maltlab灰度质心法
时间: 2024-07-26 07:00:55 浏览: 209
在MATLAB中,灰度质心法(Gray-Level Centroid Method)是一种图像处理技术,用于二值图像分割。它的基本原理是基于像素灰度级别的统计特性。该方法通过计算图像中每个区域的像素灰度值的平均(即质心),将图像划分为不同的区域。
具体步骤如下:
1. 将输入图像转换为二值图像,通常是通过阈值处理或边缘检测等算法。
2. 对于每个连通区域(即由连续黑色像素组成的部分),计算区域内所有像素灰度值的均值(灰度质心)。
3. 根据每个区域质心的灰度值大小,将其归类到相应的类别中。
这种方法简单易行,适用于那些边界明显、颜色对比强烈的图像。然而,对于复杂场景,特别是噪声较多或者边界模糊的情况,其效果可能不太理想。另外,它可能会对亮度变化敏感,因为灰度质心会受到全局亮度的影响。
相关问题
maltlab mse
MSE stands for Mean Square Error, which is a popular metric used to evaluate the performance of regression models in machine learning. It measures the average squared difference between the predicted and actual values of a regression model. In MATLAB, you can calculate MSE using the `mse` function. For example:
```matlab
actual = [1 2 3 4 5];
predicted = [1.2 2.3 3.4 4.5 5.6];
mse_value = mse(actual, predicted);
disp(mse_value);
```
This will calculate the MSE between the `actual` and `predicted` values and display the result.
maltlab2021a
当然可以!这是关于Matlab 2021a的简单教程:
1. 首先,打开Matlab软件。你可以在官方网站上下载并安装它。
2. 在Matlab的主界面上,你会看到一个命令行窗口。你可以在这里输入和运行Matlab的命令。
3. Matlab的基础命令包括数学运算、变量赋值和输出等。例如,你可以尝试计算两个数字的和:输入"3 + 4",然后按回车键。
4. Matlab还支持矩阵运算。你可以使用方括号来定义矩阵,并使用相应的运算符执行矩阵运算。
5. 如果你需要帮助,可以使用Matlab的内置帮助文档。在命令行窗口中,输入"help"加上你想要了解的函数或命令的名称,然后按回车键。例如,输入"help plot"可以获取有关绘图函数的帮助信息。
6. Matlab还提供了许多工具箱,用于特定领域的数据分析和处理。你可以通过点击菜单栏上的
阅读全文
相关推荐
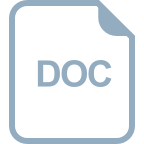
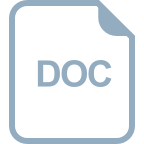
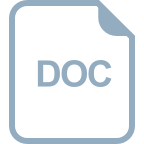
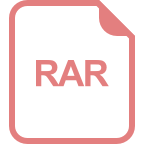












