精简下面表达:Existing protein function prediction methods integrate PPI networks and multivariate bioinformatics data to improve the performance of function prediction. By combining multivariate information, the interactions between proteins become diverse. Different interactions’ functions in functional prediction are various. Combining multiple interactions simply between two proteins can effectively reduce the effect of false negatives and increase the number of predicted functions, but it can also increase the number of false positive functions, which contribute to nonobvious enhancement for the overall functional prediction performance. In this article, we have presented a framework for protein function prediction algorithms based on PPI network and semantic similarity with the addition of protein hierarchical functions to them. The framework relies on diverse clustering algorithms and the calculation of protein semantic similarity for protein function prediction. Classification and similarity calculations for protein pairs clustered by the functional feature are more accurate and reliable, allowing for the prediction of protein function at different functional levels from different proteomes, and giving biological applications greater flexibility.The method proposed in this paper performs well on protein data from wine yeast cells, but how well it matches other data remains to be verified. Yet until now, most unknown proteins have only been able to predict protein function by calculating similarities to their homologues. The predictions result of those unknown proteins without homologues are unstable because they are relatively isolated in the protein interaction network. It is difficult to find one protein with high similarity. In the framework proposed in this article, the number of features selected after clustering and the number of protein features selected for each functional layer has a significant impact on the accuracy of subsequent functional predictions. Therefore, when making feature selection, it is necessary to select as many functional features as possible that are important for the whole interaction network. When an incorrect feature was selected, the prediction results will be somewhat different from the actual function. Thus as a whole, the method proposed in this article has improved the accuracy of protein function prediction based on the PPI network method to a certain extent and reduces the probability of false positive prediction results.
时间: 2023-03-19 07:23:13 浏览: 223
本文提出了一种基于PPI网络和语义相似性,加上蛋白质分层功能的蛋白质功能预测算法框架,对酒葡萄酵母细胞的蛋白质数据表现出良好的效果,但其他数据的效果如何仍有待验证。此外,该框架中的功能特征选择的数量以及每个功能层中蛋白质特征的选择量,对于后续功能预测的准确度有很大影响。因此,在进行特征选择时,必须尽可能选择对整个交互网络重要的多种功能特征,从而提高蛋白质功能预测的准确性,减少错误预测的概率。
阅读全文
相关推荐






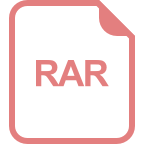
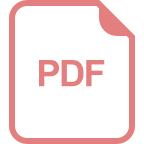

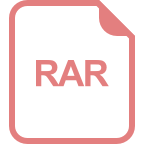
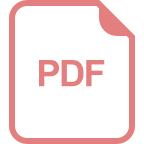
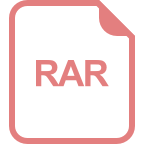
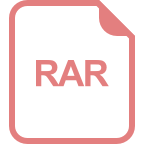
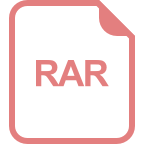

