基于tensorflow的vit模型的图像分类代码
时间: 2023-09-27 16:08:59 浏览: 50
以下是基于TensorFlow的ViT模型的图像分类代码示例:
```python
import tensorflow as tf
import tensorflow_datasets as tfds
from tensorflow.keras.layers.experimental.preprocessing import Resizing
from vit_keras import vit, utils
# Load the CIFAR-10 dataset
(ds_train, ds_test), ds_info = tfds.load('cifar10', split=['train', 'test'], with_info=True)
# Define some constants
NUM_CLASSES = ds_info.features['label'].num_classes
IMAGE_SIZE = 72 # ViT requires images to be divisible by 8
# Preprocess the data
def preprocess_data(data):
x = data['image']
y = tf.one_hot(data['label'], NUM_CLASSES)
x = Resizing(IMAGE_SIZE, IMAGE_SIZE)(x) / 255.0 # Resize and normalize
return x, y
ds_train = ds_train.map(preprocess_data).batch(32).prefetch(tf.data.experimental.AUTOTUNE)
ds_test = ds_test.map(preprocess_data).batch(32).prefetch(tf.data.experimental.AUTOTUNE)
# Define the ViT model
model = vit.vit_b16(
image_size=IMAGE_SIZE,
activation='softmax',
classes=NUM_CLASSES,
include_top=True,
pretrained=True
)
# Compile the model
model.compile(
optimizer=tf.keras.optimizers.Adam(),
loss=tf.keras.losses.CategoricalCrossentropy(),
metrics=[tf.keras.metrics.CategoricalAccuracy()]
)
# Train the model
model.fit(
ds_train,
validation_data=ds_test,
epochs=10
)
# Evaluate the model
model.evaluate(ds_test)
```
这个代码示例使用了 TensorFlow Datasets 中的 CIFAR-10 数据集,并使用了 ViT-B16 模型进行图像分类。首先,我们定义了一些常量,包括类别数量和图像大小。然后,我们定义了一个函数来对数据进行预处理,包括缩放和归一化。接下来,我们使用 `vit.vit_b16` 函数定义了 ViT 模型,并将其编译。最后,我们使用 `model.fit` 函数训练模型,并使用 `model.evaluate` 函数评估模型。
相关推荐
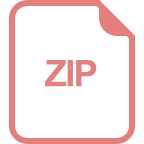
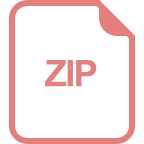
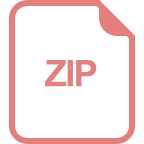














