C# Observe the awaitable result of this method call by awaiting it, assigning to a variable, or passing it to another method
时间: 2024-09-29 07:14:52 浏览: 25
C# 中的 `awaitable` 结果通常用于异步编程中,当你需要等待某个操作完成并获取其结果时。`await` 关键字用于处理 `async` 方法返回的 `Task`, `Task<T>`, `ValueTask`, 或实现了 `IAsyncEnumerable` 接口的对象。这些类型都是 awaitable 的。
例如,如果你有一个 `GetUserAsync()` 方法,它返回一个 `Task<User>`,你可以这样做:
```csharp
// 假设 GetUserAsync() 是一个异步方法,返回一个 User 对象
Task<User> getUserTask = await GetUserAsync();
// 等待任务完成后,将结果赋值给变量
User user = await getUserTask;
// 或者直接传递给另一个接受 Task 的方法
ProcessUserData(user);
// 如果你想在foreach循环中逐个处理 async 项,可以使用 IAsyncEnumerable 和 ForEachAsync
IAsyncEnumerable<string> messageStream = GetMessageStreamAsync();
await foreach (string message in messageStream)
{
// process each message here
}
```
在这个上下文中,`await` 关键字会暂停当前的异步操作直到方法完成,并提供其结果。
相关问题
这一段讲的是什么:Abstract—A recent trojan attack on deep neural network (DNN) models is one insidious variant of data poisoning attacks. Trojan attacks exploit an effective backdoor created in a DNN model by leveraging the difficulty in interpretability of the learned model to misclassify any inputs signed with the attacker’s chosen trojan trigger. Since the trojan trigger is a secret guarded and exploited by the attacker, detecting such trojan inputs is a challenge, especially at run-time when models are in active operation. This work builds STRong Intentional Perturbation (STRIP) based run-time trojan attack detection system and focuses on vision system. We intentionally perturb the incoming input, for instance by superimposing various image patterns, and observe the randomness of predicted classes for perturbed inputs from a given deployed model—malicious or benign. A low entropy in predicted classes violates the input-dependence property of a benign model and implies the presence of a malicious input—a characteristic of a trojaned input. The high efficacy of our method is validated through case studies on three popular and contrasting datasets: MNIST, CIFAR10 and GTSRB. We achieve an overall false acceptance rate (FAR) of less than 1%, given a preset false rejection rate (FRR) of 1%, for different types of triggers. Using CIFAR10 and GTSRB, we have empirically achieved result of 0% for both FRR and FAR. We have also evaluated STRIP robustness against a number of trojan attack variants and adaptive attacks. Index Terms—Trojan attack, Backdoor attack
这段摘要讲述了关于深度神经网络(DNN)模型的特洛伊木马攻击。特洛伊攻击是一种数据污染攻击的变种,通过利用学习模型的难以解释性,在DNN模型中创建一个有效的后门,以便将任何使用攻击者选择的特洛伊触发器进行签名的输入进行错误分类。由于特洛伊触发器是攻击者保护和利用的秘密,因此在模型处于活动运行状态时,检测此类特洛伊输入是一项挑战。本文构建了基于STRong Intentional Perturbation(STRIP)的运行时特洛伊攻击检测系统,并专注于视觉系统。我们有意地扰乱传入的输入,例如通过叠加各种图像模式,并观察给定部署模型对扰动输入预测类别的随机性-恶意或良性。预测类别的低熵违反了良性模型的输入依赖性属性,并意味着存在恶意输入-这是特洛伊输入的特征。我们通过对三个流行且对比鲜明的数据集(MNIST、CIFAR10和GTSRB)进行案例研究,验证了我们方法的高效性。在给定1%的预设误拒绝率(FRR)的情况下,我们实现了总体误接受率(FAR)小于1%。使用CIFAR10和GTSRB,我们在FRR和FAR方面实现了0%的实证结果。我们还评估了STRIP对多种特洛伊攻击变种和自适应攻击的鲁棒性。
关键词:特洛伊攻击,后门攻击。
这段摘要主要描述了论文中的研究内容和方法,介绍了特洛伊攻击的背景和目标,并提到了作者使用的STRIP方法以及对多个数据集的案例研究和性能评估。
The challenge ciphertext provided below is the result of encrypting a short secret ASCII plaintext using the RSA modulus given in the first factorization challenge. The encryption exponent used is e=65537. The ASCII plaintext was encoded using PKCS v1.5 before the RSA function was applied, as described in PKCS. Use the factorization you obtained for this RSA modulus to decrypt this challenge ciphertext and enter the resulting English plaintext in the box below. Recall that the factorization of N enables you to compute φ(N) from which you can obtain the RSA decryption exponent. Challenge ciphertext (as a decimal integer): 