Tansformer翻译模型
时间: 2024-01-18 15:02:02 浏览: 118
Transformer翻译模型是一种基于深度学习的神经机器翻译模型,它是由Google在2017年提出的。相较于传统的循环神经网络(RNN)翻译模型,Transformer模型使用了自注意力机制(self-attention),能够更好地捕捉长距离依赖关系,从而提升翻译质量。
Transformer模型的核心是多头自注意力机制(Multi-Head Attention)。在翻译任务中,输入的源语言句子和目标语言句子都被表示成一个由词向量组成的向量序列。在多头自注意力机制中,每个词向量都会与所有其他词向量进行相似度计算,从而得到一个权重向量。这个权重向量体现了每个词向量在整个句子中的重要性。然后,将每个词向量按照其对应的权重向量进行加权求和,得到整个句子的表示。
除了多头自注意力机制,Transformer模型还使用了残差连接和层归一化等技术,从而加速训练和提高模型的鲁棒性。
总之,Transformer模型是一种非常先进的神经机器翻译模型,具有较好的翻译质量和较高的效率。
相关问题
Tansformer翻译模型代码
以下是基于TensorFlow的Transformer翻译模型代码:
```
import tensorflow as tf
import numpy as np
class MultiHeadAttention(tf.keras.layers.Layer):
def __init__(self, d_model, num_heads):
super(MultiHeadAttention, self).__init__()
self.num_heads = num_heads
self.d_model = d_model
assert d_model % self.num_heads == 0
self.depth = d_model // self.num_heads
self.Wq = tf.keras.layers.Dense(d_model)
self.Wk = tf.keras.layers.Dense(d_model)
self.Wv = tf.keras.layers.Dense(d_model)
self.dense = tf.keras.layers.Dense(d_model)
def split_heads(self, x, batch_size):
x = tf.reshape(x, (batch_size, -1, self.num_heads, self.depth))
return tf.transpose(x, perm=[0, 2, 1, 3])
def call(self, q, k, v, mask):
batch_size = tf.shape(q)[0]
q = self.Wq(q)
k = self.Wk(k)
v = self.Wv(v)
q = self.split_heads(q, batch_size)
k = self.split_heads(k, batch_size)
v = self.split_heads(v, batch_size)
scaled_attention, attention_weights = self.scaled_dot_product_attention(q, k, v, mask)
scaled_attention = tf.transpose(scaled_attention, perm=[0, 2, 1, 3])
concat_attention = tf.reshape(scaled_attention, (batch_size, -1, self.d_model))
output = self.dense(concat_attention)
return output, attention_weights
def scaled_dot_product_attention(self, q, k, v, mask):
matmul_qk = tf.matmul(q, k, transpose_b=True)
depth = tf.cast(tf.shape(k)[-1], tf.float32)
logits = matmul_qk / tf.math.sqrt(depth)
if mask is not None:
logits += (mask * -1e9)
attention_weights = tf.nn.softmax(logits, axis=-1)
output = tf.matmul(attention_weights, v)
return output, attention_weights
def point_wise_feed_forward_network(d_model, dff):
return tf.keras.Sequential([
tf.keras.layers.Dense(dff, activation='relu'),
tf.keras.layers.Dense(d_model)
])
class EncoderLayer(tf.keras.layers.Layer):
def __init__(self, d_model, num_heads, dff, rate=0.1):
super(EncoderLayer, self).__init__()
self.mha = MultiHeadAttention(d_model, num_heads)
self.ffn = point_wise_feed_forward_network(d_model, dff)
self.layernorm1 = tf.keras.layers.LayerNormalization(epsilon=1e-6)
self.layernorm2 = tf.keras.layers.LayerNormalization(epsilon=1e-6)
self.dropout1 = tf.keras.layers.Dropout(rate)
self.dropout2 = tf.keras.layers.Dropout(rate)
def call(self, x, training, mask):
attn_output, _ = self.mha(x, x, x, mask)
attn_output = self.dropout1(attn_output, training=training)
out1 = self.layernorm1(x + attn_output)
ffn_output = self.ffn(out1)
ffn_output = self.dropout2(ffn_output, training=training)
out2 = self.layernorm2(out1 + ffn_output)
return out2
class DecoderLayer(tf.keras.layers.Layer):
def __init__(self, d_model, num_heads, dff, rate=0.1):
super(DecoderLayer, self).__init__()
self.mha1 = MultiHeadAttention(d_model, num_heads)
self.mha2 = MultiHeadAttention(d_model, num_heads)
self.ffn = point_wise_feed_forward_network(d_model, dff)
self.layernorm1 = tf.keras.layers.LayerNormalization(epsilon=1e-6)
self.layernorm2 = tf.keras.layers.LayerNormalization(epsilon=1e-6)
self.layernorm3 = tf.keras.layers.LayerNormalization(epsilon=1e-6)
self.dropout1 = tf.keras.layers.Dropout(rate)
self.dropout2 = tf.keras.layers.Dropout(rate)
self.dropout3 = tf.keras.layers.Dropout(rate)
def call(self, x, enc_output, training, look_ahead_mask, padding_mask):
attn1, attn_weights_block1 = self.mha1(x, x, x, look_ahead_mask)
attn1 = self.dropout1(attn1, training=training)
out1 = self.layernorm1(attn1 + x)
attn2, attn_weights_block2 = self.mha2(enc_output, enc_output, out1, padding_mask)
attn2 = self.dropout2(attn2, training=training)
out2 = self.layernorm2(attn2 + out1)
ffn_output = self.ffn(out2)
ffn_output = self.dropout3(ffn_output, training=training)
