os.path.isdir() #检验
时间: 2024-08-15 16:05:26 浏览: 41
`os.path.isdir()` 是Python标准库 `os.path` 中的一个函数,用于判断给定路径是否为目录(即是否指向一个存在并且是一个目录的文件)。它返回一个布尔值:如果路径确实是一个目录,则返回 True;如果不是目录(比如路径不存在或者指向的是文件),则返回 False。
下面是如何使用 `os.path.isdir()` 函数的例子:
```python
import os
# 检查当前工作目录是否是一个目录
if os.path.isdir('.'):
print("当前目录是一个存在的目录")
else:
print("当前目录可能不存在或不是一个目录")
# 创建一个新目录并检查它是否存在
try:
os.mkdir('/tmp/new_dir')
except FileExistsError:
pass # 已经存在这个目录
new_dir_path = '/tmp/new_dir'
if os.path.isdir(new_dir_path):
print(f"{new_dir_path} 是一个存在的目录")
else:
print(f"{new_dir_path} 不是一个目录或不存在")
```
### 相关问题 - os.path.isdir():
1. 如何确保在执行操作之前文件夹已存在?
2. `os.path.isdir()` 能否检测符号链接指向的是目录还是文件?
3. 如果目录不存在时调用 `os.path.isdir()`,会发生什么?
相关问题
详细解释一下这段代码,每一句都要进行注解:for dataset in datasets: print(dataset) if dataset not in out_results: out_results[dataset] = {} for scene in data_dict[dataset]: print(scene) # Fail gently if the notebook has not been submitted and the test data is not populated. # You may want to run this on the training data in that case? img_dir = f'{src}/test/{dataset}/{scene}/images' if not os.path.exists(img_dir): continue # Wrap the meaty part in a try-except block. try: out_results[dataset][scene] = {} img_fnames = [f'{src}/test/{x}' for x in data_dict[dataset][scene]] print (f"Got {len(img_fnames)} images") feature_dir = f'featureout/{dataset}{scene}' if not os.path.isdir(feature_dir): os.makedirs(feature_dir, exist_ok=True) t=time() index_pairs = get_image_pairs_shortlist(img_fnames, sim_th = 0.5644583, # should be strict min_pairs = 33, # we select at least min_pairs PER IMAGE with biggest similarity exhaustive_if_less = 20, device=device) t=time() -t timings['shortlisting'].append(t) print (f'{len(index_pairs)}, pairs to match, {t:.4f} sec') gc.collect() t=time() if LOCAL_FEATURE != 'LoFTR': detect_features(img_fnames, 2048, feature_dir=feature_dir, upright=True, device=device, resize_small_edge_to=600 ) gc.collect() t=time() -t timings['feature_detection'].append(t) print(f'Features detected in {t:.4f} sec') t=time() match_features(img_fnames, index_pairs, feature_dir=feature_dir,device=device) else: match_loftr(img_fnames, index_pairs, feature_dir=feature_dir, device=device, resize_to=(600, 800)) t=time() -t timings['feature_matching'].append(t) print(f'Features matched in {t:.4f} sec') database_path = f'{feature_dir}/colmap.db' if os.path.isfile(database_path): os.remove(database_path) gc.collect() import_into_colmap(img_dir, feature_dir=feature_dir,database_path=database_path) output_path = f'{feature_dir}/colmap_rec_{LOCAL_FEATURE}' t=time() pycolmap.match_exhaustive(database_path) t=time() - t timings['RANSAC'].append(t) print(f'RANSAC in {t:.4f} sec')
这段代码是Python代码,用于图像匹配和三维重建。以下是每行代码的注释:
```python
for dataset in datasets: # 遍历数据集列表
print(dataset) # 输出当前数据集
if dataset not in out_results: # 如果当前数据集不在输出结果中
