用python语言编写一段ICP点云匹配算法
时间: 2023-06-04 11:07:30 浏览: 604
这是一个合理的问题,我可以尝试回答。ICP(Iterative Closest Point)算法是一种用于点云匹配的算法,其目标是找到两个点云之间的最佳刚性变换。以下是一个基本的Python实现:
```python
import numpy as np
def icp(pointcloud1, pointcloud2, max_iterations=100, tolerance=1e-6):
"""
ICP pointcloud matching algorithm
:param pointcloud1: source pointcloud as a Nx3 numpy array
:param pointcloud2: target pointcloud as a Nx3 numpy array
:param max_iterations: maximum number of iterations (default=100)
:param tolerance: convergence threshold (default=1e-6)
:return: transformation matrix (4x4) and error (mean squared distance)
"""
num_points = pointcloud1.shape[0]
src_homogeneous = np.hstack((pointcloud1, np.ones((num_points, 1))))
target_homogeneous = np.hstack((pointcloud2, np.ones((num_points, 1))))
transformation = np.identity(4)
error = None
prev_error = None
it = 0
while it < max_iterations:
distances = np.linalg.norm(src_homogeneous @ transformation.T - target_homogeneous, axis=1)
error = np.mean(distances ** 2)
if prev_error is not None and np.abs(error - prev_error) < tolerance:
break
closest_indices = np.argmin(distances, axis=0)
closest_points = pointcloud1[closest_indices]
centroid_src = np.mean(pointcloud1, axis=0)
centroid_target = np.mean(pointcloud2, axis=0)
centered_src = closest_points - centroid_src
centered_target = pointcloud2[closest_indices] - centroid_target
covariance_matrix = centered_src.T @ centered_target
u, s, vh = np.linalg.svd(covariance_matrix)
rotation_matrix = vh.T @ u.T
translation_vector = centroid_target - rotation_matrix @ centroid_src
transformation[0:3, 0:3] = rotation_matrix
transformation[0:3, 3] = translation_vector
prev_error = error
it += 1
return transformation, error
```
这是一个基本的ICP实现,其中点云被表示为Nx3的矩阵,每一行表示一个点的位置。ICP可以用于许多应用,包括机器人导航、3D重建和人脸识别等。感谢您的提问!
阅读全文
相关推荐
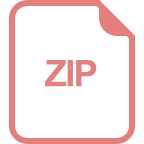




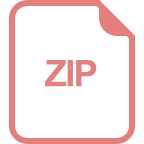
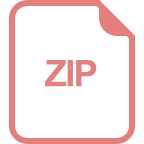
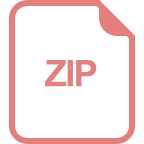
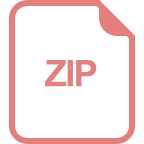
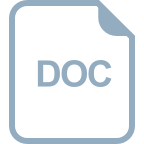






