ransac圆拟合matlab
时间: 2023-12-10 13:01:13 浏览: 75
RANSAC(Random Sample Consensus)是一种用于拟合模型的算法,它可以通过对数据集中的样本进行随机抽样来估计模型参数,然后根据估计的参数对数据进行拟合。在MATLAB中,可以使用RANSAC算法来进行圆的拟合。
首先,需要准备要拟合的数据集,包括数据点的坐标和可能的噪声。然后,可以使用MATLAB中的RANSAC函数来进行圆的拟合。该函数会随机抽取一定数量的样本,然后根据这些样本估计圆的参数,再根据参数对整个数据集进行拟合。如果拟合效果不佳,RANSAC会继续进行迭代,直到找到最佳的拟合参数。
在拟合完成后,可以通过绘图函数将拟合的圆和原始数据进行对比,以验证拟合效果。同时,也可以输出拟合的圆的参数,如圆心坐标和半径等信息。
RANSAC算法在拟合圆形数据时具有较好的鲁棒性,可以有效应对数据中的噪声和异常值,因此在实际应用中得到了广泛的应用。在MATLAB中使用RANSAC进行圆拟合可以帮助工程师和科研人员快速、准确地对数据进行分析和建模,提高工作效率并获得可靠的结果。
相关问题
matlab用ransac算法拟合圆
在MATLAB中,可以使用RANSAC算法来拟合一个圆形模型。以下是一个简单的示例代码:
```matlab
% 生成一些带有噪声的圆形数据
r = 5;
theta = linspace(0, 2*pi, 200)';
x = r*cos(theta) + 0.2*randn(size(theta));
y = r*sin(theta) + 0.2*randn(size(theta));
% 添加一些离群点
x(10) = 10;
y(10) = 10;
x(20) = -10;
y(20) = -10;
% 使用RANSAC算法拟合圆形模型
[model, inliers] = ransac([x y], @fit_circle, @dist_circle, @isdegenerate_circle);
% 显示拟合结果
figure;
plot(x, y, '.', 'MarkerSize', 10);
hold on;
plot(x(inliers), y(inliers), 'r.', 'MarkerSize', 10);
t = linspace(0, 2*pi, 100)';
xc = model(1);
yc = model(2);
r = model(3);
plot(xc + r*cos(t), yc + r*sin(t), 'g-', 'LineWidth', 2);
% 定义拟合模型函数
function [model, inliers] = fit_circle(data)
[xc,yc,r] = circfit(data(:,1), data(:,2));
model = [xc, yc, r];
inliers = 1:size(data, 1);
end
% 定义距离函数
function [dist, isinlier] = dist_circle(model, data)
xc = model(1);
yc = model(2);
r = model(3);
dist = sqrt((data(:,1)-xc).^2 + (data(:,2)-yc).^2) - r;
isinlier = abs(dist) < 0.5; % 设置阈值
end
% 定义退化判断函数
function isdegenerate = isdegenerate_circle(data)
isdegenerate = false;
end
```
在上面的代码中,我们首先生成了一些带有噪声的圆形数据,并添加了一些离群点。然后使用RANSAC算法拟合圆形模型,其中`fit_circle`函数用于拟合圆形模型,`dist_circle`函数用于计算点和模型之间的距离,`isdegenerate_circle`函数用于判断数据是否退化。最后,我们使用`plot`函数来显示拟合结果。
matlab 点云Ransac拟合圆
在Matlab中,可以使用点云处理工具箱中的`pcfitcircle`函数来进行圆的RANSAC拟合。以下是一个示例代码:
```matlab
% 生成带噪声的圆形点云
theta = linspace(0, 2*pi, 100)';
r = 10;
x = r*cos(theta) + randn(size(theta));
y = r*sin(theta) + randn(size(theta));
ptCloud = pointCloud([x,y]);
% 使用pcfitcircle函数进行RANSAC拟合
[model,inlierIndices,outlierIndices] = pcfitcircle(ptCloud,r*0.8);
% 可视化结果
figure;
pcshow(ptCloud);
title('Original Point Cloud');
hold on;
plot(model.Center(1),model.Center(2),'rx','MarkerSize',10);
viscircles(model.Center,r,'Color','g');
hold off;
```
在上面的代码中,首先生成一个带噪声的圆形点云,然后使用`pcfitcircle`函数进行RANSAC拟合,得到圆形模型。最后,通过可视化函数`pcshow`和`viscircles`将原始点云和拟合结果可视化出来。
相关推荐
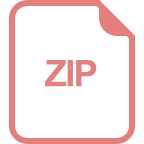
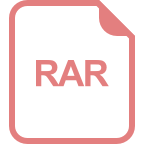












