python实现高斯低通滤波和高斯高通滤波
时间: 2024-06-18 21:05:44 浏览: 125
高斯低通滤波和高斯高通滤波都是图像处理中常见的滤波器,其中高斯低通滤波器可以用于图像平滑处理,而高斯高通滤波器可以用于图像锐化处理。
在Python中,可以使用OpenCV库来实现高斯低通滤波和高斯高通滤波。具体方法如下:
1. 高斯低通滤波:
```
import cv2
import numpy as np
img = cv2.imread('input.jpg')
blur = cv2.GaussianBlur(img, (5, 5), 0) # 进行高斯滤波,(5, 5)表示核的大小,0表示标准差
cv2.imshow('input', img)
cv2.imshow('output', blur)
cv2.waitKey(0)
```
2. 高斯高通滤波:
```
import cv2
import numpy as np
img = cv2.imread('input.jpg')
blur = cv2.GaussianBlur(img, (5, 5), 0) # 进行高斯滤波
sharp = cv2.addWeighted(img, 1.5, blur, -0.5, 0) # 图像锐化处理,可以调整参数值
cv2.imshow('input', img)
cv2.imshow('output', sharp)
cv2.waitKey(0)
```
希望这个回答能够帮助到您。
相关问题
编写一个程序,实现:输入一副图像(灰度图像),实现理想低通、巴特沃斯低通、高斯低通滤波、理想高通、巴特沃斯高通、高斯高通滤波,输出滤波结果图。
以下是Python的实现:
``` python
import cv2
import numpy as np
from matplotlib import pyplot as plt
# 读取图像
img = cv2.imread('input.jpg', 0)
# 傅里叶变换
f = np.fft.fft2(img)
fshift = np.fft.fftshift(f)
# 幅度谱
magnitude_spectrum = 20*np.log(np.abs(fshift))
# 理想低通滤波器
def ideal_lowpass_filter(size, cutoff):
rows, cols = size
crow, ccol = rows/2, cols/2
mask = np.zeros((rows, cols), np.uint8)
mask[int(crow)-cutoff:int(crow)+cutoff, int(ccol)-cutoff:int(ccol)+cutoff] = 1
return mask
# 巴特沃斯低通滤波器
def butterworth_lowpass_filter(size, cutoff, n):
rows, cols = size
crow, ccol = rows/2, cols/2
mask = np.zeros((rows, cols), np.uint8)
for i in range(rows):
for j in range(cols):
distance = np.sqrt((i-crow)**2 + (j-ccol)**2)
mask[i, j] = 1/(1+(distance/cutoff)**(2*n))
return mask
# 高斯低通滤波器
def gaussian_lowpass_filter(size, cutoff):
rows, cols = size
crow, ccol = rows/2, cols/2
x = np.arange(cols)
y = np.arange(rows)
x, y = np.meshgrid(x, y)
mask = np.exp(-((x-ccol)**2+(y-crow)**2)/(2*cutoff**2))
return mask
# 理想高通滤波器
def ideal_highpass_filter(size, cutoff):
rows, cols = size
crow, ccol = rows/2, cols/2
mask = np.ones((rows, cols), np.uint8)
mask[int(crow)-cutoff:int(crow)+cutoff, int(ccol)-cutoff:int(ccol)+cutoff] = 0
return mask
# 巴特沃斯高通滤波器
def butterworth_highpass_filter(size, cutoff, n):
rows, cols = size
crow, ccol = rows/2, cols/2
mask = np.zeros((rows, cols), np.uint8)
for i in range(rows):
for j in range(cols):
distance = np.sqrt((i-crow)**2 + (j-ccol)**2)
mask[i, j] = 1/(1+(cutoff/distance)**(2*n))
return mask
# 高斯高通滤波器
def gaussian_highpass_filter(size, cutoff):
rows, cols = size
crow, ccol = rows/2, cols/2
x = np.arange(cols)
y = np.arange(rows)
x, y = np.meshgrid(x, y)
mask = 1 - np.exp(-((x-ccol)**2+(y-crow)**2)/(2*cutoff**2))
return mask
# 理想低通滤波
ideal_lp = fshift * ideal_lowpass_filter(img.shape, 30)
ideal_lp_img = np.abs(np.fft.ifft2(np.fft.ifftshift(ideal_lp)))
# 巴特沃斯低通滤波
butterworth_lp = fshift * butterworth_lowpass_filter(img.shape, 30, 2)
butterworth_lp_img = np.abs(np.fft.ifft2(np.fft.ifftshift(butterworth_lp)))
# 高斯低通滤波
gaussian_lp = fshift * gaussian_lowpass_filter(img.shape, 30)
gaussian_lp_img = np.abs(np.fft.ifft2(np.fft.ifftshift(gaussian_lp)))
# 理想高通滤波
ideal_hp = fshift * ideal_highpass_filter(img.shape, 30)