out_results[dataset] = {} # 添加一个空字典作为该数据集的输出结果
for scene in data_dict[dataset]: # 遍历当前数据集中的场景
print(scene) # 输出当前场景
# 如果在测试集中,但该场景的图像数据未被提供,则跳过
img_dir = f'{src}/test/{dataset}/{scene}/images'
if not os.path.exists(img_dir):
continue
try: # 尝试匹配图像并执行三维重建
out_results[dataset][scene] = {} # 添加一个空字典作为该场景的输出结果
img_fnames = [f'{src}/test/{x}' for x in data_dict[dataset][scene]] # 获取当前场景中的图像文件名列表
print(f"Got {len(img_fnames)} images")
feature_dir = f'featureout/{dataset}{scene}' # 设置特征输出目录
if not os.path.isdir(feature_dir): # 如果特征输出目录不存在,则创建该目录
os.makedirs(feature_dir, exist_ok=True)
t = time()
# 获取图像对的候选列表
index_pairs = get_image_pairs_shortlist(img_fnames, sim_th=0.5644583, min_pairs=33,
exhaustive_if_less=20, device=device)
t = time() - t
timings['shortlisting'].append(t)
print(f'{len(index_pairs)}, pairs to match, {t:.4f} sec')
gc.collect() # 执行垃圾回收以释放内存
t = time()
# 如果不使用LoFTR,则检测图像中的特征点
if LOCAL_FEATURE != 'LoFTR':
detect_features(img_fnames, 2048, feature_dir=feature_dir, upright=True,
device=device, resize_small_edge_to=600)
gc.collect()
t = time() - t
timings['feature_detection'].append(t)
print(f'Features detected in {t:.4f} sec')
# 匹配图像中的特征点
match_features(img_fnames, index_pairs, feature_dir=feature_dir, device=device)
# 如果使用LoFTR,则使用LoFTR进行特征匹配
else:
match_loftr(img_fnames, index_pairs, feature_dir=feature_dir, device=device,
resize_to=(600, 800))
t = time() - t
timings['feature_matching'].append(t)
print(f'Features matched in {t:.4f} sec')
database_path = f'{feature_dir}/colmap.db'
if os.path.isfile(database_path):
os.remove(database_path)
gc.collect()
# 将特征匹配结果导入到COLMAP数据库中
import_into_colmap(img_dir, feature_dir=feature_dir, database_path=database_path)
output_path = f'{feature_dir}/colmap_rec_{LOCAL_FEATURE}'
t = time()
# 使用COLMAP执行RANSAC算法进行三维重建
pycolmap.match_exhaustive(database_path)
t = time() - t
timings['RANSAC'].append(t)
print(f'RANSAC in {t:.4f} sec')
except Exception as e: # 捕获任何异常
print(f'Scene {scene} failed. Error: {e}')
```
此代码的主要目的是使用图像匹配和三维重建技术来重建场景。在此过程中,它使用了许多库和函数,例如os、time、gc、pycolmap等。
yolov7 test.py详解
YoloV7是目标检测算法YOLO的最新版本,相较于之前的版本,它在模型结构、训练策略和速度等方面都有了较大的改进。test.py文件是用于测试已经训练好的模型的脚本,下面是对test.py文件的详细解释:
1. 导入必要的库和模块
```python
import argparse
import os
import platform
import shutil
import time
from pathlib import Path
import cv2
import torch
import torch.backends.cudnn as cudnn
import numpy as np
from models.experimental import attempt_load
from utils.datasets import LoadStreams, LoadImages
from utils.general import check_img_size, check_requirements, check_imshow, \
non_max_suppression, apply_classifier, scale_coords, xyxy2xywh, strip_optimizer, set_logging
from utils.plots import plot_one_box
from utils.torch_utils import select_device, load_classifier, time_synchronized
```
这里导入了一些必要的库和模块,比如PyTorch、OpenCV、NumPy等,以及用于测试的模型、数据集和一些工具函数。
2. 定义输入参数
```python
