如何确认我的PyTorch版本是否支持`torch.distributed`?
时间: 2024-10-07 08:02:20 浏览: 22
在Python中,你可以通过以下步骤检查你的PyTorch版本是否包含`torch.distributed`模块:
1. 首先,打开命令行终端或者Python交互环境(如Jupyter Notebook)。
2. 然后导入PyTorch库并查看其版本信息。输入以下代码:
```python
import torch
print(torch.__version__)
```
3. 如果`torch`库版本大于等于1.0.0,因为`torch.distributed`是在PyTorch 1.0版本中引入的,那么你应该就能使用分布式功能。你可以在命令结果中查找"1.x.x"这样的格式,其中"x.x.x"代表各个小版本号。
4. 接下来,可以尝试导入`torch.distributed`模块,看看是否能成功导入而不会报错。如果能成功导入,则说明你的版本支持该模块:
```python
try:
import torch.distributed as dist
print("Your PyTorch version supports torch.distributed")
except ImportError:
print("Your PyTorch version does not support torch.distributed or it's not installed.")
```
相关问题
具体怎么使用torch.distributed.launch?
### 回答1:
torch.distributed.launch是PyTorch的一个工具,可以用来启动分布式训练任务。具体使用方法如下:
首先,在你的代码中使用torch.distributed模块来定义分布式训练的参数,如下所示:
```
import torch.distributed as dist
dist.init_process_group(backend="nccl", init_method="env://")
```
这个代码片段定义了使用NCCL作为分布式后端,以及使用环境变量作为初始化方法。
接下来,在命令行中使用torch.distributed.launch来启动分布式训练任务,如下所示:
```
python -m torch.distributed.launch --nproc_per_node=NUM_GPUS YOUR_TRAINING_SCRIPT.py (--arg1 --arg2 --arg3 and so on)
```
其中,NUM_GPUS是每个节点上使用的GPU数量,YOUR_TRAINING_SCRIPT.py是你的训练脚本,(--arg1 --arg2 --arg3 and so on)是传递给训练脚本的参数。
torch.distributed.launch会自动为每个节点启动一个进程,并传递适当的环境变量和命令行参数。在训练过程中,你可以使用torch.distributed模块来进行分布式的操作,如在每个节点之间同步参数、收集梯度等。
希望这个回答对你有所帮助!
### 回答2:
torch.distributed.launch是PyTorch中用于多节点分布式训练的一个工具。它能够帮助我们简化在多个节点上启动分布式训练的过程,使得代码编写更加简单方便。
使用torch.distributed.launch,首先需要确保环境中已经安装了PyTorch库。然后,在命令行中执行以下命令:
python -m torch.distributed.launch --nproc_per_node=<num_gpus> <your_script.py> (--arg1 --arg2 ...)
其中,"<num_gpus>"是每个节点上的GPU数量,"<your_script.py>"是要运行的脚本路径。"--arg1 --arg2 ..."是你的脚本所需的各种参数,与普通的命令行参数传递方式相同。
执行上述命令后,torch.distributed.launch将会自动在每个节点上启动训练进程,并负责进程间的通信和同步。每个进程将会自动获得一个本地的rank编号,从0开始递增,并且可以通过torch.distributed.get_rank()函数获得。
在你的训练脚本中,可以通过torch.distributed.get_world_size()获得总的节点数量,通过torch.distributed.get_rank()获得当前节点的rank编号。你可以根据这些信息来区分不同的节点,进行相应的分布式操作。
除了以上基本用法外,torch.distributed.launch还提供了其他的一些选项,如--use_env、--master_addr、--master_port等,可以根据需要进行使用。可以通过在命令行中执行python -m torch.distributed.launch --help来查看更多详细的帮助信息。
总之,使用torch.distributed.launch可以方便地实现多节点分布式训练,简化了代码编写和启动的过程,提高了训练效率和灵活性。
torch.distributed.
torch.distributed是PyTorch中用于分布式训练的模块。它提供了一组用于在多个进程之间进行通信和同步的函数,以便在分布式环境中进行模型训练。其中包括上述三个函数:torch.distributed.barrier、torch.distributed.send和torch.distributed.isend。
1. torch.distributed.barrier函数用于在分布式环境中同步多个进程。当一个进程调用该函数时,它会等待所有其他进程也调用该函数,然后所有进程才会继续执行。
2. torch.distributed.send函数用于将张量发送到指定的进程。它需要指定要发送的张量、目标进程的ID和可选的标记。
3. torch.distributed.isend函数与torch.distributed.send函数类似,但它是异步的,即它不会等待接收进程接收数据。相反,它会立即返回一个请求对象,该对象可以用于检查发送是否完成或等待发送完成。
相关推荐
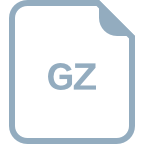
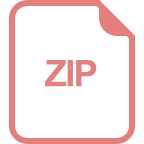
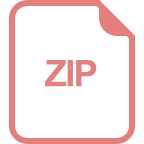