ideal_hp_img = np.abs(np.fft.ifft2(np.fft.ifftshift(ideal_hp)))
# 巴特沃斯高通滤波
butterworth_hp = fshift * butterworth_highpass_filter(img.shape, 30, 2)
butterworth_hp_img = np.abs(np.fft.ifft2(np.fft.ifftshift(butterworth_hp)))
# 高斯高通滤波
gaussian_hp = fshift * gaussian_highpass_filter(img.shape, 30)
gaussian_hp_img = np.abs(np.fft.ifft2(np.fft.ifftshift(gaussian_hp)))
# 显示结果
plt.subplot(331), plt.imshow(img, cmap='gray')
plt.title('Input Image'), plt.xticks([]), plt.yticks([])
plt.subplot(332), plt.imshow(magnitude_spectrum, cmap='gray')
plt.title('Magnitude Spectrum'), plt.xticks([]), plt.yticks([])
plt.subplot(333), plt.imshow(ideal_lp_img, cmap='gray')
plt.title('Ideal Lowpass Filter'), plt.xticks([]), plt.yticks([])
plt.subplot(334), plt.imshow(butterworth_lp_img, cmap='gray')
plt.title('Butterworth Lowpass Filter'), plt.xticks([]), plt.yticks([])
plt.subplot(335), plt.imshow(gaussian_lp_img, cmap='gray')
plt.title('Gaussian Lowpass Filter'), plt.xticks([]), plt.yticks([])
plt.subplot(336), plt.imshow(ideal_hp_img, cmap='gray')
plt.title('Ideal Highpass Filter'), plt.xticks([]), plt.yticks([])
plt.subplot(337), plt.imshow(butterworth_hp_img, cmap='gray')
plt.title('Butterworth Highpass Filter'), plt.xticks([]), plt.yticks([])
plt.subplot(338), plt.imshow(gaussian_hp_img, cmap='gray')
plt.title('Gaussian Highpass Filter'), plt.xticks([]), plt.yticks([])
plt.show()
```
其中,`ideal_lowpass_filter`、`butterworth_lowpass_filter`、`gaussian_lowpass_filter`、`ideal_highpass_filter`、`butterworth_highpass_filter`、`gaussian_highpass_filter` 分别为六种滤波器的实现函数。这里的例子中使用的是 30 作为截止频率,可以根据需要进行调整。最终的结果如下图所示:

python opencv 高斯高通滤波
高斯高通滤波是一种图像增强技术,可以通过增强图像中高频信息的方式来增强图像的细节。
使用OpenCV实现高斯高通滤波可以分为以下几步:
1.读取图像并将其转换为灰度图像。
import cv2
img = cv2.imread('input.png')
gray = cv2.cvtColor(img, cv2.COLOR_BGR2GRAY)
2.计算高斯核。
ksize = 3 # 此处选择核的大小为3,可以根据实际情况进行调整
sigma = 1.5 # 此处选择sigma=1.5,可以根据实际情况进行调整
kernel = cv2.getGaussianKernel(ksize, sigma)
kernel = kernel * kernel.T # 使高斯核变成二维
3.进行高斯卷积。
gaussian = cv2.filter2D(gray, -1, kernel)
4.进行高通滤波。
alpha = 1.5 # 此处选择alpha=1.5,可以根据实际情况进行调整
beta = 0.5 # 此处选择beta=0.5,可以根据实际情况进行调整
highpass = gray - beta * gaussian
highpass = cv2.convertScaleAbs(alpha * highpass)
5.将处理后的图像进行保存。
cv2.imwrite('output.png', highpass)
完整代码如下:
import cv2
img = cv2.imread('input.png')
gray = cv2.cvtColor(img, cv2.COLOR_BGR2GRAY)
ksize = 3
sigma = 1.5
kernel = cv2.getGaussianKernel(ksize, sigma)
kernel = kernel * kernel.T
gaussian = cv2.filter2D(gray, -1, kernel)
alpha = 1.5
beta = 0.5
highpass = gray - beta * gaussian
highpass = cv2.convertScaleAbs(alpha * highpass)
cv2.imwrite('output.png', highpass)
阅读全文
相关推荐
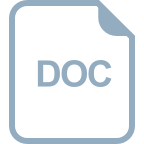
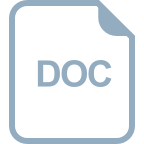
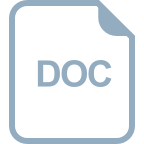
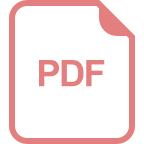
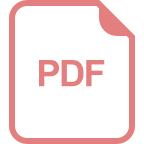
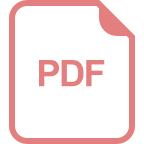







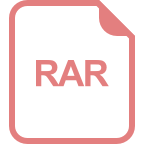