out3 = self.layernorm3(ffn_output + out2)
return out3, attn_weights_block1, attn_weights_block2
class Encoder(tf.keras.layers.Layer):
def __init__(self, num_layers, d_model, num_heads, dff, input_vocab_size,
maximum_position_encoding, rate=0.1):
super(Encoder, self).__init__()
self.d_model = d_model
self.num_layers = num_layers
self.embedding = tf.keras.layers.Embedding(input_vocab_size, d_model)
self.pos_encoding = positional_encoding(maximum_position_encoding, self.d_model)
self.enc_layers = [EncoderLayer(d_model, num_heads, dff, rate)
for _ in range(num_layers)]
self.dropout = tf.keras.layers.Dropout(rate)
def call(self, x, training, mask):
seq_len = tf.shape(x)[1]
x = self.embedding(x)
x *= tf.math.sqrt(tf.cast(self.d_model, tf.float32))
x += self.pos_encoding[:, :seq_len, :]
x = self.dropout(x, training=training)
for i in range(self.num_layers):
x = self.enc_layers[i](x, training, mask)
return x
class Decoder(tf.keras.layers.Layer):
def __init__(self, num_layers, d_model, num_heads, dff, target_vocab_size,
maximum_position_encoding, rate=0.1):
super(Decoder, self).__init__()
self.d_model = d_model
self.num_layers = num_layers
self.embedding = tf.keras.layers.Embedding(target_vocab_size, d_model)
self.pos_encoding = positional_encoding(maximum_position_encoding, d_model)
self.dec_layers = [DecoderLayer(d_model, num_heads, dff, rate)
for _ in range(num_layers)]
self.dropout = tf.keras.layers.Dropout(rate)
def call(self, x, enc_output, training,
look_ahead_mask, padding_mask):
seq_len = tf.shape(x)[1]
attention_weights = {}
x = self.embedding(x)
x *= tf.math.sqrt(tf.cast(self.d_model, tf.float32))
x += self.pos_encoding[:, :seq_len, :]
x = self.dropout(x, training=training)
for i in range(self.num_layers):
x, block1, block2 = self.dec_layers[i](x, enc_output, training,
look_ahead_mask, padding_mask)
attention_weights[f'decoder_layer{i + 1}_block1'] = block1
attention_weights[f'decoder_layer{i + 1}_block2'] = block2
return x, attention_weights
def positional_encoding(position, d_model):
angle_rates = 1 / np.power(10000, (2 * (np.arange(d_model)[np.newaxis, :] // 2)) / np.float32(d_model))
angle_rads = np.arange(position)[:, np.newaxis] * angle_rates
sines = np.sin(angle_rads[:, 0::2])
cosines = np.cos(angle_rads[:, 1::2])
pos_encoding = np.concatenate([sines, cosines], axis=-1)
pos_encoding = pos_encoding[np.newaxis, ...]
return tf.cast(pos_encoding, dtype=tf.float32)
class Transformer(tf.keras.Model):
def __init__(self, num_layers, d_model, num_heads, dff, input_vocab_size,
target_vocab_size, pe_input, pe_target, rate=0.1):
super(Transformer, self).__init__()
self.encoder = Encoder(num_layers, d_model, num_heads, dff,
input_vocab_size, pe_input, rate)
self.decoder = Decoder(num_layers, d_model, num_heads, dff,
target_vocab_size, pe_target, rate)
self.final_layer = tf.keras.layers.Dense(target_vocab_size)
def call(self, inp, tar, training, enc_padding_mask,
look_ahead_mask, dec_padding_mask):
enc_output = self.encoder(inp, training, enc_padding_mask)
dec_output, attention_weights = self.decoder(
tar, enc_output, training, look_ahead_mask, dec_padding_mask)
final_output = self.final_layer(dec_output)
return final_output, attention_weights
```
这里实现了一个基本的Transformer模型结构,包括Encoder和Decoder。其中,MultiHeadAttention用于实现多头注意力机制,EncoderLayer和DecoderLayer分别为编码器和解码器层,Encoder和Decoder则分别为编码器和解码器,positional_encoding用于对输入进行位置编码。完整的模型结构包括输入、输出、损失函数等封装在一个Transformer类中。
tansformer模型
Transformer模型是一种用于自然语言处理任务的深度学习模型,由Google在2017年提出。与传统的循环神经网络(RNN)不同,Transformer模型使用了注意力机制(Attention)来实现输入与输出之间的对齐,从而避免了RNN中的梯度消失问题。Transformer模型在机器翻译、语言模型、文本分类等任务中均表现出色,成为自然语言处理领域中的重要模型之一。
阅读全文
相关推荐
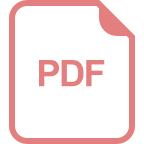
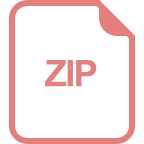
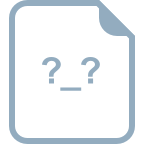




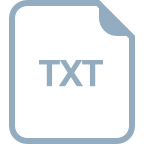
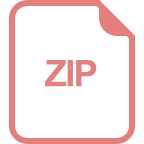
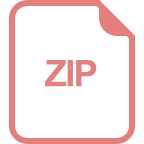
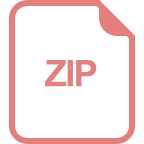
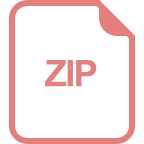
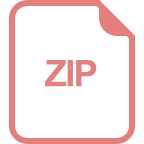
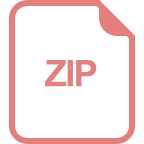