keras实现双向LSTM
时间: 2023-11-16 11:00:22 浏览: 161
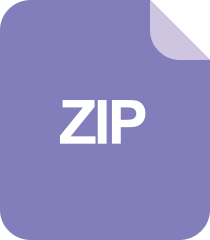
基于keras实现的LSTM网络

双向LSTM是一种能够同时考虑过去和未来信息的循环神经网络,它可以通过前向和后向两个方向来处理序列数据。在Keras中,可以通过使用Bidirectional函数来实现双向LSTM。下面是一个简单的例子:
```python
from keras.models import Sequential
from keras.layers import LSTM, Bidirectional
model = Sequential()
model.add(Bidirectional(LSTM(64, return_sequences=True), input_shape=(timesteps, input_dim)))
model.add(Bidirectional(LSTM(32)))
model.add(Dense(num_classes, activation='softmax'))
model.compile(loss='categorical_crossentropy', optimizer='adam', metrics=['accuracy'])
```
在这个例子中,我们首先定义了一个Sequential模型,然后添加了两个Bidirectional LSTM层,其中第一个LSTM层返回完整的输出序列,而第二个LSTM层只返回最后一个时间步的输出。最后,我们添加了一个全连接层来输出预测结果,并编译了模型。
阅读全文
相关推荐
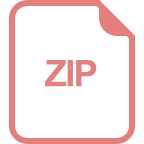


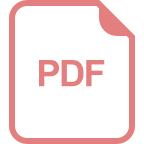
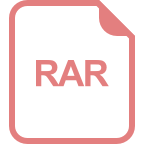
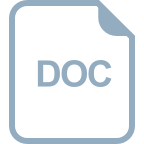

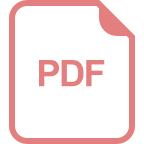
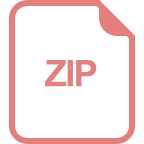
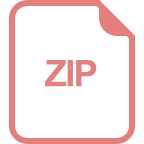
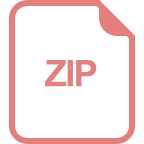
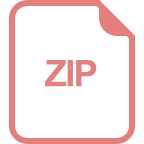
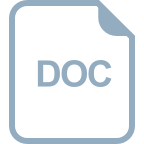



