pytorch训练两个数字的mnist数据集
时间: 2023-09-29 12:03:32 浏览: 81
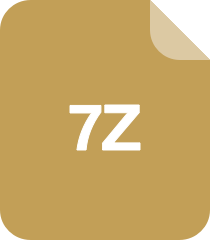
《实战2-pytorch训练MNIST网络,tensorflow预测》对应的资源和脚本
可以使用以下代码来训练两个数字的mnist数据集:
```python
import torch
import torch.nn as nn
import torch.optim as optim
from torchvision import datasets, transforms
# Define the two digits to train on
digit1 = 3
digit2 = 7
# Define the transformation to be applied to the data
transform = transforms.Compose([
transforms.ToTensor(),
transforms.Normalize((0.1307,), (0.3081,))
])
# Load the MNIST dataset
train_dataset = datasets.MNIST('data', train=True, download=True, transform=transform)
# Filter the dataset to only include the two digits of interest
train_dataset = torch.utils.data.Subset(train_dataset, torch.where((train_dataset.targets == digit1) | (train_dataset.targets == digit2))[0])
# Define the model to be used
class Net(nn.Module):
def __init__(self):
super(Net, self).__init__()
self.conv1 = nn.Conv2d(1, 10, kernel_size=5)
self.conv2 = nn.Conv2d(10, 20, kernel_size=5)
self.dropout = nn.Dropout2d()
self.fc1 = nn.Linear(320, 50)
self.fc2 = nn.Linear(50, 2)
def forward(self, x):
x = nn.functional.relu(nn.functional.max_pool2d(self.conv1(x), 2))
x = nn.functional.relu(nn.functional.max_pool2d(self.dropout(self.conv2(x)), 2))
x = x.view(-1, 320)
x = nn.functional.relu(self.fc1(x))
x = self.fc2(x)
return nn.functional.log_softmax(x, dim=1)
model = Net()
# Define the optimizer and loss function
optimizer = optim.SGD(model.parameters(), lr=0.01, momentum=0.5)
criterion = nn.CrossEntropyLoss()
# Train the model
model.train()
for epoch in range(5):
for batch_idx, (data, target) in enumerate(train_loader):
optimizer.zero_grad()
output = model(data)
loss = criterion(output, target)
loss.backward()
optimizer.step()
if batch_idx % 100 == 0:
print('Train Epoch: {} [{}/{} ({:.0f}%)]\tLoss: {:.6f}'.format(
epoch, batch_idx * len(data), len(train_loader.dataset),
100. * batch_idx / len(train_loader), loss.item()))
```
请问您还有其他问题吗?
阅读全文
相关推荐
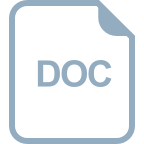
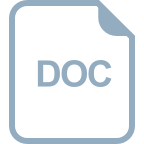
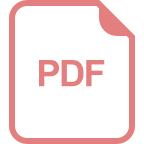
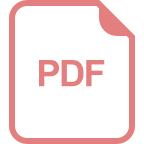


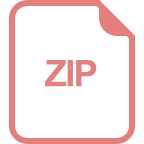
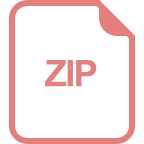
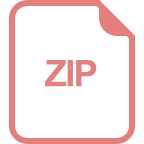
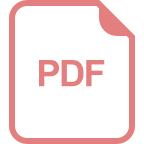
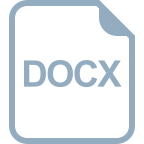
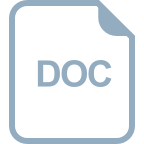
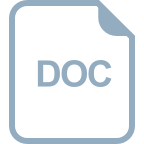




