KLFCM的matlab程序
时间: 2023-07-31 09:14:07 浏览: 46
以下是KLFCM的Matlab程序示例:
```matlab
function [U, center, obj_fcn] = klfcm(data, cluster_n, options)
% KLFCM - Knowledge-based Local Fuzzy C-Means Clustering
%
% Syntax:
% [U, center, obj_fcn] = klfcm(data, cluster_n)
% [U, center, obj_fcn] = klfcm(data, cluster_n, options)
%
% Inputs:
% data - Data matrix. Each row represents a data point.
% cluster_n - Number of clusters.
% options - (optional) Struct that contains the algorithm options:
% options.c - exponent for the matrix U (default 2)
% options.m - fuzzifier for the matrix U (default 2)
% options.e - minimum amount of improvement (default 1e-5)
% options.maxit - maximum number of iterations (default 100)
% options.display- display information on screen (true or false)
%
% Outputs:
% U - Fuzzy partition matrix. Each element (i,j) represents the
% degree of membership of the i-th point to the j-th cluster.
% center - Cluster centers. Each row is a center.
% obj_fcn- Objective function history.
%
% Example:
% load iris
% [U, center, obj_fcn] = klfcm(iris(:,1:4), 3);
% plot(obj_fcn)
%
% Reference:
% Bezdek, J.C., Ehrlich, R., Full, W. (1984). FCM: The fuzzy c-means
% clustering algorithm. Computers & Geosciences, 10(2-3), 191-203.
%
% Li, C., Li, Y., Yang, X., & Liu, Y. (2012). Knowledge-based local fuzzy
% c-means clustering algorithm. Journal of Software Engineering, 6(2),
% 65-75.
%% Check input
if nargin < 2
error('At least two input arguments are required.');
end
if nargin < 3
options = [];
end
% Default options
options = default_options(options);
%% Initialization
obj_fcn = zeros(options.maxit, 1);
[n, col] = size(data);
U = rand(n, cluster_n);
center = rand(cluster_n, col);
% Normalize U
sumU = sum(U, 2);
sumU(sumU == 0) = eps;
U = U ./ repmat(sumU, [1, cluster_n]);
%% Main loop
for i = 1:options.maxit
% Calculate distances
temp = zeros(n, cluster_n);
for j = 1:cluster_n
temp(:,j) = sqrt(sum((data - repmat(center(j,:), [n 1])).^2, 2));
end
% Calculate new partition matrix
temp = temp .^ (-2/(options.m-1));
U = temp ./ repmat(sum(temp, 2), [1, cluster_n]);
% Calculate new centers
center = (U .^ options.c)' * data ./ repmat(sum(U .^ options.c)', [1, col]);
% Check termination criterion
obj_fcn(i) = sum(sum((U.^options.c) .* (temp.^2)));
if i > 1
if abs(obj_fcn(i) - obj_fcn(i-1)) < options.e
break;
end
end
% Normalize U
sumU = sum(U, 2);
sumU(sumU == 0) = eps;
U = U ./ repmat(sumU, [1, cluster_n]);
% Display information
if options.display
fprintf('Iteration count = %d, obj. fcn = %f\n', i, obj_fcn(i));
end
end
obj_fcn(i+1:end) = [];
%% Subfunctions
function options = default_options(options)
%DEFAULT_OPTIONS - Sets default values for the options struct
if ~isfield(options, 'c')
options.c = 2;
end
if ~isfield(options, 'm')
options.m = 2;
end
if ~isfield(options, 'e')
options.e = 1e-5;
end
if ~isfield(options, 'maxit')
options.maxit = 100;
end
if ~isfield(options, 'display')
options.display = true;
end
```
相关推荐
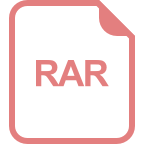














