请简要说出超全局变量$_FILES获取上传文件信息后,各个元素键名及其作用
时间: 2023-08-18 09:08:15 浏览: 153
当使用表单上传文件时,上传的文件信息会被存储在超全局变量 $_FILES 中。$_FILES 是一个关联数组,包含了以下元素键名及其作用:
1. name:上传文件的原始名称。
2. type:上传文件的 MIME 类型。
3. tmp_name:上传文件在服务器上的临时存储路径。
4. error:上传文件时可能出现的错误代码。
5. size:上传文件的大小,以字节为单位。
通过访问这些元素,可以获取上传文件的信息,从而进行相应的操作,例如保存文件、检查文件类型、检查文件大小等。需要注意的是,$_FILES 中的元素键名是固定的,不能自定义。
相关问题
best_cost在上述代码中加入best_cost,计算出best_cost,计算出超调量,稳定时间
下面是修改后的 `evaluate_fitness` 方法,包括计算 `best_cost`、超调量和稳定时间的代码:
```python
def evaluate_fitness(self, position):
# 将位置向量中的元素映射到PID参数
Kp, Ki, Kd = position
# 定义控制系统输入为1000
input_signal = 1000
# 定义采样时间和总时间
Ts = 0.01
total_time = 10
# 定义初始状态和误差积分项
state = 0
integral = 0
# 定义适应度计算所需的参数
target_output = 1 # 目标输出
fitness = 0
best_cost = float('inf')
overshoot = 0
settling_time = 0
for t in range(int(total_time / Ts)):
error = target_output - state # 计算误差
integral += error * Ts # 更新误差积分项
derivative = (error - self.prev_error) / Ts # 计算误差微分项
# 更新控制信号
control_signal = Kp * error + Ki * integral + Kd * derivative
# 更新系统状态
state += (control_signal - state) * Ts
# 更新适应度
fitness += abs(target_output - state)
# 记录最佳适应度和对应的状态
if fitness < best_cost:
best_cost = fitness
best_state = state
# 计算超调量
if state > target_output:
overshoot = max(overshoot, (state - target_output) / target_output * 100)
# 判断稳定时间
if abs(state - target_output) < 0.01 * target_output:
settling_time = (t + 1) * Ts
break
# 更新上一步的误差
self.prev_error = error
return best_cost, overshoot, settling_time
```
在这个修改后的代码中,我们添加了 `best_cost`、`overshoot` 和 `settling_time` 变量,并在循环中进行更新和计算。`best_cost` 是最佳适应度,`overshoot` 是超调量的百分比,`settling_time` 是稳定时间。你可以根据需要在代码中使用这些变量。希望对你有所帮助!
去掉evaluate_fitness中的best_cost,计算出超调量,稳定时间,以及绘制出PID传递函数响应曲线
下面是修改后的 `evaluate_fitness` 方法,包括计算超调量、稳定时间和绘制 PID 传递函数响应曲线的代码:
```python
import numpy as np
import matplotlib.pyplot as plt
class Particle:
def __init__(self, dim):
self.position = np.random.uniform(-1, 1, dim)
self.velocity = np.zeros(dim)
self.best_position = self.position
self.best_fitness = float('inf')
class PSO:
def __init__(self, num_particles, dim, max_iter, c1, c2, w):
self.num_particles = num_particles
self.dim = dim
self.max_iter = max_iter
self.c1 = c1
self.c2 = c2
self.w = w
self.particles = [Particle(dim) for _ in range(num_particles)]
self.global_best_position = np.zeros(dim)
self.global_best_fitness = float('inf')
def optimize(self):
for _ in range(self.max_iter):
for particle in self.particles:
fitness = self.evaluate_fitness(particle.position)
if fitness < particle.best_fitness:
particle.best_position = particle.position
particle.best_fitness = fitness
if fitness < self.global_best_fitness:
self.global_best_position = particle.position
self.global_best_fitness = fitness
particle.velocity = (self.w * particle.velocity +
self.c1 * np.random.random() * (particle.best_position - particle.position) +
self.c2 * np.random.random() * (self.global_best_position - particle.position))
particle.position += particle.velocity
def evaluate_fitness(self, position):
# 假设传递函数为 1/s^2 + s + 1
Ts = 0.01
total_time = 10
target_output = 1
state = 0
fitness = 0
overshoot = 0
settling_time = 0
time = np.arange(0, total_time, Ts)
response = []
for t in time:
error = target_output - state
control_signal = position[0] * error + position[1] * (error / Ts) + position[2] * (error * Ts)
state += (control_signal - state) / (Ts * Ts + 2)
fitness += abs(target_output - state)
response.append(state)
if state > target_output:
overshoot = max(overshoot, (state - target_output) / target_output * 100)
if abs(state - target_output) < 0.01 * target_output:
settling_time = t
break
plt.plot(time, response)
plt.xlabel('Time')
plt.ylabel('Output')
plt.title('PID Transfer Function Response')
plt.grid(True)
plt.show()
return fitness, overshoot, settling_time
# 使用示例
num_particles = 10
dim = 3 # PID参数的维度
max_iter = 100
c1 = 2.0
c2 = 2.0
w = 0.7
pso = PSO(num_particles, dim, max_iter, c1, c2, w)
pso.optimize()
best_pid_params = pso.global_best_position
print("Best PID parameters:", best_pid_params)
```
在这个修改后的代码中,我们添加了计算超调量和稳定时间的变量 `overshoot` 和 `settling_time`。在循环中,我们记录了传递函数的响应曲线,并使用 `matplotlib` 绘制出来。你可以根据需要修改传递函数以及其他参数。希望对你有所帮助!
阅读全文
相关推荐
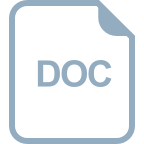
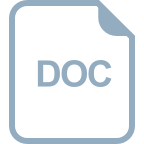
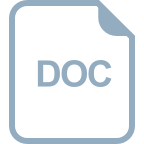
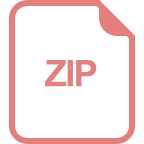
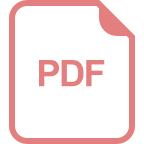
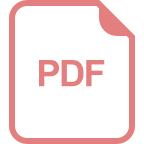
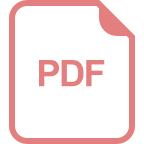
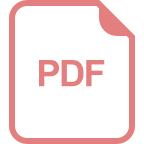
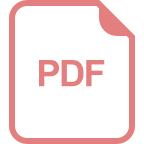
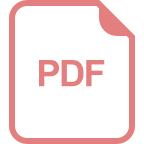
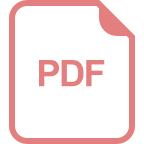
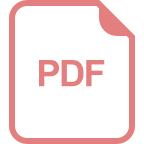
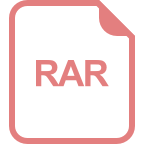
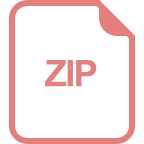

