how to apply cnn, bilstm, attention to predict one feature based on other features. Assume that I want to predict the hourly data of the target feature from 6 am to 18 pm of tomorrow, and I know the hourly data of other features for tomorrow, and I also have historical data of the target feature and other features for training the model. Then, how to apply cnn-bilstm-attention in python
时间: 2024-03-05 09:50:03 浏览: 126
To apply CNN-BiLSTM-Attention to predict one feature based on other features, you can follow these steps in Python:
1. Data Preparation: Collect the historical data of the target feature and other features, and split them into training and testing sets. You can use libraries like pandas and numpy for data manipulation.
2. Feature Engineering: Extract relevant features from the data, and normalize them to reduce the effect of outliers. You can use sklearn.preprocessing for feature scaling.
3. Model Building: Build a CNN-BiLSTM-Attention model using libraries like Keras or PyTorch. The model should take the other features as input and predict the target feature for the next 12 hours (6 am to 18 pm).
4. Model Training: Train the model on the training set, and validate it on the testing set. You can use libraries like TensorFlow or PyTorch for model training.
5. Model Evaluation: Evaluate the model performance using metrics like Mean Squared Error (MSE) or Root Mean Squared Error (RMSE). You can use libraries like sklearn.metrics for evaluation.
Here is some sample code to get started with:
```
# Data Preparation
import pandas as pd
import numpy as np
# Load data
data = pd.read_csv('data.csv')
# Split data into training and testing sets
train_data = data[:-24]
test_data = data[-24:]
# Feature Engineering
from sklearn.preprocessing import MinMaxScaler
# Extract relevant features
train_features = train_data[['feature1', 'feature2', 'feature3']].values
train_target = train_data['target'].values
test_features = test_data[['feature1', 'feature2', 'feature3']].values
test_target = test_data['target'].values
# Normalize features
scaler = MinMaxScaler()
train_features = scaler.fit_transform(train_features)
test_features = scaler.transform(test_features)
# Model Building
from keras.models import Sequential
from keras.layers import Dense, Conv1D, MaxPooling1D, LSTM, Bidirectional, Attention
# Build model
model = Sequential()
model.add(Conv1D(filters=64, kernel_size=3, activation='relu', input_shape=(train_features.shape[1], 1)))
model.add(MaxPooling1D(pool_size=2))
model.add(Bidirectional(LSTM(64, return_sequences=True)))
model.add(Attention())
model.add(Dense(1))
# Model Training
model.compile(optimizer='adam', loss='mse')
model.fit(train_features.reshape(train_features.shape[0], train_features.shape[1], 1), train_target, epochs=50, batch_size=32)
# Model Evaluation
from sklearn.metrics import mean_squared_error
# Test model
test_pred = model.predict(test_features.reshape(test_features.shape[0], test_features.shape[1], 1))
test_mse = mean_squared_error(test_target, test_pred)
print('Test MSE:', test_mse)
```
Note that this is just a sample code, and you may need to modify it based on your specific use case.
阅读全文
相关推荐
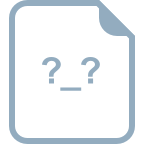
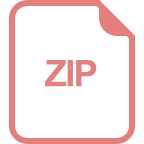
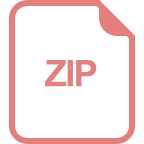
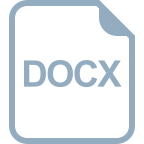
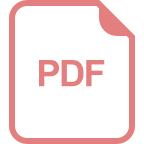
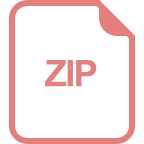
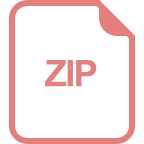
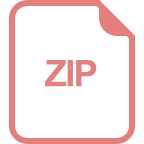
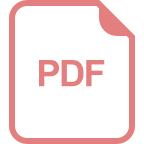
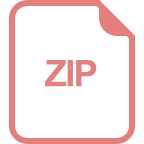
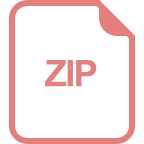
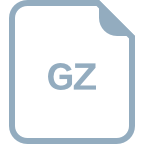
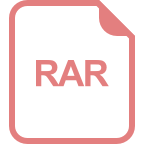
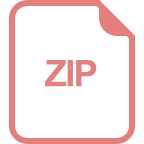
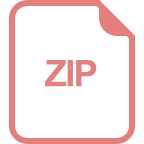
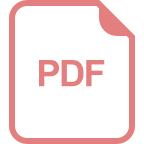
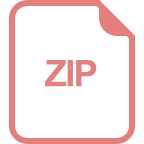
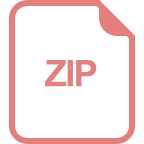