parser = argparse.ArgumentParser()
parser.add_argument('--weights', nargs='+', type=str, default='yolov5s.pt', help='model.pt path(s)')
parser.add_argument('--source', type=str, default='data/images', help='source')
parser.add_argument('--img-size', type=int, default=640, help='inference size (pixels)')
parser.add_argument('--conf-thres', type=float, default=0.25, help='object confidence threshold')
parser.add_argument('--iou-thres', type=float, default=0.45, help='IOU threshold for NMS')
parser.add_argument('--device', default='', help='cuda device, i.e. 0 or 0,1,2,3 or cpu')
parser.add_argument('--view-img', action='store_true', help='display results')
parser.add_argument('--save-txt', action='store_true', help='save results to *.txt')
parser.add_argument('--save-conf', action='store_true', help='save confidences in --save-txt labels')
parser.add_argument('--save-crop', action='store_true', help='save cropped prediction boxes')
parser.add_argument('--nosave', action='store_true', help='do not save images/videos')
parser.add_argument('--classes', nargs='+', type=int, help='filter by class: --class 0, or --class 0 2 3')
parser.add_argument('--agnostic-nms', action='store_true', help='class-agnostic NMS')
parser.add_argument('--augment', action='store_true', help='augmented inference')
parser.add_argument('--update', action='store_true', help='update all models')
parser.add_argument('--project', default='runs/detect', help='save results to project/name')
parser.add_argument('--name', default='exp', help='save results to project/name')
parser.add_argument('--exist-ok', action='store_true', help='existing project/name ok, do not increment')
opt = parser.parse_args()
```
这里使用Python的argparse库来定义输入参数,包括模型权重文件、输入数据源、推理尺寸、置信度阈值、NMS阈值等。
3. 加载模型
```python
# 加载模型
model = attempt_load(opt.weights, map_location=device) # load FP32 model
imgsz = check_img_size(opt.img_size, s=model.stride.max()) # check img_size
if device.type != 'cpu':
model(torch.zeros(1, 3, imgsz, imgsz).to(device).type_as(next(model.parameters()))) # run once
```
这里使用`attempt_load()`函数来加载模型,该函数会根据传入的权重文件路径自动选择使用哪个版本的YoloV7模型。同时,这里还会检查输入图片的大小是否符合模型的要求。
4. 设置计算设备
```python
# 设置计算设备
device = select_device(opt.device)
half = device.type != 'cpu' # half precision only supported on CUDA
# Initialize model
model.to(device).eval()
```
这里使用`select_device()`函数来选择计算设备(GPU或CPU),并将模型移动到选择的设备上。
5. 加载数据集
```python
# 加载数据集
if os.path.isdir(opt.source):
dataset = LoadImages(opt.source, img_size=imgsz)
else:
dataset = LoadStreams(opt.source, img_size=imgsz)
```
根据输入参数中的数据源,使用`LoadImages()`或`LoadStreams()`函数来加载数据集。这两个函数分别支持从图片文件夹或摄像头/视频中读取数据。
6. 定义类别和颜色
```python
# 定义类别和颜色
names = model.module.names if hasattr(model, 'module') else model.names
colors = [[np.random.randint(0, 255) for _ in range(3)] for _ in names]
```
这里从模型中获取类别名称,同时为每个类别随机生成一个颜色,用于在图片中绘制框和标签。
7. 定义输出文件夹
```python
