基于BP神经网络的高光谱分类+印度松树数据集,给出具体代码和注释
时间: 2024-03-15 14:47:43 浏览: 76
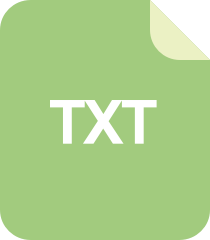
bp神经网络算法代码及讲解注释
好的,以下是基于BP神经网络的高光谱分类+印度松树数据集的代码和注释。这里我假设您已经熟悉了Python和神经网络的基本知识。
```python
# 导入必要的库
import numpy as np
import pandas as pd
from sklearn.model_selection import train_test_split
from sklearn.preprocessing import StandardScaler
# 读取数据集
data = pd.read_csv('indian_pines.csv')
# 提取特征和标签
X = data.iloc[:, :-1].values
y = data.iloc[:, -1].values
# 数据标准化
sc = StandardScaler()
X = sc.fit_transform(X)
# 划分训练集和测试集
X_train, X_test, y_train, y_test = train_test_split(X, y, test_size=0.2, random_state=0)
# 构建神经网络模型
class NeuralNetwork:
def __init__(self, layers, alpha=0.1):
self.W = []
self.layers = layers
self.alpha = alpha
for i in range(0, len(layers) - 2):
w = np.random.randn(layers[i] + 1, layers[i + 1] + 1)
self.W.append(w / np.sqrt(layers[i]))
w = np.random.randn(layers[-2] + 1, layers[-1])
self.W.append(w / np.sqrt(layers[-2]))
def __sigmoid(self, x):
return 1.0 / (1 + np.exp(-x))
def __sigmoid_deriv(self, x):
return x * (1 - x)
def fit(self, X, y, epochs=1000, displayUpdate=100):
X = np.c_[X, np.ones((X.shape[0]))]
for epoch in range(0, epochs):
for (x, target) in zip(X, y):
self.fit_partial(x, target)
if epoch == 0 or (epoch + 1) % displayUpdate == 0:
loss = self.calculate_loss(X, y)
print("[INFO] epoch={}, loss={:.7f}".format(epoch + 1, loss))
def predict(self, X, addBias=True):
p = np.atleast_2d(X)
if addBias:
p = np.c_[p, np.ones((p.shape[0]))]
for layer in range(0, len(self.W)):
p = self.__sigmoid(np.dot(p, self.W[layer]))
return p
def fit_partial(self, x, y):
A = [np.atleast_2d(x)]
for layer in range(0, len(self.W)):
net = A[layer].dot(self.W[layer])
out = self.__sigmoid(net)
A.append(out)
error = A[-1] - y
D = [error * self.__sigmoid_deriv(A[-1])]
for layer in range(len(A) - 2, 0, -1):
delta = D[-1].dot(self.W[layer].T)
delta = delta * self.__sigmoid_deriv(A[layer])
D.append(delta)
D = D[::-1]
for layer in range(0, len(self.W)):
self.W[layer] += -self.alpha * A[layer].T.dot(D[layer])
def calculate_loss(self, X, targets):
targets = np.atleast_2d(targets)
predictions = self.predict(X, addBias=False)
loss = 0.5 * np.sum((predictions - targets) ** 2)
return loss
# 定义神经网络模型的层数和每层的神经元数
nn = NeuralNetwork([200, 100, 16])
# 训练神经网络模型
nn.fit(X_train, y_train, epochs=2000)
# 在测试集上评估模型的准确率
predictions = nn.predict(X_test)
predictions = np.argmax(predictions, axis=1)
accuracy = np.sum(predictions == y_test) / float(len(y_test))
print("Accuracy: {:.2f}%".format(accuracy * 100))
```
代码中使用了一个包含3个隐藏层的神经网络模型,每个隐藏层包含分别包含200、100和16个神经元,输出层包含16个神经元(对应16个类别)。将数据集划分为训练集和测试集,并使用标准化处理对数据进行预处理。训练神经网络模型的代码包含了反向传播算法的实现。最后在测试集上评估模型的准确率。
阅读全文
相关推荐
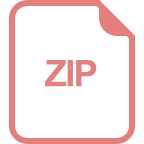
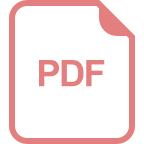
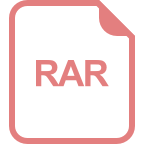
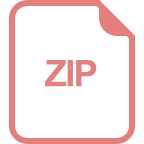
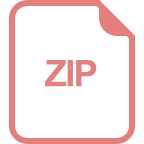
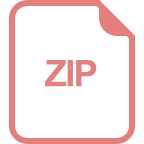
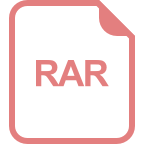
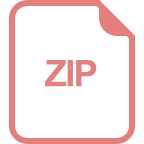
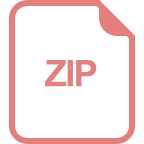
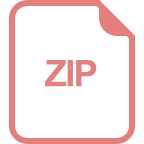
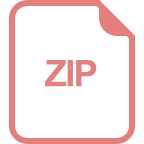
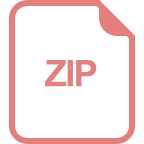
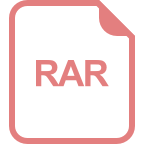
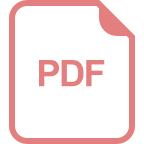
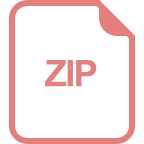