决策曲线DCA代码 python
时间: 2024-10-07 13:03:13 浏览: 110
决策曲线(Decision Curve Analysis, DCA)是一种统计分析工具,用于评估预测模型在不同阈值下的临床决策效果。在Python中,虽然没有直接内置的库来计算DCA,但我们通常会结合`pandas`、`matplotlib`以及`scikit-learn`等库进行数据处理和可视化。
以下是一个简单的步骤说明如何使用这些库实现DCA:
1. 导入必要的库:
```python
import pandas as pd
import matplotlib.pyplot as plt
from sklearn.metrics import roc_curve, roc_auc_score
```
2. 准备数据,包括真实标签和模型预测概率:
```python
y_true = your_data['true_labels']
probs = your_model.predict_proba(your_data)[:, 1] # 对于二分类任务
```
3. 计算每个阈值下的真正率和假正率:
```python
fpr, tpr, thresholds = roc_curve(y_true, probs)
```
4. 创建决策曲线图:
```python
plt.plot(fpr, tpr, label='ROC curve')
plt.plot([0, 1], [0, 1], 'k--', label='Chance') # 随机猜测线
for threshold in thresholds:
y_pred_binary = (probs > threshold).astype(int)
df = pd.DataFrame({'True Positives': y_true[y_pred_binary],
'False Positives': ~y_true[y_pred_binary]})
df.plot(kind='bar', stacked=True, ax=plt.gca(), legend=False)
plt.axhline(df.iloc[-1].mean(), color='grey', linestyle='--')
plt.xlabel('False Positive Rate')
plt.ylabel('True Positive Rate')
plt.title('Decision Curve Analysis')
plt.legend()
```
5. 显示图形:
```python
plt.show()
```
阅读全文
相关推荐
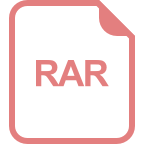
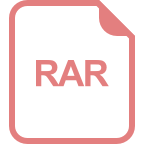
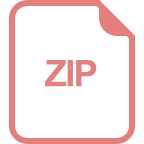
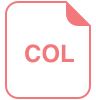
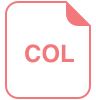

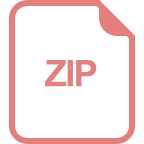
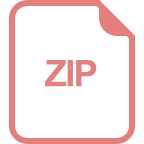