修改以下代码使其正常运行“f = io.imread("C\\Users\\56454\\Desktop\\课程\\生物图像处理\\编程2\\cell_nucleus.tif")
时间: 2024-04-21 18:21:11 浏览: 61
要使代码正常运行,需要对文件路径进行修改。在Windows系统中,文件路径中的反斜杠`\`需要使用双反斜杠`\\`进行转义。同时,建议使用原始字符串(raw string)来表示文件路径,以避免转义字符的干扰。
修改后的代码如下所示:
```python
f = io.imread(r"C:\Users\56454\Desktop\课程\生物图像处理\编程2\cell_nucleus.tif")
```
请注意,修改后的代码中,文件路径中的反斜杠`\`被替换为双反斜杠`\\`,并且整个文件路径被包裹在原始字符串前缀`r`中。
相关问题
from scipy import ndimage from skimage import io from matplotlib import pyplot as plt # img为原始图像 img = io.imread('C:/Users/lvxiong/Desktop/3.jpg') # 中值滤波 n = 3 new_img = ndimage.median_filter(img, (n, n)) # 显示图像 plt.rcParams['font.sans-serif'] = ['SimHei'] plt.rcParams['axes.unicode_minus'] = False plt.subplot(1, 2, 1) plt.axis('off') plt.imshow(img) plt.title('原图像') plt.subplot(1, 2, 2) plt.axis('off') plt.imshow(new_img) plt.title('中值滤波') plt.savefig('中值滤波结果.tif') plt.show()
这段代码是使用 Python 中的 Scipy 和 Skimage 库实现对图像进行中值滤波的操作,并将滤波后的图像结果进行显示和保存。
具体的实现步骤如下:
1. 导入所需要的库:Scipy 中的 ndimage 模块用于图像处理,Skimage 中的 io 模块用于图像读取,Matplotlib 中的 pyplot 模块用于图像显示和保存。
2. 读取原始图像:使用 io.imread 函数读取指定路径下的图片文件,得到一个表示图像的数组 img。
3. 中值滤波处理:使用 ndimage.median_filter 函数对原始图像进行中值滤波,得到一个新的滤波后的图像数组 new_img。
4. 显示图像结果:使用 pyplot.subplot 函数创建一个包含两个子图的画布,分别用于显示原始图像和滤波后的图像。使用 pyplot.imshow 函数显示图像,并设置标题和标签等属性。最后使用 pyplot.savefig 函数保存滤波后的图像结果,并使用 pyplot.show 函数显示画布。
中值滤波是一种图像处理中常用的滤波方法,它可以有效地去除图像中的噪点,提高图像的质量。
import cv2 import os import numpy as np from sklearn import svm import joblib def read_images(folder): images = [] labels = [] for filename in os.listdir(folder): label = filename.split('.')[0] img = cv2.imread(os.path.join(folder,filename)) if img is not None: images.append(img) labels.append(label) return images, labels # 提取特征向量 def extract_features(images): features = [] for img in images: gray = cv2.cvtColor(img, cv2.COLOR_BGR2GRAY) hist = cv2.calcHist([gray],[0],None,[256],[0,256]) features.append(hist.flatten()) return features # 读取图像和标签 images, labels = read_images('C:/Users/Administrator/Desktop/111') # 提取特征向量 features = extract_features(images) # 训练模型 clf = svm.SVC() clf.fit(features, labels) # 保存模型到文件 joblib.dump(clf, 'model.pkl') clf = joblib.load('E:/xiangmu/measure/model.pkl') print(clf) # 预测新图像 img = cv2.imread('C:/Users/Administrator/Desktop/dc9cd0a3a572635fb27973627f514dfb.jpeg') gray = cv2.cvtColor(img, cv2.COLOR_BGR2GRAY) hist = cv2.calcHist([gray],[0],None,[256],[0,256]) features = np.array(hist.flatten()).reshape(1, -1) print(hist) label = clf.predict(features)[0] print('识别结果:', label) 帮我优化代码,可以打印概率