# 定义输出文件夹
save_dir = Path(increment_path(Path(opt.project) / opt.name, exist_ok=opt.exist_ok)) # increment run
(save_dir / 'labels' if opt.save_txt else save_dir).mkdir(parents=True, exist_ok=True) # make dir
```
这里使用`increment_path()`函数来生成输出文件夹的名称,同时创建相应的文件夹。
8. 开始推理
```python
# 开始推理
for path, img, im0s, vid_cap in dataset:
t1 = time_synchronized()
# 图像预处理
img = torch.from_numpy(img).to(device)
img = img.half() if half else img.float()
img /= 255.0
if img.ndimension() == 3:
img = img.unsqueeze(0)
# 推理
pred = model(img)[0]
# 后处理
pred = non_max_suppression(pred, opt.conf_thres, opt.iou_thres, classes=opt.classes, agnostic=opt.agnostic_nms)
t2 = time_synchronized()
# 处理结果
for i, det in enumerate(pred): # detections per image
if webcam: # batch_size >= 1
p, s, im0 = path[i], f'{i}: ', im0s[i].copy()
else:
p, s, im0 = path, '', im0s
save_path = str(save_dir / p.name)
txt_path = str(save_dir / 'labels' / p.stem) + ('' if dataset.mode == 'image' else f'_{counter}') + '.txt'
if det is not None and len(det):
det[:, :4] = scale_coords(img.shape[2:], det[:, :4], im0.shape).round()
for *xyxy, conf, cls in reversed(det):
c = int(cls)
label = f'{names[c]} {conf:.2f}'
plot_one_box(xyxy, im0, label=label, color=colors[c], line_thickness=3)
if opt.save_conf:
with open(txt_path, 'a') as f:
f.write(f'{names[c]} {conf:.2f}\n')
if opt.save_crop:
w = int(xyxy[2] - xyxy[0])
h = int(xyxy[3] - xyxy[1])
x1 = int(xyxy[0])
y1 = int(xyxy[1])
x2 = int(xyxy[2])
y2 = int(xyxy[3])
crop_img = im0[y1:y2, x1:x2]
crop_path = save_path + f'_{i}_{c}.jpg'
cv2.imwrite(crop_path, crop_img)
# 保存结果
if opt.nosave:
pass
elif dataset.mode == 'images':
cv2.imwrite(save_path, im0)
else:
if vid_path != save_path: # new video
vid_path = save_path
if isinstance(vid_writer, cv2.VideoWriter):
vid_writer.release() # release previous video writer
fourcc = 'mp4v' # output video codec
fps = vid_cap.get(cv2.CAP_PROP_FPS)
w = int(vid_cap.get(cv2.CAP_PROP_FRAME_WIDTH))
h = int(vid_cap.get(cv2.CAP_PROP_FRAME_HEIGHT))
vid_writer = cv2.VideoWriter(save_path, cv2.VideoWriter_fourcc(*fourcc), fps, (w, h))
vid_writer.write(im0)
# 打印结果
print(f'{s}Done. ({t2 - t1:.3f}s)')
# 释放资源
if cv2.waitKey(1) == ord('q'): # q to quit
raise StopIteration
elif cv2.waitKey(1) == ord('p'): # p to pause
cv2.waitKey(-1)
```
这里使用一个循环来遍历数据集中的所有图像或视频帧,对每张图像或视频帧进行以下操作:
- 图像预处理:将图像转换为PyTorch张量,并进行归一化和类型转换。
- 推理:将图像张量传入模型进行推理,得到预测结果。
- 后处理:对预测结果进行非极大值抑制、类别筛选等后处理操作,得到最终的检测结果。
- 处理结果:对每个检测框进行标签和颜色的绘制,同时可以选择保存检测结果的图片或视频以及标签信息的TXT文件。
- 释放资源:根据按键输入决定是否退出或暂停程序。
9. 总结
以上就是YoloV7的测试脚本test.py的详细解释,通过这个脚本可以方便地测试已经训练好的模型,并对检测结果进行可视化和保存等操作。
相关推荐
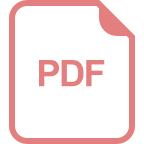
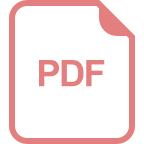











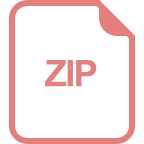
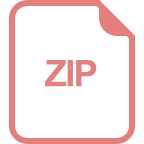
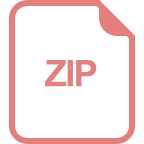
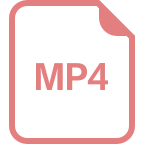