``` # `input_ids` is a list or tensor of the input IDs and `embedding_layer` is model's embedding layer if USE_MAMBA: ```
时间: 2024-05-05 10:14:19 浏览: 130
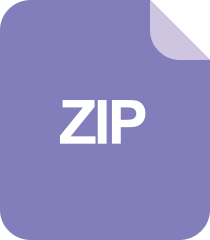
tensor_toolbox.zip_TensorToolbox_tensor_tensor toolbox _tensor_t

这段代码是一个条件语句,判断变量`USE_MAMBA`是否为真。如果`USE_MAMBA`为真,则执行下面的代码块。
在这个代码块中,`input_ids`是一个输入ID的列表或张量,`embedding_layer`是模型的嵌入层。
这段代码没有给出具体的操作,只是说明了在某种条件下会使用`input_ids`和`embedding_layer`进行一些操作。具体的操作需要在这段代码之后的代码中进行。
阅读全文
相关推荐
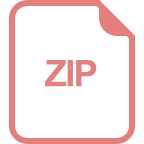
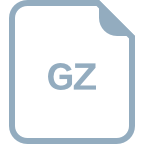
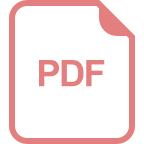
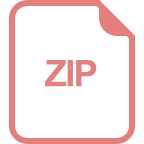
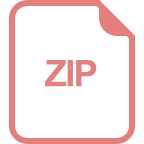
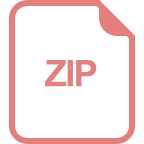
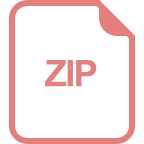
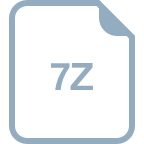
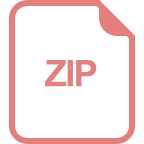
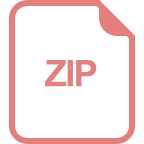
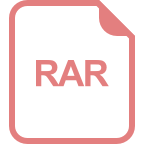
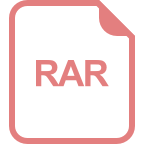
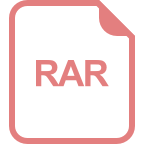




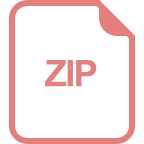