任何实现临床-影像-深度学习模型的叠加模型(stacking0
时间: 2024-05-19 19:15:51 浏览: 87
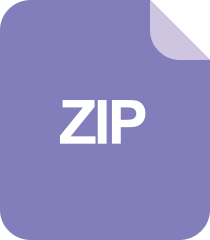
medical:模型融合 stacking
1. Stacking with Single Classifier: In this approach, a single classifier is trained on the original data and the predictions made by this classifier are then used as inputs for a second classifier. This process is repeated for multiple classifiers, and the final prediction is made by combining the outputs of all classifiers.
2. Stacking with Multiple Classifiers: In this approach, multiple classifiers are trained on the original data, and the predictions made by these classifiers are combined using a meta-classifier. The meta-classifier is trained on the outputs of the base classifiers, and the final prediction is made by the meta-classifier.
3. Stacking with Feature Engineering: In this approach, feature engineering is performed on the original data, and the engineered features are used as inputs for the base classifiers. The outputs of the base classifiers are then combined using a meta-classifier, and the final prediction is made by the meta-classifier.
4. Stacking with Data Augmentation: In this approach, data augmentation techniques are used to increase the size of the training dataset. The augmented data is then used to train the base classifiers, and the outputs of the base classifiers are combined using a meta-classifier.
5. Stacking with Transfer Learning: In this approach, transfer learning techniques are used to transfer knowledge from pre-trained models to the base classifiers. The outputs of the base classifiers are then combined using a meta-classifier to make the final prediction.
阅读全文
相关推荐
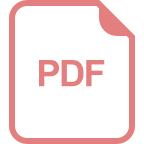
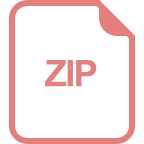
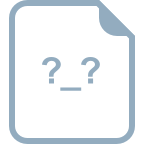
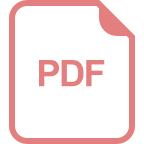
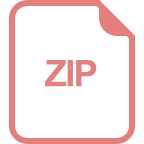
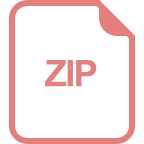
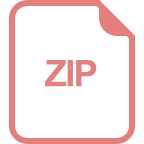
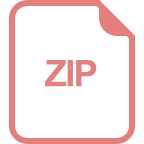
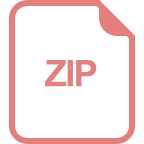
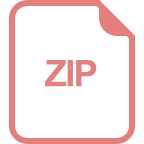
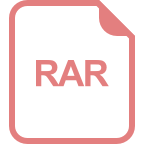
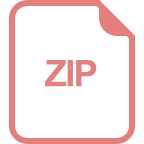
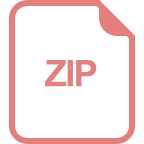
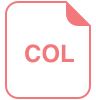
