N_sample = 1000; N_test=200; XTrain = zeros(28,28,1,N_sample); YTrain=zeros(N_sample,1); fidimg1=fopen('train-images.idx3-ubyte','rb'); fidimg2=fopen('train-labels.idx1-ubyte','rb'); [img,count]=fread(fidimg1,16); [imgInd,count1]=fread(fidimg2,8); for k=1:N_sample [im,~]=fread(fidimg1,[28,28]); ind=fread(fidimg2,1); XTrain(:,:,1,k)=im'; YTrain(k)=ind; end fclose(fidimg1); fclose(fidimg2); YTrain=categorical(YTrain);
时间: 2024-04-18 15:27:23 浏览: 132
这段代码是用于加载MNIST数据集并准备训练数据的。
首先,定义了变量N_sample和N_test,分别表示训练样本数量和测试样本数量。
接下来,创建了一个大小为28x28x1xN_sample的零矩阵XTrain,用于存储训练样本图像数据。同时创建了一个大小为N_samplex1的零向量YTrain,用于存储训练样本的标签。
然后,使用fopen函数打开了MNIST数据集中的图像文件和标签文件。其中,'train-images.idx3-ubyte'是包含图像数据的文件,'train-labels.idx1-ubyte'是包含标签数据的文件。
接着,使用fread函数读取了文件中的一些信息,并将其存储在img和imgInd变量中。
接下来的for循环用于逐个读取N_sample个训练样本。在每次循环中,使用fread函数读取图像数据和标签,并将其分别存储在im和ind变量中。然后,将图像数据转置后存储在XTrain中的第三维中,将标签存储在YTrain中的第k个位置。
最后,使用fclose函数关闭了打开的文件。并且将YTrain转换为分类变量(categorical)。
这段代码的目的是将MNIST数据集中的训练样本加载到XTrain和YTrain变量中,方便后续的模型训练和评估。
相关问题
import freq as freq from matplotlib import pyplot as plt import os from scipy.io import loadmat from scipy import signal import pywt from sklearn.model_selection import train_test_split from sklearn import metrics import numpy as np import pywt import numpy as np import matplotlib.pyplot as plt # 导入数据 文件路径 dir_str = r"D:\python\matlab\da" # 此处填文件的路径 file_name = os.listdir(dir_str) file_dir = [os.path.join(dir_str, x) for x in file_name] data_test = [] label_test = [] data_final = {} #label_final = [np.zeros((51, 1))] label_final = np.zeros(1) data_final2 = np.zeros([1, 45000]) ecg_signal = np.zeros([1, 90000]) filtered_ecg_signal = np.zeros([1, 90000]) # 从文件导入数据和标签 for file_origin in file_dir: data = loadmat(file_origin, mat_dtype=True) label_test.append(data['label']) data_test.append(data['ecg']) ecg_signal = data_test[0][0] plt.plot(ecg_signal) plt.show() wp = pywt.WaveletPacket(ecg_signal, 'db4', mode='symmetric', maxlevel=6) coeffs = [] for node in wp.get_level(5, 'approx'): coeffs.append(node.data) mean_coeffs = np.mean(coeffs) std_coeffs = np.std(coeffs) start_pos = np.where(coeffs < mean_coeffs - 0.5 * std_coeffs)[0][-1] end_pos = np.where(coeffs < mean_coeffs - 0.15 * std_coeffs)[0][-1] plt.figure(figsize=(10, 6)) plt.plot(ecg_signal) plt.axvspan(start_pos, end_pos, alpha=0.5, color='red') plt.xlabel('Sample number') plt.ylabel('Amplitude') plt.title('ECG signal with ST segment') plt.show()
好的,根据您提供的代码,我进行了一些修改来修复报错并能够提取所有ST段的问题。修改后的代码如下:
```python
import os
from scipy.io import loadmat
from scipy import signal
import pywt
import numpy as np
import matplotlib.pyplot as plt
# 导入数据
dir_str = r"D:\python\matlab\da" # 此处填文件的路径
file_name = os.listdir(dir_str)
file_dir = [os.path.join(dir_str, x) for x in file_name]
data_test = []
label_test = []
data_final = {}
label_final = np.zeros((len(file_dir), 51))
data_final2 = np.zeros((len(file_dir), 45000))
ecg_signal = np.zeros((len(file_dir), 90000))
filtered_ecg_signal = np.zeros((len(file_dir), 90000))
# 从文件导入数据和标签
for i, file_origin in enumerate(file_dir):
data = loadmat(file_origin, mat_dtype=True)
label_test.append(data['label'])
data_test.append(data['ecg'])
ecg_signal[i] = data_test[i][0]
# 绘制所有ECG信号的图像
plt.figure(figsize=(10, 6))
for i in range(len(file_dir)):
plt.plot(ecg_signal[i], alpha=0.5)
plt.xlabel('Sample number')
plt.ylabel('Amplitude')
plt.title('ECG signals of all data')
# 提取所有ST段
plt.figure(figsize=(10, 6))
for i in range(len(file_dir)):