好的,我们可以使用`predict_proba`方法来获取预测结果的概率。以下是优化后的代码示例:
```python
import cv2
import os
import numpy as np
from sklearn import svm
from sklearn.metrics import accuracy_score
from sklearn.model_selection import train_test_split
from skimage.io import imread
from skimage.transform import resize
import joblib
# 读取图像和标签
def read_images(folder):
images = []
labels = []
for filename in os.listdir(folder):
label = filename.split('.')[0]
img = cv2.imread(os.path.join(folder,filename))
if img is not None:
images.append(img)
labels.append(label)
return images, labels
# 提取特征向量
def extract_features(images):
features = []
for img in images:
gray = cv2.cvtColor(img, cv2.COLOR_BGR2GRAY)
hist = cv2.calcHist([gray],[0],None,[256],[0,256])
features.append(hist.flatten())
return features
# 读取图像和标签
images, labels = read_images('C:/Users/Administrator/Desktop/111')
# 提取特征向量
features = extract_features(images)
# 将数据集分为训练集和测试集
X_train, X_test, y_train, y_test = train_test_split(features, labels, test_size=0.3)
# 创建SVM分类器
clf = svm.SVC(kernel='linear', probability=True)
# 训练分类器
clf.fit(X_train, y_train)
# 保存模型到文件
joblib.dump(clf, 'model.pkl')
# 加载模型
clf = joblib.load('model.pkl')
# 预测新图像
img = cv2.imread('C:/Users/Administrator/Desktop/dc9cd0a3a572635fb27973627f514dfb.jpeg')
gray = cv2.cvtColor(img, cv2.COLOR_BGR2GRAY)
hist = cv2.calcHist([gray],[0],None,[256],[0,256])
features = np.array(hist.flatten()).reshape(1, -1)
# 预测分类和概率
label = clf.predict(features)[0]
prob = clf.predict_proba(features)[0]
print('识别结果:', label)
print('概率分布:', prob)
```
在这个示例中,我们首先将数据集分为训练集和测试集,然后创建SVM分类器并训练它。我们设置`probability=True`来启用预测结果的概率估计。在预测新图像时,我们使用`predict`方法获取预测分类,使用`predict_proba`方法获取预测结果的概率分布。最后,我们打印预测结果和概率分布。
阅读全文
相关推荐
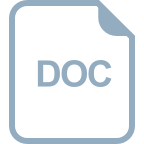
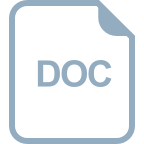
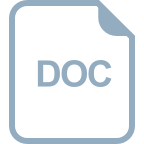


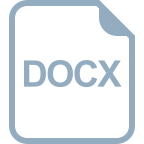
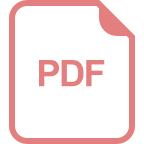

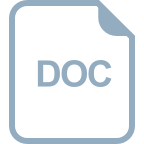
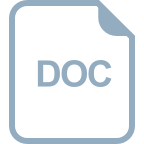
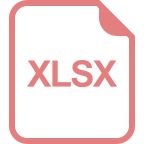
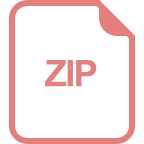
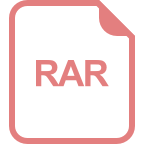
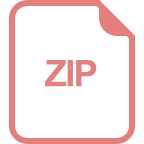
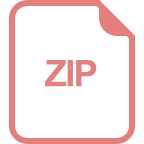
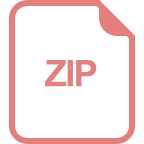
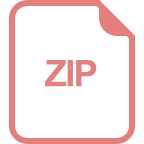
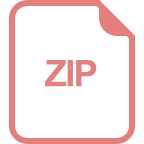