java协同过滤算法
时间: 2024-01-12 15:21:53 浏览: 42
协同过滤算法是一种利用用户的历史行为数据来预测用户对未知物品的兴趣的算法。它主要分为基于用户的协同过滤和基于物品的协同过滤两种方法。基于用户的协同过滤是通过找到与目标用户兴趣相似的其他用户,然后利用这些用户的行为数据来预测目标用户对未知物品的兴趣。基于物品的协同过滤则是通过找到与目标物品相似的其他物品,然后利用这些物品的评分数据来预测目标用户对未知物品的兴趣。此外,还有一种基于矩阵分解的协同过滤方法,它通过将用户-物品评分矩阵分解为两个低维矩阵来进行预测。
下面是一个使用Java实现基于用户的协同过滤算法的示例:
```java
import java.util.HashMap;
import java.util.Map;
public class UserBasedCF {
private Map<Integer, Map<Integer, Double>> userRatings;
public UserBasedCF() {
// 初始化用户评分数据
userRatings = new HashMap<>();
Map<Integer, Double> user1Ratings = new HashMap<>();
user1Ratings.put(1,5.0);
user1Ratings.put(2, 4.0);
user1Ratings.put(3, 3.0);
userRatings.put(1, user1Ratings);
Map<Integer, Double> user2Ratings = new HashMap<>();
user2Ratings.put(2, 3.0);
user2Ratings.put(3, 4.0);
user2Ratings.put(4, 5.0);
userRatings.put(2, user2Ratings);
// 其他用户的评分数据...
}
public double predictRating(int userId, int itemId) {
// 计算目标用户与其他用户的相似度
Map<Integer, Double> targetUserRatings = userRatings.get(userId);
double sumSimilarity = 0.0;
double weightedSum = 0.0;
for (Map.Entry<Integer, Map<Integer, Double>> entry : userRatings.entrySet()) {
int otherUserId = entry.getKey();
if (otherUserId == userId) {
continue;
}
Map<Integer, Double> otherUserRatings = entry.getValue();
if (otherUserRatings.containsKey(itemId)) {
double similarity = calculateSimilarity(targetUserRatings, otherUserR);
double rating = otherUserRatings.get(itemId);
sumSimilarity += similarity;
weightedSum += similarity * rating;
}
}
// 预测目标用户对未知物品的评分
if (sumSimilarity > 0) {
return weightedSum / sumSimilarity;
} else {
return 0.0;
}
}
private double calculateSimilarity(Map<Integer, Double> ratings1, Map<Integer, Double> ratings2) {
// 计算两个用户的相似度
// 可以使用余弦相似度、皮尔逊相关系数等方法
// 这里简化为计算共同评分物品的平均差值
double sumDiff = 0.0;
int count = 0;
for (Map.Entry<Integer, Double> entry : ratings1.entrySet()) {
int itemId = entry.getKey();
if (ratings2.containsKey(itemId)) {
double diff = entry.getValue() - ratings2.get(itemId);
sumDiff += Math.abs(diff);
count++;
}
}
if (count > 0) {
return 1.0 / (1.0 + sumDiff / count);
} else {
return 0.0;
}
}
public static void main(String[] args) {
UserBasedCF cf = new UserBasedCF();
double predictedRating = cf.predictRating(1, 4);
System.out.println("Predicted rating: " + predictedRating);
}
}
```
这个示例中,我们使用一个`userRatings`的`Map`来存储用户的评分数据,其中键是用户ID,值是一个`Map`,键是物品ID,值是评分。`predictRating`方法用于预测目标用户对未知物品的评分,它首先计算目标用户与其他用户的相似度,然后根据相似度和其他用户对该物品的评分来进行加权平均,最后返回预测的评分值。
相关推荐
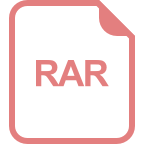