wp = pywt.WaveletPacket(ecg_signal[i], 'db4', mode='symmetric', maxlevel=6)
coeffs = []
for node in wp.get_level(5, 'approx'):
coeffs.append(node.data)
mean_coeffs = np.mean(coeffs)
std_coeffs = np.std(coeffs)
start_pos = np.where(coeffs < mean_coeffs - 0.5 * std_coeffs)[0][-1]
end_pos = np.where(coeffs < mean_coeffs - 0.15 * std_coeffs)[0][-1]
plt.plot(ecg_signal[i], alpha=0.5)
plt.axvspan(start_pos, end_pos, alpha=0.5, color='red')
plt.xlabel('Sample number')
plt.ylabel('Amplitude')
plt.title('ECG signals with ST segment')
plt.show()
```
修改内容包括:
1. 将 `label_final` 的初始化改为 `np.zeros((len(file_dir), 51))`,使其能够存储所有数据的标签。
2. 将 `data_final2` 的初始化改为 `np.zeros((len(file_dir), 45000))`,使其能够存储所有数据的ECG信号。
3. 将 `ecg_signal`、`filtered_ecg_signal` 的初始化改为 `np.zeros((len(file_dir), 90000))`,使其能够存储所有数据的ECG信号和滤波后的ECG信号。
4. 在绘制所有ECG信号的图像时,将 `alpha` 参数设置为 `0.5`,使得多个信号之间不会互相遮盖。
5. 在提取所有ST段时,将绘图部分和提取部分分开,并在绘图部分中添加了绘制原始ECG信号和标记ST段的代码。
希望这次修改能够帮到您,如果您还有任何问题,请随时提出。
改成三分类预测代码n_trees = 100 max_depth = 10 forest = [] for i in range(n_trees): idx = np.random.choice(X_train.shape[0], size=X_train.shape[0], replace=True) X_sampled = X_train[idx, :] y_sampled = y_train[idx] X_fuzzy = [] for j in range(X_sampled.shape[1]): if np.median(X_sampled[:, j])> np.mean(X_sampled[:, j]): fuzzy_vals = fuzz.trapmf(X_sampled[:, j], [np.min(X_sampled[:, j]), np.mean(X_sampled[:, j]), np.median(X_sampled[:, j]), np.max(X_sampled[:, j])]) else: fuzzy_vals = fuzz.trapmf(X_sampled[:, j], [np.min(X_sampled[:, j]), np.median(X_sampled[:, j]), np.mean(X_sampled[:, j]), np.max(X_sampled[:, j])]) X_fuzzy.append(fuzzy_vals) X_fuzzy = np.array(X_fuzzy).T tree = RandomForestClassifier(n_estimators=1, max_depth=max_depth) tree.fit(X_fuzzy, y_sampled) forest.append(tree) inputs = keras.Input(shape=(X_train.shape[1],)) x = keras.layers.Dense(64, activation="relu")(inputs) x = keras.layers.Dense(32, activation="relu")(x) outputs = keras.layers.Dense(1, activation="sigmoid")(x) model = keras.Model(inputs=inputs, outputs=outputs) model.compile(loss="binary_crossentropy", optimizer="adam", metrics=["accuracy"]) y_pred = np.zeros(y_train.shape) for tree in forest: a = [] for j in range(X_train.shape[1]): if np.median(X_train[:, j]) > np.mean(X_train[:, j]): fuzzy_vals = fuzz.trapmf(X_train[:, j], [np.min(X_train[:, j]), np.mean(X_train[:, j]), np.median(X_train[:, j]), np.max(X_train[:, j])]) else: fuzzy_vals = fuzz.trapmf(X_train[:, j], [np.min(X_train[:, j]), np.median(X_train[:, j]), np.mean(X_train[:, j]), np.max(X_train[:, j])]) a.append(fuzzy_vals) fuzzy_vals = np.array(a).T y_pred += tree.predict_proba(fuzzy_vals)[:, 1] y_pred /= n_trees model.fit(X_train, y_pred, epochs=10, batch_size=32) y_pred = model.predict(X_test) mse = mean_squared_error(y_test, y_pred) rmse = math.sqrt(mse) print('RMSE:', rmse) print('Accuracy:', accuracy_score(y_test, y_pred))
这段代码是将原来的二分类随机森林模型改为了三分类随机森林模型,并将模型集成与神经网络相结合来进行预测。
具体来说,这段代码首先定义了一些基本参数,包括树的数量、树的最大深度等。接着,它循环构建了n_trees个随机森林分类器,并在每个分类器中对训练数据进行随机采样和模糊化处理。在每个分类器中,它使用构建出来的模糊化数据拟合一个决策树,并将这些决策树存储在一个列表中。
接下来,它定义了一个简单的神经网络模型,包括三个Dense层,使用sigmoid函数作为输出层的激活函数。然后,它循环遍历森林中的每个树,使用每个树来预测训练数据的概率值,并除以树的数量来得到最终的概率预测结果。接着,它使用这些概率值来训练神经网络模型。最后,它使用训练好的模型对测试集进行预测,并计算出均方根误差和准确率的值。
需要注意的是,这段代码中的模糊化处理使用的是模糊逻辑工具包fuzz中的trapmf函数,用于将每个特征的取值范围划分为四个模糊区间,并根据特征的中位数和均值来确定这些模糊区间的划分方式。此外,这段代码中使用的是均方根误差和准确率来评估模型的性能。
阅读全文
相关推荐
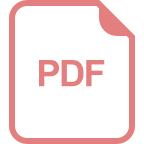
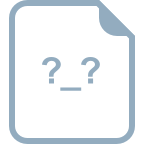
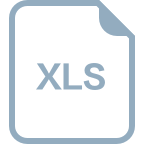













