screen detech
时间: 2024-03-31 22:08:13 浏览: 15
屏幕检测是指识别和分析计算机屏幕上显示的图像或内容的过程。它可以用于许多应用,如图像处理、计算机视觉、自动化测试等。屏幕检测通常涉及以下几个步骤:
1. 屏幕截取:使用截屏工具或编程接口捕捉计算机屏幕的图像。
2. 图像预处理:对截取的图像进行预处理,如裁剪、缩放、去噪等,以便后续处理。
3. 物体识别:使用计算机视觉技术,如图像分类、目标检测、语义分割等,来识别屏幕上的物体或内容。
4. 特征提取:从识别到的物体或内容中提取有用的特征,如颜色、形状、纹理等。
5. 决策和应用:根据提取的特征进行决策或应用,如自动化测试、图像分析、用户界面交互等。
屏幕检测涉及多个领域的知识,包括计算机视觉、机器学习、图像处理等。具体的实现方法和技术选择会根据具体的应用需求和实际情况而定。
相关问题
detect pyautogui
根据提供的引用内容,可以看出pyautogui是一个Python库,用于自动化鼠标和键盘操作。它可以在不同的操作系统上模拟鼠标和键盘的输入,以及进行屏幕截图和图像识别等功能。
关于pyautogui的检测,可以通过以下步骤进行:
1. 首先,确保已经安装了pyautogui库。可以使用以下命令来安装:
```shell
pip install pyautogui
```
2. 在Python脚本中导入pyautogui库:
```python
import pyautogui
```
3. 如果没有报错,说明pyautogui库已经成功安装并可以正常使用。
4. 可以尝试使用pyautogui库的一些功能,例如模拟鼠标移动、点击、键盘输入等操作,以验证pyautogui是否正常工作。
以下是一个示例代码,演示了如何使用pyautogui模拟鼠标移动和点击操作:
```python
import pyautogui
# 获取屏幕尺寸
screen_width, screen_height = pyautogui.size()
# 将鼠标移动到屏幕中心
pyautogui.moveTo(screen_width / 2, screen_height / 2)
# 模拟鼠标点击
pyautogui.click()
```
请注意,pyautogui的具体用法和功能可以根据具体需求进行进一步学习和探索。
修改此代码使其可重复运行import pygame import sys from pygame.locals import * from robomaster import * import cv2 import numpy as np focal_length = 750 # 焦距 known_radius = 2 # 已知球的半径 def calculate_distance(focal_length, known_radius, perceived_radius): distance = (known_radius * focal_length) / perceived_radius return distance def show_video(ep_robot, screen): 获取机器人第一视角图像帧 img = ep_robot.camera.read_cv2_image(strategy="newest") 转换图像格式,转换为pygame的surface对象 img = cv2.cvtColor(img, cv2.COLOR_BGR2RGB) img = cv2.transpose(img) # 行列互换 img = pygame.surfarray.make_surface(img) screen.blit(img, (0, 0)) # 绘制图像 def detect_white_circle(ep_robot): 获取机器人第一视角图像帧 img = ep_robot.camera.read_cv2_image(strategy="newest") 转换为灰度图像 gray = cv2.cvtColor(img, cv2.COLOR_BGR2GRAY) 进行中值滤波处理 gray = cv2.medianBlur(gray, 5) 检测圆形轮廓 circles = cv2.HoughCircles(gray, cv2.HOUGH_GRADIENT, 1, 50, param1=160, param2=40, minRadius=5, maxRadius=60) if circles is not None: circles = np.uint16(np.around(circles)) for circle in circles[0, :]: center = (circle[0], circle[1]) known_radius = circle 在图像上绘制圆形轮廓 cv2.circle(img, center, known_radius, (0, 255, 0), 2) 显示图像 distance = calculate_distance(focal_length, known_radius, known_radius) 在图像上绘制圆和距离 cv2.circle(img, center, known_radius, (0, 255, 0), 2) cv2.putText(img, f"Distance: {distance:.2f} cm", (center[0] - known_radius, center[1] - 10), cv2.FONT_HERSHEY_SIMPLEX, 0.5, (0, 255, 0), 2) cv2.imshow("White Circle Detection", img) cv2.waitKey(1) def main(): pygame.init() screen_size = width, height = 1280, 720 screen = pygame.display.set_mode(screen_size) ep_robot = robot.Robot() ep_robot.initialize(conn_type='ap') version = ep_robot.get_version() print("Robot version: {0}".format(version)) ep_robot.camera.start_video_stream(display=False) pygame.time.wait(100) clock = pygame.time.Clock() while True: clock.tick(5) # 将帧数设置为25帧 for event in pygame.event.get(): if event.type == QUIT: ep_robot.close() pygame.quit() sys.exit() show_video(ep_robot, screen) detect_white_circle(ep_robot) if name == 'main': main()
修改后的代码如下所示:
```python
import pygame
import sys
from pygame.locals import *
from robomaster import robot
import cv2
import numpy as np
focal_length = 750 # 焦距
known_radius = 2 # 已知球的半径
def calculate_distance(focal_length, known_radius, perceived_radius):
distance = (known_radius * focal_length) / perceived_radius
return distance
def show_video(ep_robot, screen):
# 获取机器人第一视角图像帧
img = ep_robot.camera.read_cv2_image(strategy="newest")
# 转换图像格式,转换为pygame的surface对象
img = cv2.cvtColor(img, cv2.COLOR_BGR2RGB)
img = cv2.transpose(img) # 行列互换
img = pygame.surfarray.make_surface(img)
screen.blit(img, (0, 0)) # 绘制图像
def detect_white_circle(ep_robot):
# 获取机器人第一视角图像帧
img = ep_robot.camera.read_cv2_image(strategy="newest")
# 转换为灰度图像
gray = cv2.cvtColor(img, cv2.COLOR_BGR2GRAY)
# 进行中值滤波处理
gray = cv2.medianBlur(gray, 5)
# 检测圆形轮廓
circles = cv2.HoughCircles(gray, cv2.HOUGH_GRADIENT, 1, 50, param1=160, param2=40, minRadius=5, maxRadius=60)
if circles is not None:
circles = np.uint16(np.around(circles))
for circle in circles[0, :]:
center = (circle[0], circle[1])
known_radius = circle[2]
# 在图像上绘制圆形轮廓
cv2.circle(img, center, known_radius, (0, 255, 0), 2)
# 计算距离
distance = calculate_distance(focal_length, known_radius, known_radius)
# 在图像上绘制圆和距离
cv2.circle(img, center, known_radius, (0, 255, 0), 2)
cv2.putText(img, f"Distance: {distance:.2f} cm", (center[0] - known_radius, center[1] - 10),
cv2.FONT_HERSHEY_SIMPLEX, 0.5, (0, 255, 0), 2)
cv2.imshow("White Circle Detection", img)
cv2.waitKey(1)
def main():
pygame.init()
screen_size = width, height = 1280, 720
screen = pygame.display.set_mode(screen_size)
ep_robot = robot.Robot()
ep_robot.initialize(conn_type='ap')
version = ep_robot.get_version()
print("Robot version: {0}".format(version))
ep_robot.camera.start_video_stream(display=False)
pygame.time.wait(100)
clock = pygame.time.Clock()
while True:
clock.tick(5) # 将帧数设置为25帧
for event in pygame.event.get():
if event.type == QUIT:
ep_robot.close()
pygame.quit()
sys.exit()
show_video(ep_robot, screen)
detect_white_circle(ep_robot)
if __name__ == '__main__':
main()
```
相关推荐
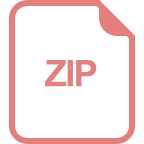
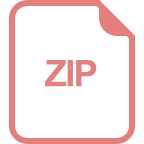
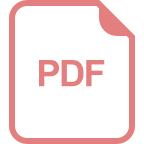













