.DataFrame({'A': ['A3', 'A4', 'A5'],
'B': ['B3', 'B4', 'B5'],
'C': ['C3', 'C4', 'C5']},
index=[3, 4, 5])
df3 = pd.DataFrame({'A': ['A6', 'A7', 'A8'],
'B': ['B6', 'B7', 'B8'],
'C': ['C6', 'C7', 'C8']},
index=[6, 7, 8])
df_list = [df1, df2, df3]
all_df = pd.concat(df_list, ignore_index=True)
print(all_df)
```
输出结果:
```
A B C
0 A0 B0 C0
1 A1 B1 C1
2 A2 B2 C2
3 A3 B3 C3
4 A4 B4 C4
5 A5 B5 C5
6 A6 B6 C6
7 A7 B7 C7
8 A8 B8 C8
```
这样就将三个数据帧合并成了一个数据帧,并且使用了新的索引。
优化代码 def cluster_format(self, start_time, end_time, save_on=True, data_clean=False, data_name=None): """ local format function is to format data from beihang. :param start_time: :param end_time: :return: """ # 户用簇级数据清洗 if data_clean: unused_index_col = [i for i in self.df.columns if 'Unnamed' in i] self.df.drop(columns=unused_index_col, inplace=True) self.df.drop_duplicates(inplace=True, ignore_index=True) self.df.reset_index(drop=True, inplace=True) dupli_header_lines = np.where(self.df['sendtime'] == 'sendtime')[0] self.df.drop(index=dupli_header_lines, inplace=True) self.df = self.df.apply(pd.to_numeric, errors='ignore') self.df['sendtime'] = pd.to_datetime(self.df['sendtime']) self.df.sort_values(by='sendtime', inplace=True, ignore_index=True) self.df.to_csv(data_name, index=False) # 调用基本格式化处理 self.df = super().format(start_time, end_time) module_number_register = np.unique(self.df['bat_module_num']) # if registered m_num is 0 and not changed, there is no module data if not np.any(module_number_register): logger.logger.warning("No module data!") sys.exit() if 'bat_module_voltage_00' in self.df.columns: volt_ref = 'bat_module_voltage_00' elif 'bat_module_voltage_01' in self.df.columns: volt_ref = 'bat_module_voltage_01' elif 'bat_module_voltage_02' in self.df.columns: volt_ref = 'bat_module_voltage_02' else: logger.logger.warning("No module data!") sys.exit() self.df.dropna(axis=0, subset=[volt_ref], inplace=True) self.df.reset_index(drop=True, inplace=True) self.headers = list(self.df.columns) # time duration of a cluster self.length = len(self.df) if self.length == 0: logger.logger.warning("After cluster data clean, no effective data!") raise ValueError("No effective data after cluster data clean.") self.cluster_stats(save_on) for m in range(self.mod_num): print(self.clusterid, self.mod_num) self.module_list.append(np.unique(self.df[f'bat_module_sn_{str(m).zfill(2)}'].dropna())[0])
Here are some possible optimizations for the given code:
1. Instead of using a list comprehension to find columns with 'Unnamed' in their names, you can use the `filter()` function along with a lambda function to achieve the same result in a more concise way:
```
unused_index_col = list(filter(lambda x: 'Unnamed' in x, self.df.columns))
```
2. Instead of dropping duplicates and resetting the index separately, you can use the `drop_duplicates()` function with the `ignore_index` parameter set to `True` to achieve both in one step:
```
self.df.drop_duplicates(inplace=True, ignore_index=True)
```
3. Instead of using `sys.exit()` to terminate the program when there is no module data, you can raise a `ValueError` with an appropriate error message:
```
raise ValueError("No module data!")
```
4. Instead of using a series of `if` statements to find the voltage reference column, you can use the `loc` accessor with a boolean mask to select the first column that starts with 'bat_module_voltage':
```
volt_ref_col = self.df.columns[self.df.columns.str.startswith('bat_module_voltage')][0]
```
5. Instead of using a loop to append a single item to a list, you can use the `append()` method directly:
```
self.module_list.append(np.unique(self.df[f'bat_module_sn_{str(m).zfill(2)}'].dropna())[0])
```
By applying these optimizations, the code can become more concise and efficient.
阅读全文
相关推荐
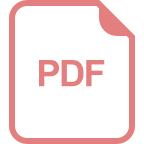
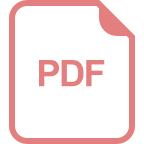
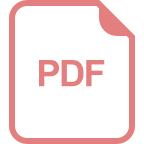












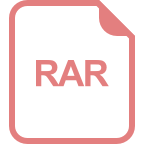
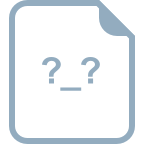