使用双向LSTM将文本转为向量并进行预测的代码
时间: 2023-02-13 20:56:44 浏览: 148
使用双向 LSTM 将文本转换为向量并进行预测的代码如下:
```python
from tensorflow.keras.layers import LSTM, Embedding, Dense
from tensorflow.keras.models import Sequential
# 定义模型
model = Sequential()
# 嵌入层,将单词转换为向量
model.add(Embedding(input_dim=vocab_size, output_dim=embedding_dim))
# 双向 LSTM 层
model.add(Bidirectional(LSTM(units=hidden_dim)))
# 输出层,使用 sigmoid 激活函数
model.add(Dense(1, activation='sigmoid'))
# 编译模型
model.compile(loss='binary_crossentropy', optimizer='adam', metrics=['accuracy'])
# 训练模型
model.fit(X_train, y_train, batch_size=batch_size, epochs=num_epochs)
# 预测结果
predictions = model.predict(X_test)
```
在这段代码中,我们定义了一个使用 Embedding 层和双向 LSTM 层的模型,并使用 sigmoid 激活函数在输出层进行二元分类。然后我们使用 binary_crossentropy 损失函数和 Adam 优化器来编译模型,并使用训练数据训练模型。最后,我们使用测试数据对模型进行预测。
注意:在这段代码中,我们假设已经定义了训练数据 `X_train` 和 `y_train`,测试数据 `X_test`,以及模型所需的其他参数,如词汇表大小 `vocab_size`、嵌入维度 `embedding_dim`、隐藏层单元数 `hidden_dim`、批大
阅读全文
相关推荐
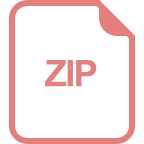

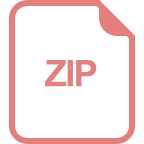
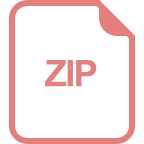
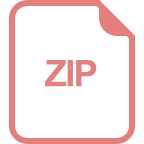
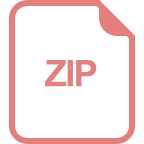
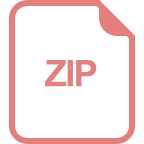
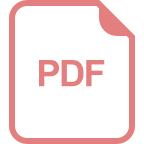
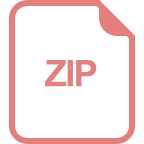
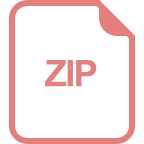
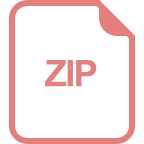
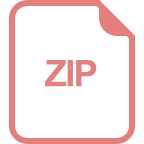
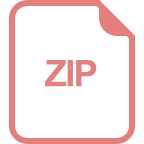
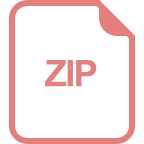
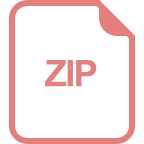
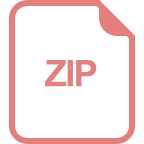