def build_overall_sequence(sequences): unique_sequence = [] matrix = [sequences[::-1, :].diagonal(i) for i in range(-sequences.shape[0] + 1, sequences.shape[1])] for i in range(len(matrix)): unique_sequence.append(np.median(matrix[i])) unique_sequence = np.array(unique_sequence) return unique_sequence
时间: 2024-04-05 08:33:14 浏览: 101
这是一个函数,其输入为一个矩阵sequences。该函数的作用是将矩阵sequences的所有对角线提取出来,并计算它们的中位数,最终得到一个矩阵unique_sequence。
具体来说,该函数首先创建一个空列表unique_sequence,然后使用numpy中的diagonal函数将sequences的所有对角线提取出来,并存储在一个列表matrix中。接下来,对于matrix中的每个元素,使用numpy中的median函数计算其中位数,并将其添加到unique_sequence中。最后,将unique_sequence转换为numpy数组并返回。
相关问题
def build_sequences(text, window_size): #text:list of capacity x, y = [],[] for i in range(len(text) - window_size): sequence = text[i:i+window_size] target = text[i+1:i+1+window_size] x.append(sequence) y.append(target) return np.array(x), np.array(y) # 留一评估:一组数据为测试集,其他所有数据全部拿来训练 def get_train_test(data_dict, name, window_size=8): data_sequence=data_dict[name][1] train_data, test_data = data_sequence[:window_size+1], data_sequence[window_size+1:] train_x, train_y = build_sequences(text=train_data, window_size=window_size) for k, v in data_dict.items(): if k != name: data_x, data_y = build_sequences(text=v[1], window_size=window_size) train_x, train_y = np.r_[train_x, data_x], np.r_[train_y, data_y] return train_x, train_y, list(train_data), list(test_data) def relative_error(y_test, y_predict, threshold): true_re, pred_re = len(y_test), 0 for i in range(len(y_test)-1): if y_test[i] <= threshold >= y_test[i+1]: true_re = i - 1 break for i in range(len(y_predict)-1): if y_predict[i] <= threshold: pred_re = i - 1 break return abs(true_re - pred_re)/true_re def evaluation(y_test, y_predict): mae = mean_absolute_error(y_test, y_predict) mse = mean_squared_error(y_test, y_predict) rmse = sqrt(mean_squared_error(y_test, y_predict)) return mae, rmse def setup_seed(seed): np.random.seed(seed) # Numpy module. random.seed(seed) # Python random module. os.environ['PYTHONHASHSEED'] = str(seed) # 为了禁止hash随机化,使得实验可复现。 torch.manual_seed(seed) # 为CPU设置随机种子 if torch.cuda.is_available(): torch.cuda.manual_seed(seed) # 为当前GPU设置随机种子 torch.cuda.manual_seed_all(seed) # if you are using multi-GPU,为所有GPU设置随机种子 torch.backends.cudnn.benchmark = False torch.backends.cudnn.deterministic = True
这段代码主要是用来进行数据预处理和模型评估的。其中,`build_sequences`函数用来将数据转化为序列数据,`get_train_test`函数用来获取训练集和测试集,`relative_error`函数用来计算相对误差,`evaluation`函数用来计算模型的MAE、MSE和RMSE指标。另外,`setup_seed`函数用来设置随机种子,以保证实验的可复现性。整个代码涉及到了numpy、random、os和torch等库的使用,可以用来进行深度学习相关的实验和研究。
def get_occurrences_of_sequence(original_dataset: [], checked_sequence: ()) -> []:
# function definition
def get_occurrences_of_sequence(original_dataset: [], checked_sequence: ()) -> []:
"""
This function takes in two parameters:
1. original_dataset: a list of tuples containing sequences of integers
2. checked_sequence: a tuple containing sequence of integers to be searched in the original_dataset
The function returns a list of tuples containing the index of the sequence in the original_dataset and the number of times the checked_sequence occurs in that sequence.
If the checked_sequence is not found in any of the sequences in the original_dataset, the function returns an empty list.
"""
occurrences_list = []
for i, sequence in enumerate(original_dataset):
# check if checked_sequence is a sub-sequence of sequence
if checked_sequence in [sequence[j:j+len(checked_sequence)] for j in range(len(sequence)-len(checked_sequence)+1)]:
# count the number of times checked_sequence occurs in sequence
count = sequence.count(checked_sequence)
# store the index of the sequence and the count of checked_sequence in a tuple
occurrences_list.append((i, count))
return occurrences_list
阅读全文
相关推荐











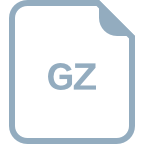
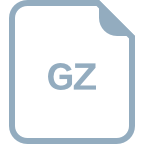


