>>> type(fee) <class 'numpy.float64'>
时间: 2024-11-09 22:21:29 浏览: 19
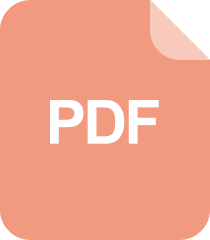
numpy.where() 用法详解

`type(fee)` 是用来检查变量 `fee` 的数据类型的。在 TensorFlow 中,如果你有一个数值变量(如浮点数),它的类型通常是与 NumPy 类似的。例如:
```python
# 假设 fee 是一个 TensorFlow 浮点数
fee = tf.ones([1], dtype=tf.float32) # 创建一个一维全为 1 的浮点数向量
# 使用 TensorFlow 的 type 函数可以确认其类型
tf_type_fee = type(fee)
print(tf_type_fee) # 输出可能是 <class 'tensorflow.python.framework.ops.Tensor'>
# 或者使用 numpy 兼容的方法来查看内部类型
np_type_fee = fee.numpy().dtype.type
print(np_type_fee) # 输出:<class 'numpy.float32'>
```
从这里可以看出,`fee` 变量在 TensorFlow 中实际上是作为一个 `Tensor` 对象存储的,但你可以通过 `.numpy()` 方法将其转换为 NumPy 数组,从而访问到其底层的 `numpy.float32` 类型。
阅读全文
相关推荐
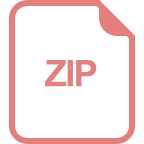