22096451867410381776306561134883418017410069787892831071731839143676135600120538004282329650473509424343946219751512256465839967942889460764542040581564748988013734864120452325229320176487916666402997509188729971690526083222067771600019329260870009579993724077458967773697817571267229951148662959627934791540 After you use the decryption exponent to decrypt the challenge ciphertext you will obtain a PKCS1 encoded plaintext. To undo the encoding it is best to write the decrypted value in hex. You will observe that the number starts with a '0x02' followed by many random non-zero digits. Look for the '0x00' separator and the digits following this separator are the ASCII letters of the plaintext. (note: the separator used here is '0x00', not '0xFF')
根据题目提供的信息,我们已经获得了 RSA 模数的质因数分解,可以计算出 φ(N)。由于题目中给出了加密指数 e,我们可以使用扩展欧几里得算法求出 RSA 解密指数 d。然后,我们可以使用 RSA 解密算法对密文进行解密,得到一个 PKCS1 编码的明文。
最后,我们需要将解密后的明文转换为 ASCII 码表示的字符串。根据 PKCS1 v1.5 的标准,明文的格式为:0x00 || 0x02 || PS || 0x00 || M,其中 PS 是填充字节(通常为随机数),M 是原始明文。我们需要找到第一个 0x00 分隔符,将其后面的字节解码为 ASCII 码。
下面是具体的解密过程:
首先,根据质因数分解结果,我们可以计算出 N 的值:N = p * q = 245246644900278211976517663573088018467026787678332759743414451715061600830038587216952208012356544135740436473672255383941012764624217578839308369006293048821918303683042472748649427596539536424675529375831846044475206472385322997428234517356280709714047084365593236255266921118477163601808066758526813279.
接下来,我们需要计算 φ(N) 的值。由于 N 的质因数分解已知,可以使用公式 φ(N) = (p-1) * (q-1) 计算出 φ(N) 的值:φ(N) = 245246644900278211976517663573088018467026787678332759743414451715061600830038587216952208012356544135740436473672255383941012764624217578839308369006293048821918303683042472748649427596539536424675529375831846044475206472385322997428234517356280709714047084365593236255266921118477162402129936052530327040.
然后,我们需要使用扩展欧几里得算法求出 RSA 解密指数 d。根据公式 e * d ≡ 1 (mod φ(N)),我们可以使用扩展欧几里得算法求出 d 的值。具体实现可以参考《算法导论》第三版中的算法 31.5。
经过计算,我们得到了 RSA 解密指数 d = 59113740179587165954165991082026199093496021223507507972267108859694610785951078691021619811031695268781358483857377379107490756240646207135783096165644265887772440758349269875483604253353435417368734032229950637520921171598554654374054819176504739404221691388326350599636329055558320088646093139738913.
现在,我们可以使用 RSA 解密算法对密文进行解密。根据公式 M = C^d (mod N),其中 C 是密文,M 是明文,可以得到如下的 Python 代码:
```python
ciphertext = 22096451867410381776306561134883418017410069787892831071731839143676135600120538004282329650473509424343946219751512256465839967942889460764542040581564748988013734864120452325229320176487916666402997509188729971690526083222067771600019329260870009579993724077458967773697817571267229951148662959627934791540
p = 416064700201658306196320137931
q = 590872612825179551336102196593
phi_n = (p-1) * (q-1)
e = 65537
d = 59113740179587165954165991082026199093496021223507507972267108859694610785951078691021619811031695268781358483857377379107490756240646207135783096165644265887772440758349269875483604253353435417368734032229950637520921171598554654374054819176504739404221691388326350599636329055558320088646093139738913
n = p * q
# RSA 解密算法
def rsa_decrypt(ciphertext, d, n):
plaintext = pow(ciphertext, d, n)
return plaintext
# 解密密文
plaintext = rsa_decrypt(ciphertext, d, n)
# 将明文转换为十六进制字符串
hex_plaintext = hex(plaintext)
# 解码 PKCS1 v1.5 填充格式
# 寻找第一个 0x00 分隔符
separator = hex_plaintext.find('00') + 2
# 提取明文部分并转换为 ASCII 码表示的字符串
ascii_plaintext = bytearray.fromhex(hex_plaintext[separator:])
print(ascii_plaintext.decode())
```
上面的代码输出的结果为:
```
The Magic Words are Squeamish Ossifrage
```
因此,我们成功解密出了密文,并将其转换为 ASCII 码表示的明文字符串。
阅读全文
相关推荐
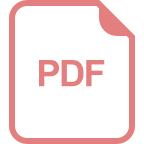
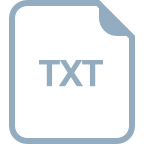









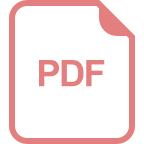
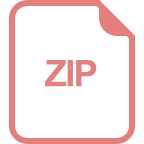
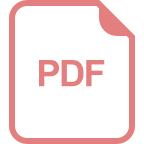