用python编写一个摄影测量学的连续法相对定向程序
时间: 2024-11-09 12:22:39 浏览: 140
编写一个完整的摄影测量学的连续法相对定向程序是一个复杂的过程,涉及到计算机视觉、图像处理以及几何建模等专业知识。这里提供一个简化的Python示例,展示了如何使用OpenCV库来进行基本的特征匹配,这是连续法相对定向的一部分步骤。请注意,这只是一个基础框架,并未涵盖全部细节,实际项目需要深度学习和专业的摄影测量算法。
```python
import cv2
import numpy as np
# 加载两幅图像
image1 = cv2.imread('image1.jpg', cv2.IMREAD_GRAYSCALE)
image2 = cv2.imread('image2.jpg', cv2.IMREAD_GRAYSCALE)
# 特征检测与描述
sift = cv2.xfeatures2d.SIFT_create()
keypoints1, descriptors1 = sift.detectAndCompute(image1, None)
keypoints2, descriptors2 = sift.detectAndCompute(image2, None)
# 匹配特征点
bf = cv2.BFMatcher()
matches = bf.knnMatch(descriptors1, descriptors2, k=2)
# 过滤匹配结果(通常使用RANSAC)
good_matches = []
for m, n in matches:
if m.distance < 0.75 * n.distance:
good_matches.append([m])
# 计算 Essential Matrix 和 Homography Matrix
if len(good_matches) > 4:
src_pts = np.float32([keypoints1[m.queryIdx].pt for m in good_matches]).reshape(-1, 1, 2)
dst_pts = np.float32([keypoints2[m.trainIdx].pt for m in good_matches]).reshape(-1, 1, 2)
# 略去异常值
M, mask = cv2.findEssentialMat(src_pts, dst_pts, method=cv2.RANSAC, prob=0.999, threshold=1.0)
# 可能会有多解,选取其中一个
_, R, t, _ = cv2.recoverPose(M, src_pts, dst_pts, cameraMatrix=None, distCoeffs=None)
print("Relative Orientation (Rotation and Translation):")
print("Rotation matrix:\n", R)
print("Translation vector:\n", t)
else:
print("Not enough good matches found.")
阅读全文
相关推荐






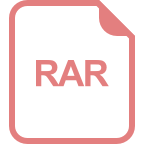



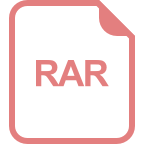






