Special tokens have been added in the vocabulary, make sure the associated word embeddings are fine-tuned or trained.
时间: 2024-08-13 07:05:40 浏览: 81
In natural language processing (NLP) models, special tokens serve as placeholders or indicators for specific types of inputs or tasks that require unique handling. These tokens are usually added to the vocabulary during model initialization or can be dynamically incorporated during training. They help the model understand and process:
1. **Padding** and **masking**: `[PAD]` and `[MASK]` tokens are used to handle variable-length sequences and indicate missing or padding elements.
2. **Segmentation**: `[SEP]` or `[CLS]` tokens separate sentences or segments in multi-token inputs, like in BERT or RoBERTa.
3. **Classification and tagging**: `[CLS]` is often used as the first token in a sequence for classification tasks, while `[MASK]` might be used for masked language modeling.
4. **Positional information**: `[unused1]`, `[unused2]`, etc., denote positions in the sequence.
When these special tokens are present, their word embeddings must be fine-tuned or trained along with the rest of the model parameters. Fine-tuning ensures that the model adapts to the specific task at hand, while training from scratch would initialize them anew without prior knowledge. This adaptation helps the model capture the context-specific meaning of these tokens and improve overall performance.
相关推荐
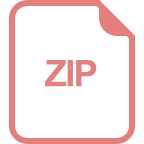
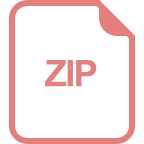
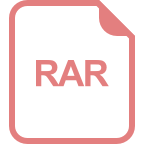














