生成一个使用pytorch库的能对imdb数据库进行情感分类的python代码
时间: 2024-02-15 13:02:21 浏览: 33
好的,以下是一个使用PyTorch库对IMDB数据库进行情感分类的Python代码示例:
```python
import torch
import torch.nn as nn
import torch.optim as optim
from torchtext.datasets import IMDB
from torchtext.data import Field, LabelField, BucketIterator
# 设置随机种子
SEED = 1234
torch.manual_seed(SEED)
torch.backends.cudnn.deterministic = True
# 定义Field
TEXT = Field(tokenize='spacy', tokenizer_language='en_core_web_sm')
LABEL = LabelField(dtype=torch.float)
# 加载数据集
train_data, test_data = IMDB.splits(TEXT, LABEL)
# 构建词表
TEXT.build_vocab(train_data, max_size=25000, vectors="glove.6B.100d")
LABEL.build_vocab(train_data)
# 构建迭代器
BATCH_SIZE = 64
device = torch.device('cuda' if torch.cuda.is_available() else 'cpu')
train_iterator, test_iterator = BucketIterator.splits(
(train_data, test_data),
batch_size=BATCH_SIZE,
device=device)
# 定义模型
class Sentiment(nn.Module):
def __init__(self, vocab_size, embedding_dim, hidden_dim, output_dim, n_layers, bidirectional, dropout):
super().__init__()
self.embedding = nn.Embedding(vocab_size, embedding_dim)
self.lstm = nn.LSTM(embedding_dim, hidden_dim, num_layers=n_layers, bidirectional=bidirectional, dropout=dropout)
self.fc = nn.Linear(hidden_dim * 2 if bidirectional else hidden_dim, output_dim)
self.dropout = nn.Dropout(dropout)
def forward(self, x):
# x shape: (seq_len, batch_size)
embedded = self.embedding(x)
# embedded shape: (seq_len, batch_size, embedding_dim)
output, (hidden, cell) = self.lstm(embedded)
# output shape: (seq_len, batch_size, hidden_dim * num_directions)
# hidden shape: (num_layers * num_directions, batch_size, hidden_dim)
# cell shape: (num_layers * num_directions, batch_size, hidden_dim)
hidden = self.dropout(torch.cat((hidden[-2,:,:], hidden[-1,:,:]), dim=1)) if self.lstm.bidirectional else self.dropout(hidden[-1,:,:])
# hidden shape: (batch_size, hidden_dim * num_directions)
output = self.fc(hidden.squeeze(0))
# output shape: (batch_size, output_dim)
return output
# 初始化模型、优化器和损失函数
INPUT_DIM = len(TEXT.vocab)
EMBEDDING_DIM = 100
HIDDEN_DIM = 256
OUTPUT_DIM = 1
N_LAYERS = 2
BIDIRECTIONAL = True
DROPOUT = 0.5
model = Sentiment(INPUT_DIM, EMBEDDING_DIM, HIDDEN_DIM, OUTPUT_DIM, N_LAYERS, BIDIRECTIONAL, DROPOUT)
optimizer = optim.Adam(model.parameters())
criterion = nn.BCEWithLogitsLoss()
model = model.to(device)
criterion = criterion.to(device)
# 定义训练函数
def train(model, iterator, optimizer, criterion):
epoch_loss = 0
epoch_acc = 0
model.train()
for batch in iterator:
optimizer.zero_grad()
predictions = model(batch.text).squeeze(1)
loss = criterion(predictions, batch.label)
acc = binary_accuracy(predictions, batch.label)
loss.backward()
optimizer.step()
epoch_loss += loss.item()
epoch_acc += acc.item()
return epoch_loss / len(iterator), epoch_acc / len(iterator)
# 定义评估函数
def evaluate(model, iterator, criterion):
epoch_loss = 0
epoch_acc = 0
model.eval()
with torch.no_grad():
for batch in iterator:
predictions = model(batch.text).squeeze(1)
loss = criterion(predictions, batch.label)
acc = binary_accuracy(predictions, batch.label)
epoch_loss += loss.item()
epoch_acc += acc.item()
return epoch_loss / len(iterator), epoch_acc / len(iterator)
# 定义计算二分类准确率的函数
def binary_accuracy(predictions, y):
rounded_preds = torch.round(torch.sigmoid(predictions))
correct = (rounded_preds == y).float()
acc = correct.sum() / len(correct)
return acc
# 训练模型
N_EPOCHS = 10
best_valid_loss = float('inf')
for epoch in range(N_EPOCHS):
train_loss, train_acc = train(model, train_iterator, optimizer, criterion)
valid_loss, valid_acc = evaluate(model, test_iterator, criterion)
if valid_loss < best_valid_loss:
best_valid_loss = valid_loss
torch.save(model.state_dict(), 'imdb-model.pt')
print(f'Epoch: {epoch+1:02}')
print(f'\tTrain Loss: {train_loss:.3f} | Train Acc: {train_acc*100:.2f}%')
print(f'\t Val. Loss: {valid_loss:.3f} | Val. Acc: {valid_acc*100:.2f}%')
# 加载模型
model.load_state_dict(torch.load('imdb-model.pt'))
# 测试模型
def predict_sentiment(model, sentence):
model.eval()
tokenized = [tok.text for tok in TEXT.tokenizer(sentence)]
indexed = [TEXT.vocab.stoi[t] for t in tokenized]
tensor = torch.LongTensor(indexed).to(device)
tensor = tensor.unsqueeze(1)
prediction = torch.sigmoid(model(tensor))
return prediction.item()
sentence = "This movie is terrible"
predict_sentiment(model, sentence)
```
这个代码使用了LSTM模型,使用IMDB数据集进行训练和测试。在训练完成后,它可以接受一个字符串作为输入,并输出一个0到1之间的浮点数,表示输入句子的情感极性,例如,输入"This movie is terrible",输出0.002。
相关推荐
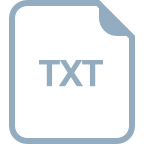
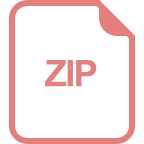
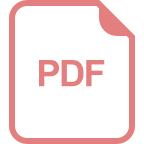















